Fine-tune Stable Diffusion with LoRA Training
A comprehensive guide on fine-tuning Stable Diffusion with LoRA training to generate custom images.
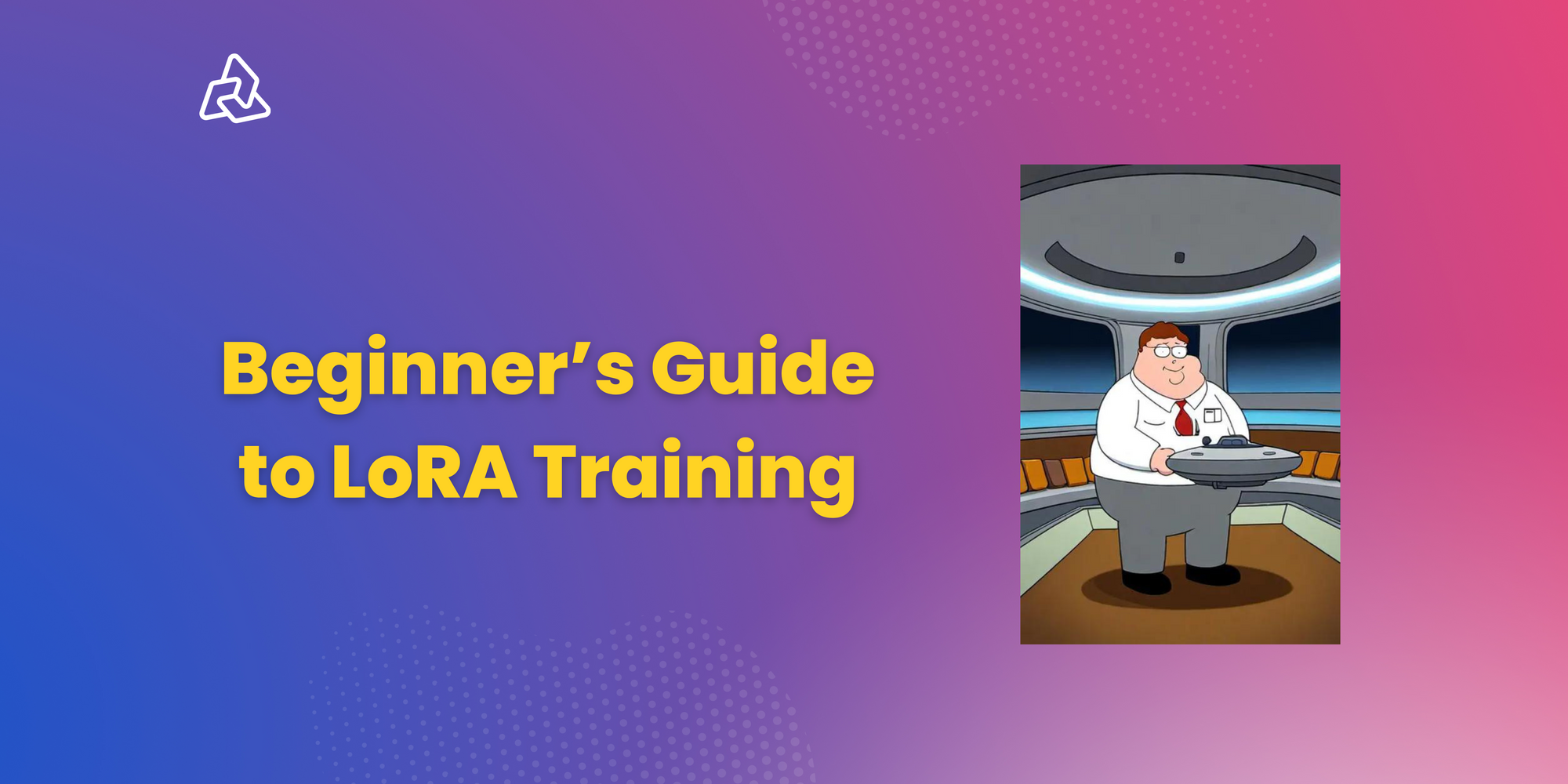
Stable Diffusion models are trained on vast datasets, enabling them to generate a wide array of images with impressive versatility. However, despite their broad capabilities, Stable Diffusion models can sometimes struggle when it comes to generating images of specific themes or styles.
Imagine you're trying to create images in a specific style. For instance, you might want to generate images that resemble the bright, exaggerated features of comic book art. While a base Stable Diffusion model is quite capable, it might not consistently nail these distinct visual styles.
This is where fine-tuning comes into play. Fine-tuning is essentially a process of teaching the Stable Diffusion model to become more adept at handling specific types of image generation tasks. It involves adjusting and retraining the model on a more focused dataset that represents the specific style, theme, or subject matter you are interested in. By doing so, the model becomes more specialized and can produce results that are more aligned with your specific needs.
What is LoRA Fine-tuning?
But how do we fine-tune these models effectively and efficiently? This is where LoRA, or Low-Rank Adaptation, comes into the picture. LoRA is a technique that allows us to fine-tune large models like Stable Diffusion without the need to retrain them entirely, saving both time and computational resources.
LoRA stands for Low-Rank Adaptation, a method designed to fine-tune large-scale models in a more efficient manner. The key idea behind LoRA is to update only a small part of the model's weights, specifically targeting those that have the most significant impact on the task at hand. This approach contrasts with traditional fine-tuning methods, where a large portion of the model's weights might be updated, requiring substantial computational power and time.
Types of LoRA Fine-tuning?
Each of the below LoRA models, by being fine-tuned on a specific set of images, becomes highly specialized in generating images within its trained theme. This specialization allows for more accurate and contextually appropriate image generation in line with the specific needs of the users they serve.LoRA models can be classified into various types based on the underlying concept guiding their training. Some examples are given below.
Style LoRA
- Crayon Syle LoRA SDXL: Here, the model is trained on images that look like they were drawn with crayons - with all the characteristic texture and vibrant, uneven color application. This would be ideal for generating images that have a childlike, hand-drawn quality, useful for children's books, educational materials, or nostalgic art pieces.
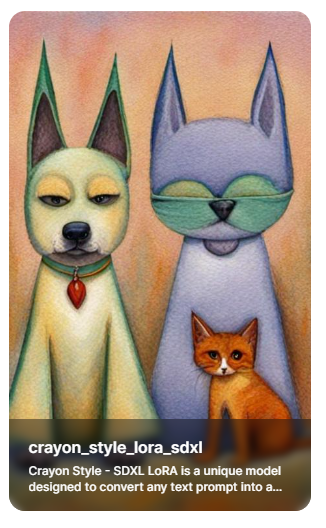
Character LoRA
- SDXL Ugly Sonic LoRA: This model would be specifically fine-tuned on images of 'Ugly Sonic,' a version of the Sonic the Hedgehog character. By training on this niche, the model could generate new images or variations of this particular character rendition, which could be of interest for meme creators, fan art communities, or discussions about character design in media.
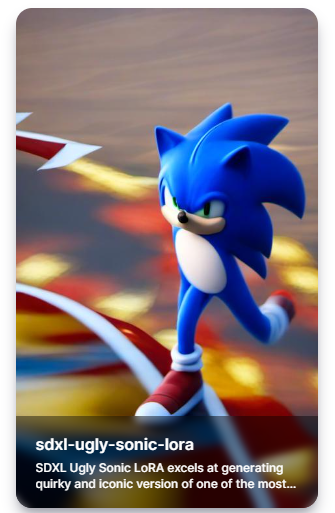
Concept LoRA
- Sticker Sheet LoRA: This model trained on the concept of 'Sticker Sheet' would specialize in generating images that resemble stickers, complete with outlines, bright colors, and perhaps even simulated textures or effects like glossiness. This could be incredibly useful for graphic designers, educators, or marketers who need custom sticker designs for various projects.
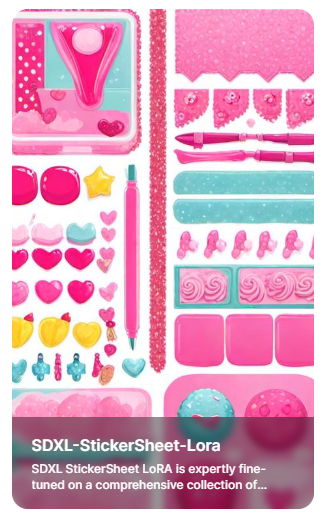
Object LoRA
- Dog Example SDXL LoRA: Training a model specifically on images of dogs would make it adept at creating a wide variety of dog images, possibly in different poses, breeds, or artistic styles. This could be particularly useful for pet-related industries, artists who specialize in pet portraits, or for creating educational content about different dog breeds.
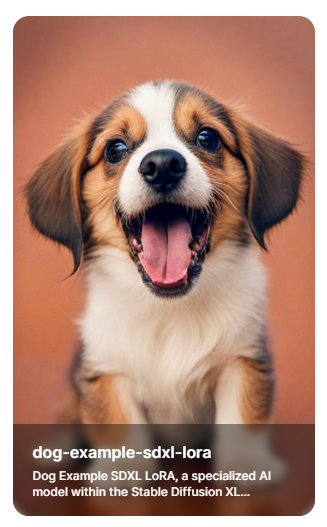
How to train your own LoRA model?
Here are the basic steps you can make your own LoRA model:
a. Preparing Data Set
- Start by defining a clear theme or subject for your model. With this focus, gather a set of 10 to 15 high-resolution images. These images should not only be clear and relevant to your theme but also varied. Expanding the dataset (50 to 100 Images) can significantly enhance the performance of a LoRA model, especially when the objective is to replicate a specific style or theme. The total file size of images should be less than 5GB.
- To avoid overfitting, where the model becomes too narrowly attuned to your specific dataset, limit your selection to this range. Ensure that the images are not too similar to each other; diversity in your dataset prevents the model from becoming overly specialized.
b. Training LoRA
You can seamlessly web train your LoRA model on Segmind. All your model training tasks can be efficiently managed and initiated through the model training module in your console. To kickstart the training process, you'll need to provide specific details for your custom model. This includes uploading the image zip file that contains your chosen dataset for training. Additionally, Segmind offers advanced settings, which give you the flexibility to fine-tune various parameters of the training process, allowing for more precise and controlled model development.
Conclusion
In closing, in this guide we tried to simplify the process of fine-tuning LoRA models, providing you with the foundational knowledge and practical strategies to embark on this exciting journey. Each step you take in fine-tuning and applying LoRA models opens up new possibilities for innovation and creativity.