Fine-tune Stable Diffusion models with Segmind
Fine-tune Stable Diffusion models (SSD-1B & SDXL 1.0) using your own dataset with the Segmind training module.
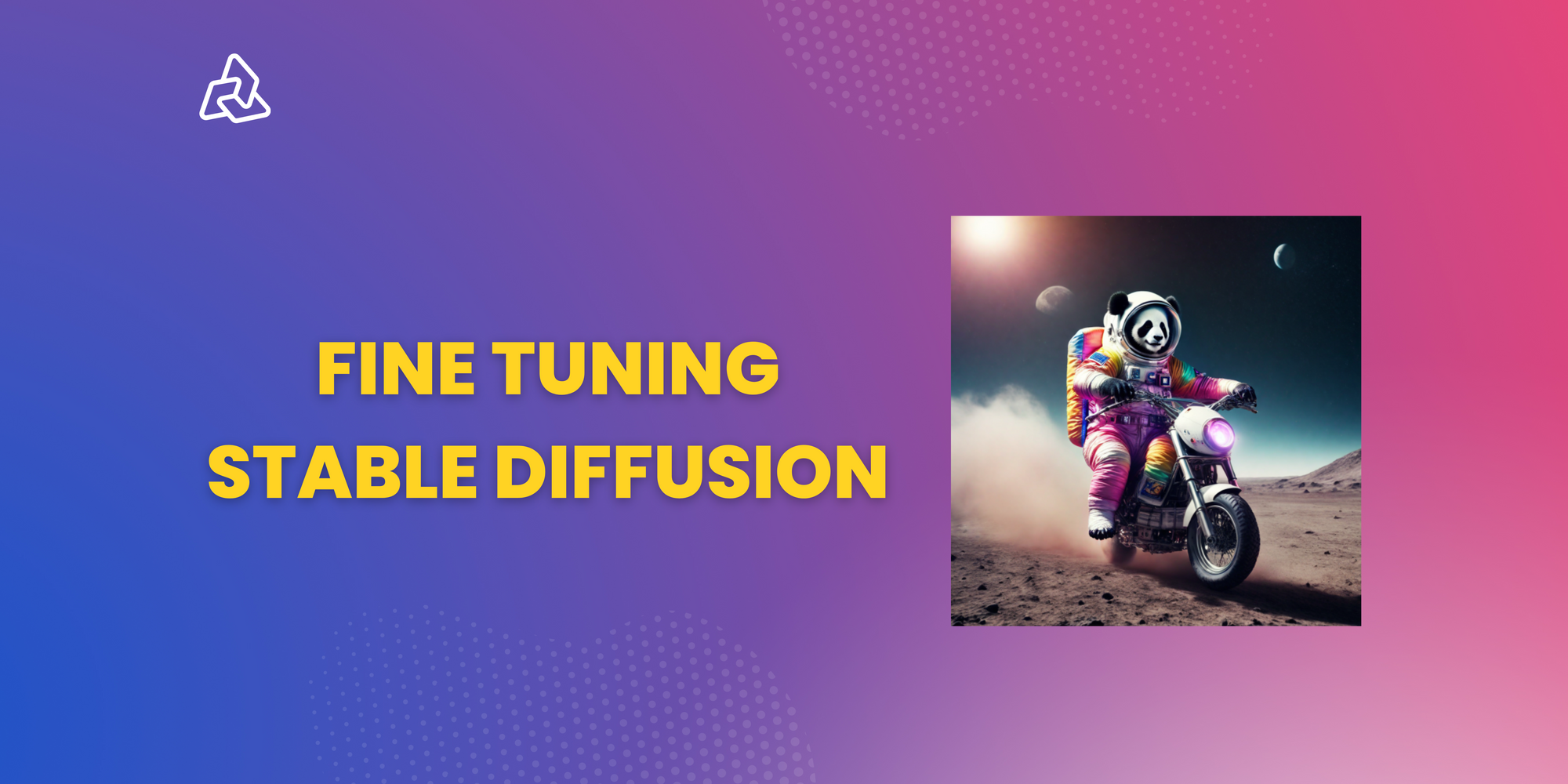
In the realm of generative art and AI-driven content creation, Stable Diffusion models have emerged as a versatile tool, capable of producing a wide array of images across various styles and subjects. However, their generalist nature means they may lack finesse in generating highly specific or nuanced content. This is where fine-tuning comes into play, allowing users to specialize these models in particular domains, such as anime, human figures etc.
Fine-tuning is the process of continuing the training of a pre-existing Stable Diffusion model or checkpoint on a new dataset that focuses on the specific subject or style you want the model to master. The result is a fine-tuned model that maintains the broad capabilities of the original while excelling in your chosen area.
Fine tuning methods
Here are some of the fine tuning methods:
- Checkpoint Training: Enhances the base model's capabilities by integrating a new dataset for a specific subject or style, ideal for creating a targeted thematic focus and combining broad model knowledge with specialized domains.
- LoRA (Low-Rank Adaptation): Offers a streamlined fine-tuning process by making minor yet significant adjustments to the model, resulting in smaller file sizes and a good balance between efficiency and customization capabilities.
- Dreambooth: Allows for deep personalization by fine-tuning the model with a small set of images, enabling the generation of highly specific content that captures the subtleties of the chosen subject.
Checkpoint training broadens the scope of a base model by integrating a new dataset tailored to a specific subject or style. This approach deepens the model's expertise in the selected area such as anime, realism etc, making it adept at generating content with a particular thematic focus. Especially useful for inducing a specific bias in the model, such as training Stable Diffusion with vintage car imagery to steer its generative abilities towards that niche. Checkpoint training is ideal for those wishing to combine the model's extensive knowledge with a specialized domain.
Dreambooth training significantly enhances a model's ability to generate images of a particular subject using a small set of 4-5 images and maps it to a special token, allowing for the model to generate that subject in various different styles and settings. For example, by using Dreambooth, you can integrate personal photographs into the model, enabling it to generate images featuring these subjects. Models trained with Dreambooth utilize a unique keyword to guide the generation process.
LoRA models are essentially compact versions of Stable Diffusion that introduce minor, yet impactful modifications to the standard models. Their size is significantly smaller — often 10 to 100 times less than full checkpoint models — making them a practical choice for those with a vast array of models. LoRA training strikes a fine balance between efficiency and capability, offering manageable file sizes (ranging from 2 to 200 MBs) without compromising much on the training potential. LoRA training requires more images (20 to 30 images) and acts as a more efficient finetuning method since it only trains a fraction of the weights and can be used to learn various styles such as anime, realism, cartoon etc.
How to fine tune Stable Diffusion Models
At present, Segmind offers support for both LoRA and Dreambooth training methods. Checkpoint training will soon be added. We will promptly revise this article to reflect the availability of checkpoint training once it's launched.
Here's how you can use your own dataset to fine-tune stable diffusion models.
- You can manage and initiate all your model training tasks on the Model Training module of your console.
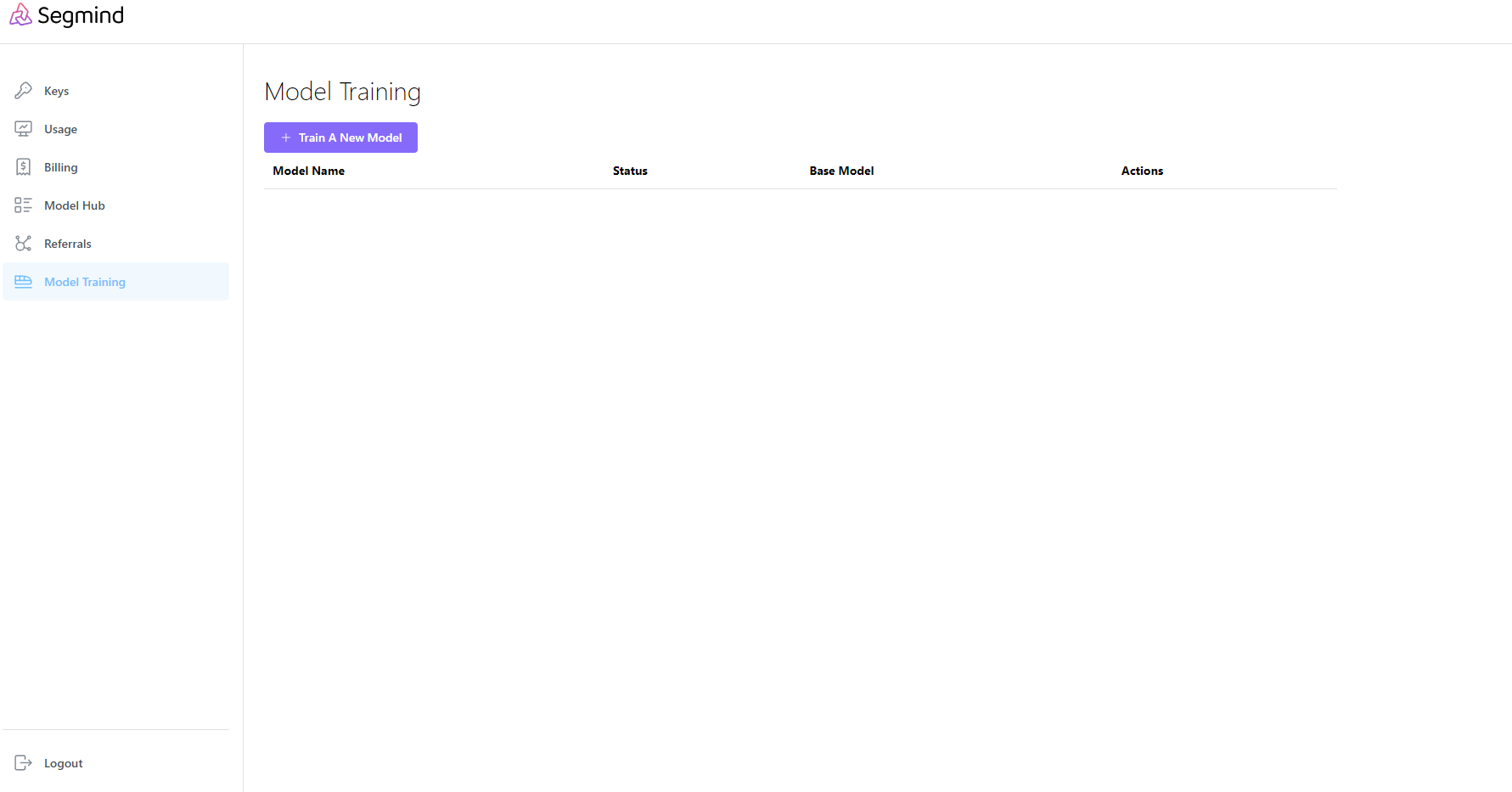
2. To begin the training process specify details for training your custom model.
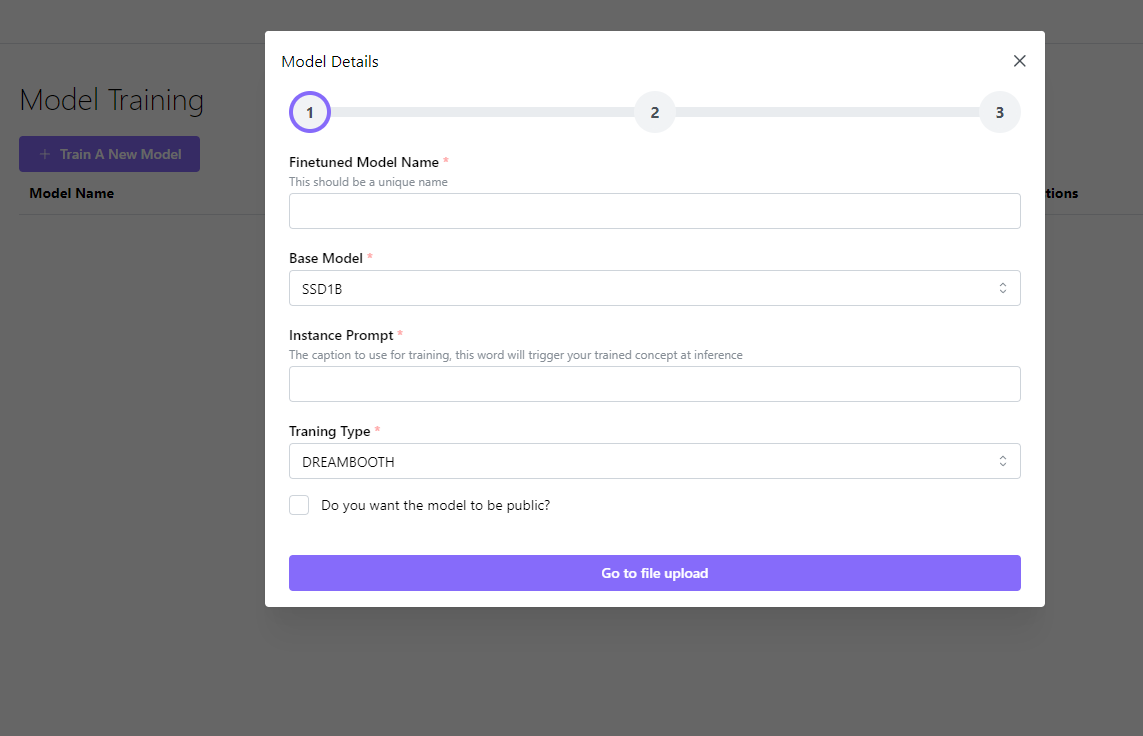
3. Upload the image file containing the dataset you wish to use for training.
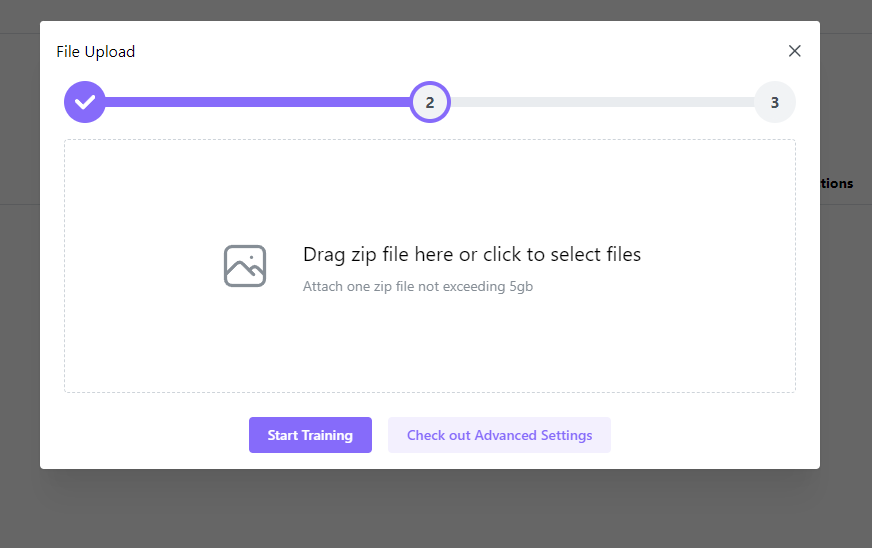
4. Advanced Settings allow you to adjust various parameters of the training process for more precise control.
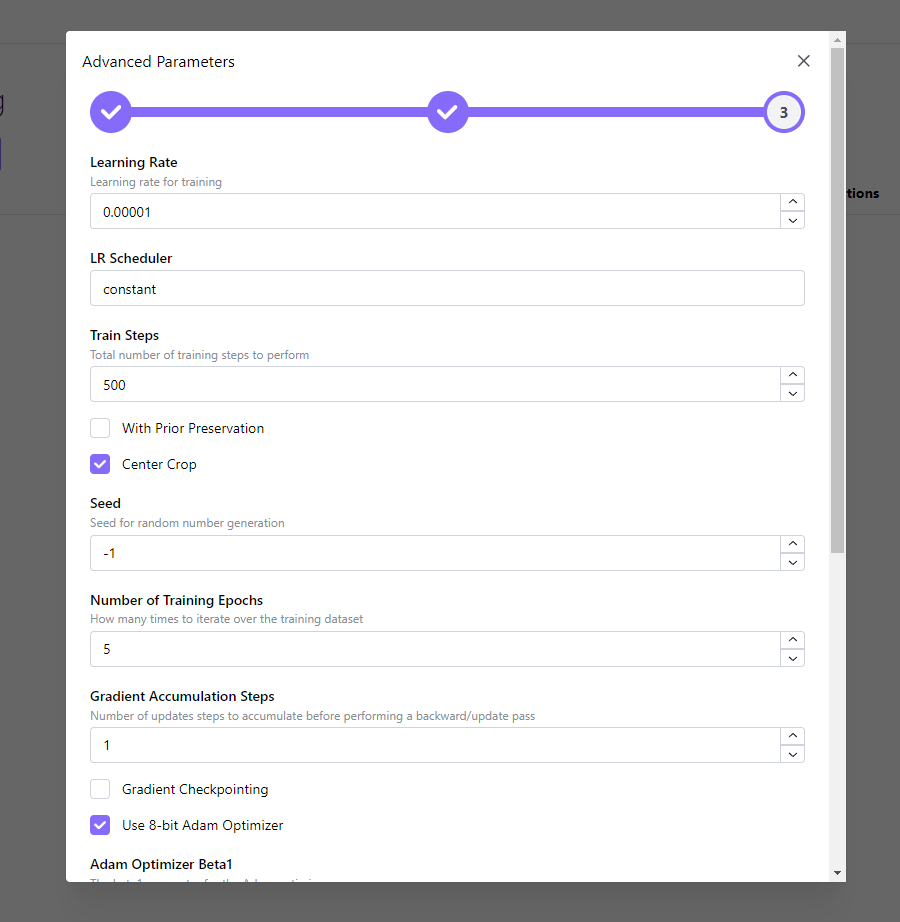
Striking the Perfect Balance
When embarking on the fine-tuning of Stable Diffusion models, a key consideration is finding the right balance between the model's file size and the depth of training customization you require. Each method available comes with its own set of trade-offs that cater to different needs and constraints.
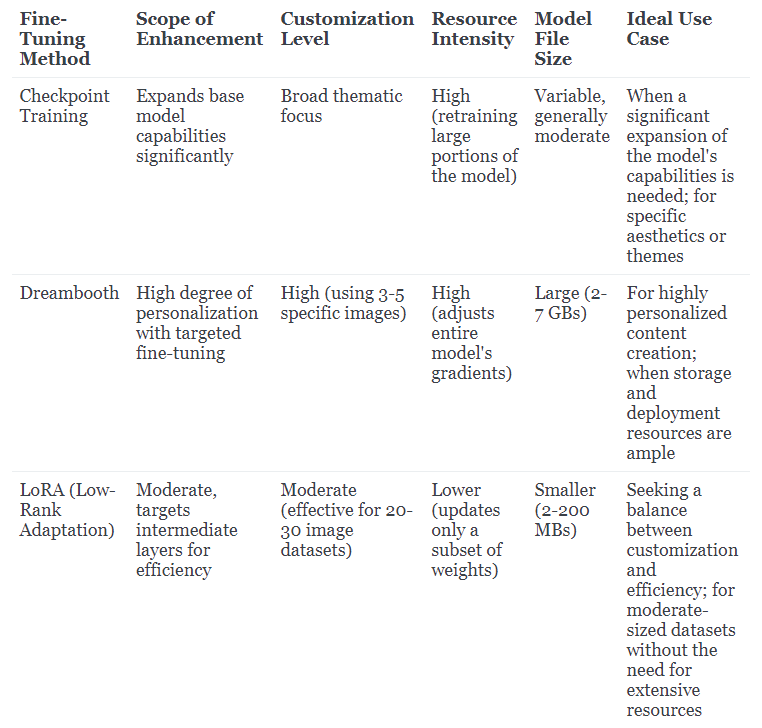
By leveraging these techniques through Segmind, you can transform a generalist Stable Diffusion model into a specialized tool tailored to your creative needs. Whether you're looking to generate art in a particular style or create images with a specific subject, fine-tuning with Segmind can help you achieve your vision with greater precision and efficiency.