4 Best Inpainting Models in Stable Diffusion
Dive into our exploration of best Stable Diffusion Inpainting models on Segmind, each offering unique capabilities for targeted image modifications.
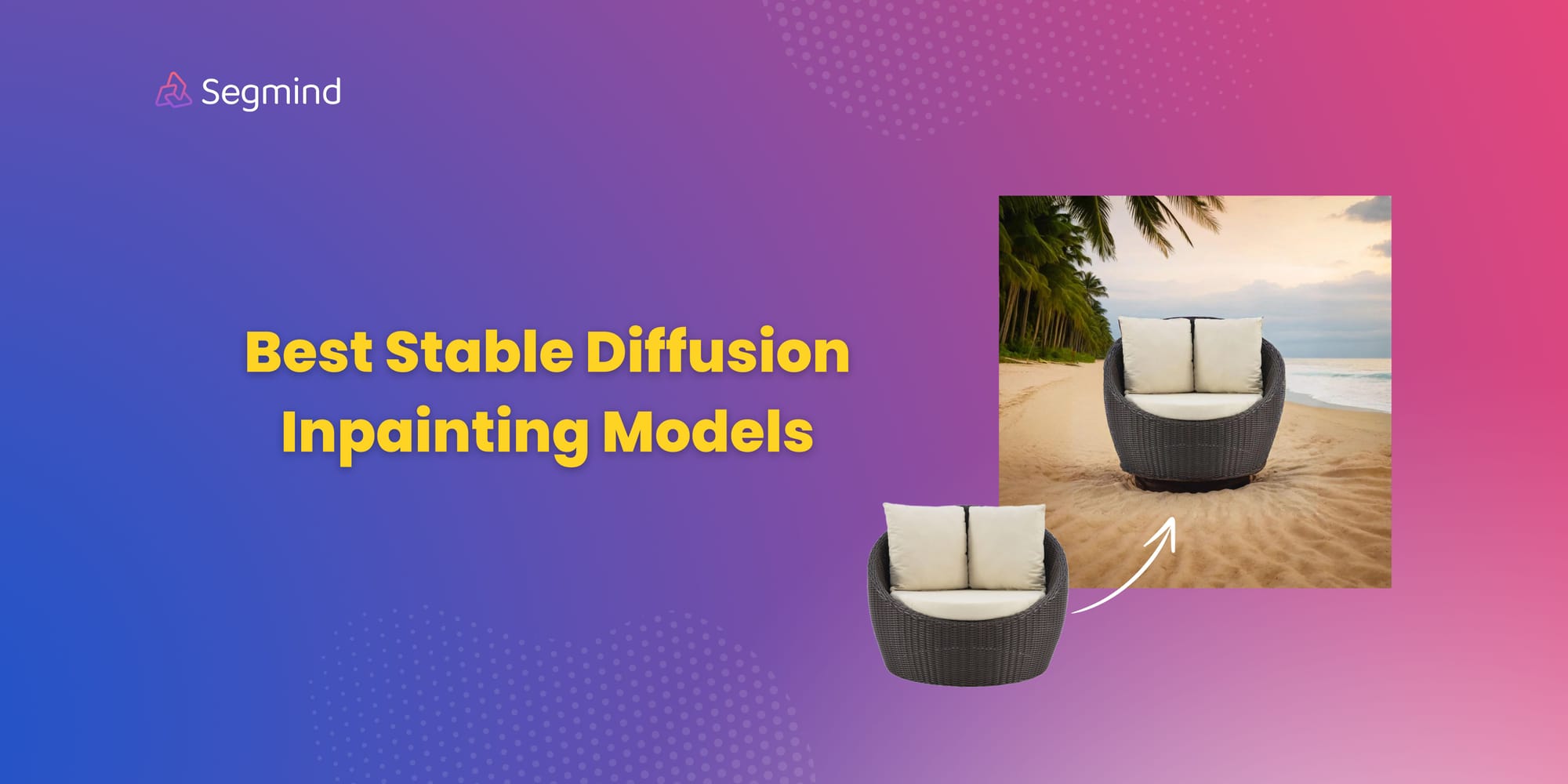
Inpainting is a process which enables the alteration of specific parts of an image, typically achieved by masking the section that needs to be changed. The areas designated for alteration are marked with white pixels, while the sections to be preserved are indicated with black pixels, allowing for precise and targeted modifications. In this blog post, we will explore various Stable Diffusion Inpainting models available on Segmind.
* Get $0.50 daily free credits on Segmind.
SDXL Inpainting
SDXL inpainting works with an input image, a mask image, and a text prompt. The input is the image to be altered. The mask image, marked with white pixels for areas to change and black pixels for areas to preserve, guides the alteration. The prompt provides textual instructions for the model to follow when altering the masked areas. The result is a seamlessly blended image with the masked areas altered as per the prompt.
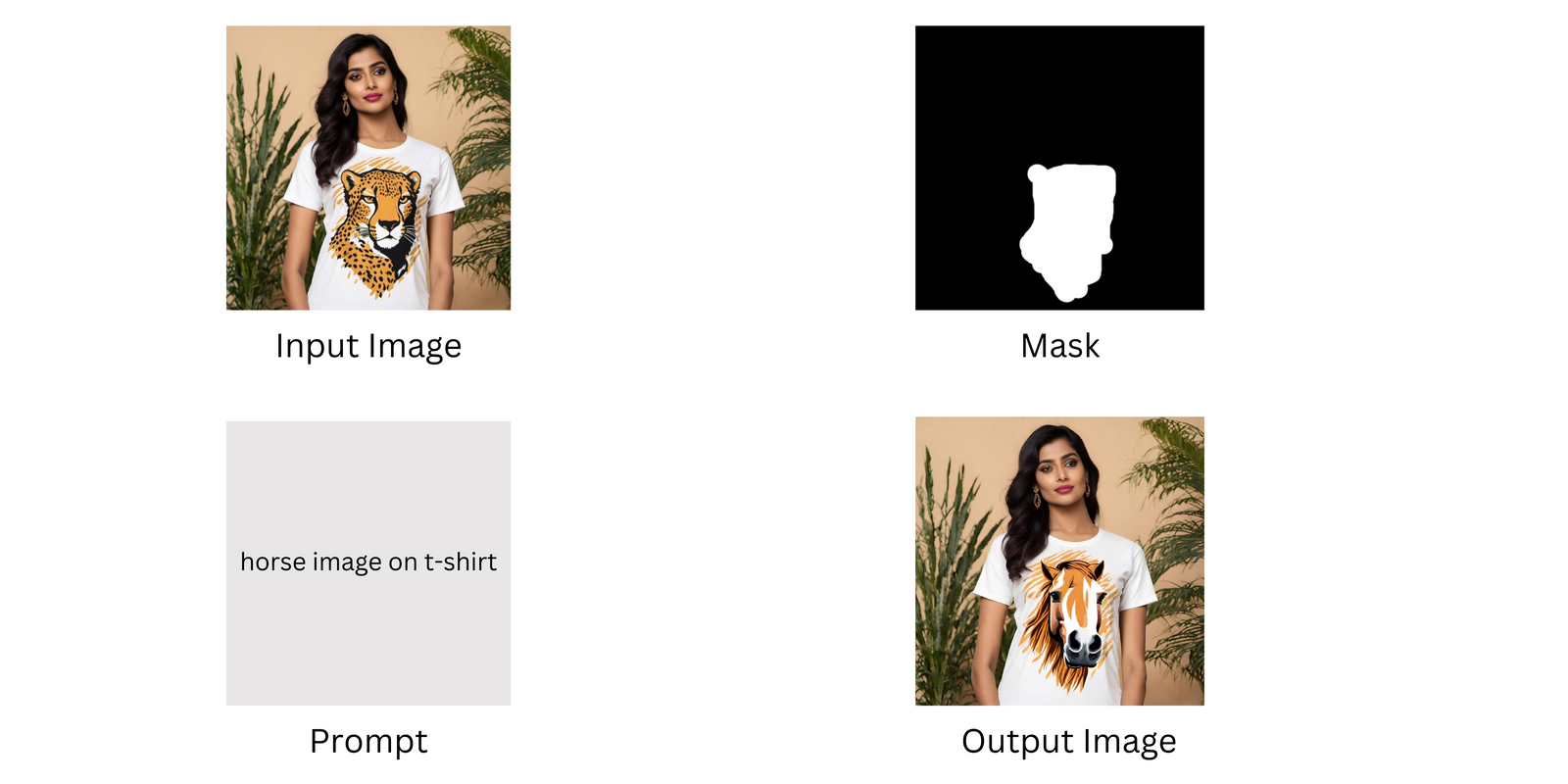
SDXL ControlNet Inpainting
Now, when we pair SDXL Inpainting with ControlNet, things get even more interesting. ControlNet like a Canny edge detector is a popular preprocessing technique used for edge detection in image processing. What does this mean? Well, ControlNet helps to preserve the composition of the subject in the image. It clearly separates the subject from the background and vice versa. This is crucial when you want to maintain the structure of the subject while changing its appearance or the surrounding area.
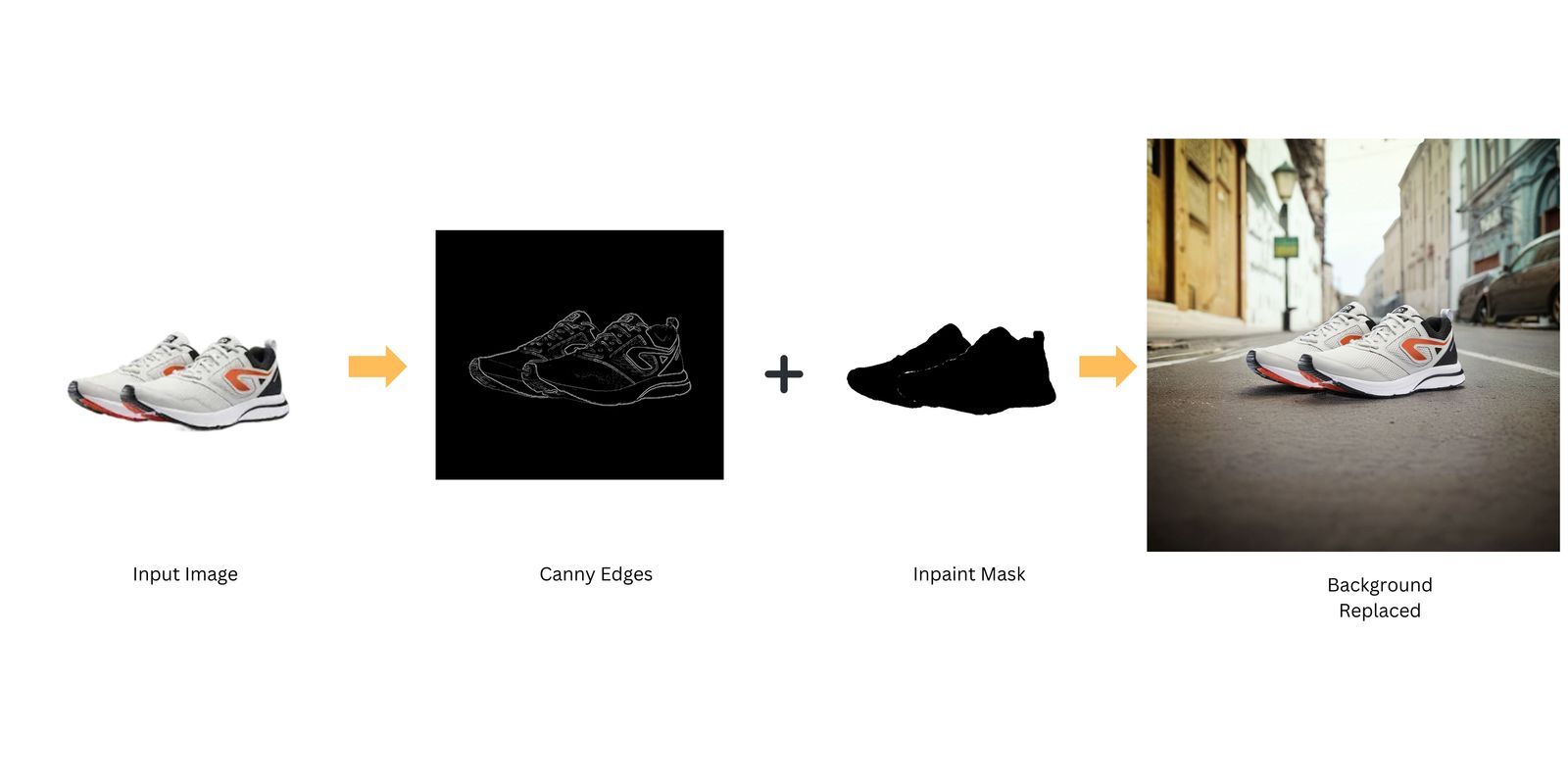
Background Replace with Inpainting
ControlNet + SDXL Inpainting + IP Adapter
Background Replace is SDXL inpainting when paired with both ControlNet and IP Adapter conditioning. This model offers more flexibility by allowing the use of an image prompt along with a text prompt to guide the image generation process. This means you can use an existing image as a reference and a text prompt to specify the desired background.
- ControlNet helps in preserving the composition of the subject in the image by clearly separating it from the background. This is crucial when you want to maintain the structure of the subject while changing its appearance or the surrounding area.
- IP Adapter allows to use an existing image as a reference, and a text prompt to specify the desired background. For example, if you have a picture of a bench and you want to change the background to a living room with the help of a reference image (or IP image) of a living room, you could use a text prompt like “living room”. The IP Adapter then guides the Stable Diffusion Inpainting model to replace the background of the image in a way that matches the reference image. So, in our example, the bench in the room would now appear to be in a living room just like in the reference image.
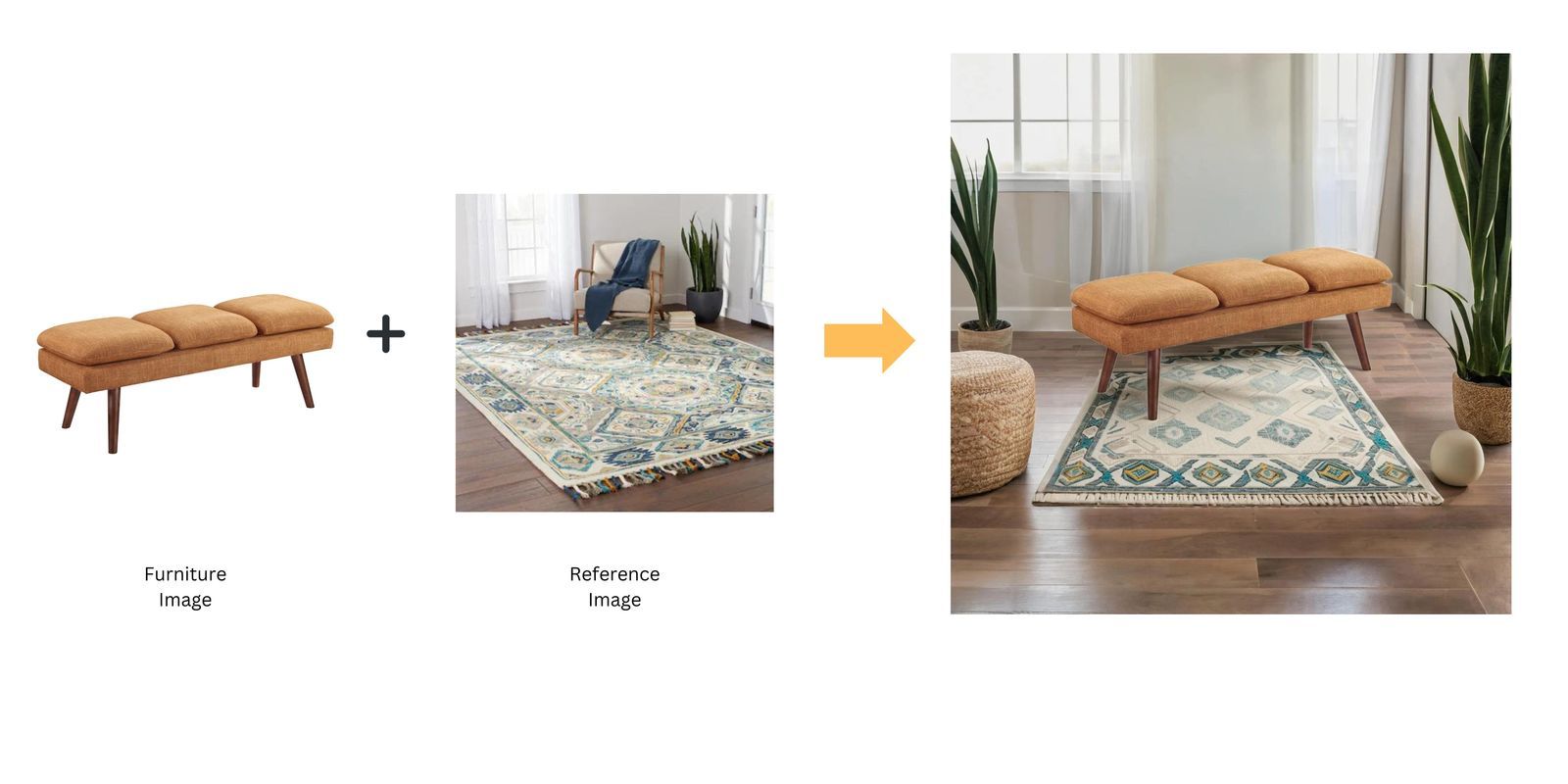
Fooocus Inpaint
Fooocus Inpainting takes the input image of an object and the mask created in the previous step. It then fills the background with a new one based on the text prompts. Fooocus has control methods like Pyracanny, CPDS, and Image Prompt to guide inpainting. Pyracanny is similar to the Canny edge preprocessor, CPDS is similar to the Depth preprocessor, and Image Prompt works along similar lines as an image encoder like IP Adapter.
The inpainting is influenced by control methods like Pyracanny, CPDS, Image Prompt. The extent of this influence is determined by two settings:
- Stop At: This setting determines the duration of the control method’s influence. It stops the control at certain sampling steps. For instance, a value of 0.5 implies stopping 50% of the sampling steps and 1 (which is the maximum value) implies stopping 100% of the sampling step.
- Weight: This setting determines the degree of influence the control method has on the original image. A higher weight means a greater influence.
Try these variations of inpainting models on Segmind based on your needs. Each of these models offers unique capabilities.