AI Image Enhancement in Stable Diffusion workflows
Explore how models like ESRGAN and Codeformer elevate image sharpness and detail preservation in stable diffusion workflows.
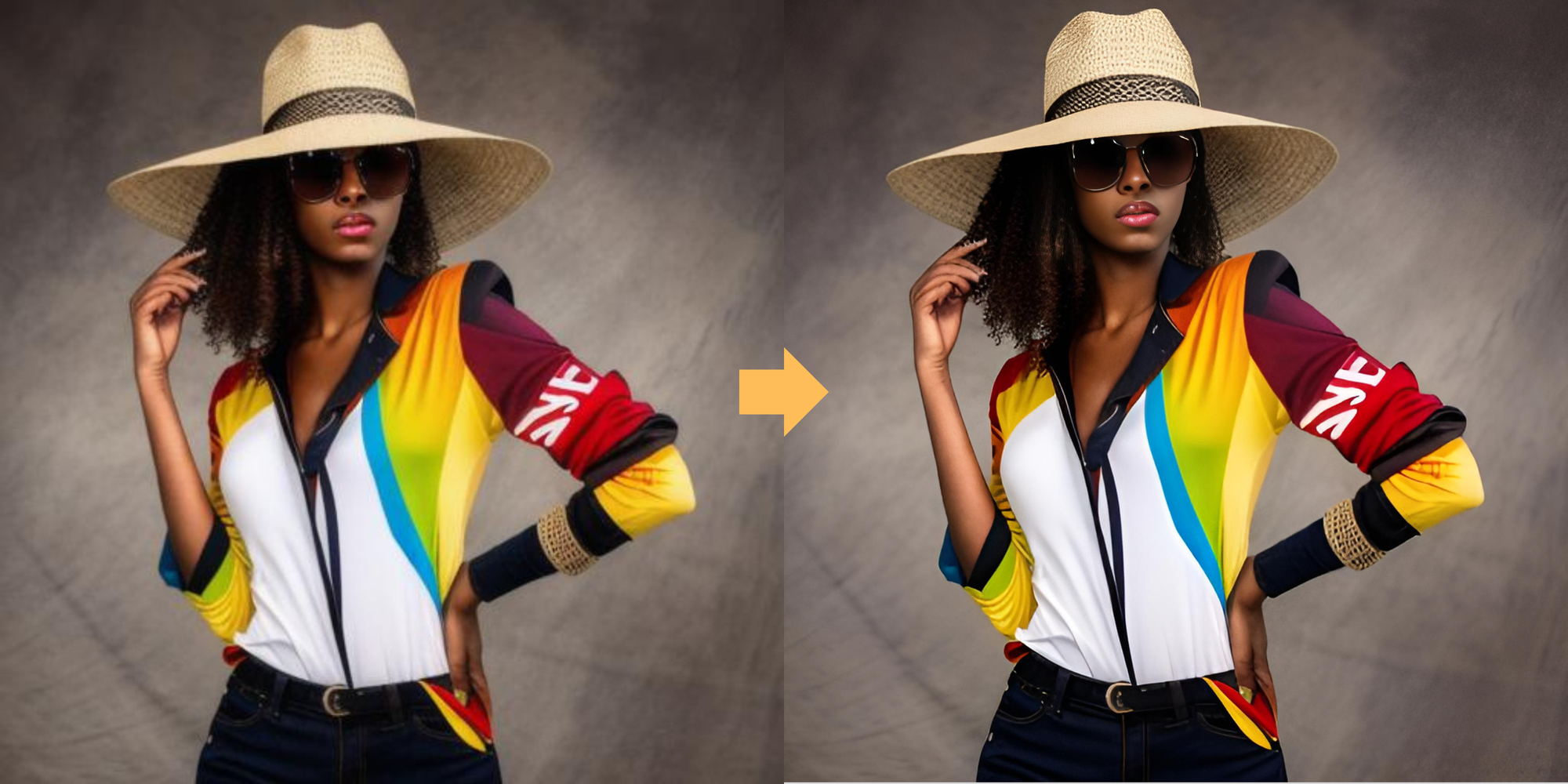
Stable diffusion models have emerged as a valuable tool for generating images. They offer versatility in producing a wide range of images that are often captivating and realistic. However, these models face a persistent challenge - the preservation of fine details and image sharpness. Despite their reputation for creating coherent and conceptually rich images, stable diffusion models struggle to maintain high-frequency information. This struggle results in a trade-off between image diversity and sharpness.
The challenges posed by stable diffusion models regarding image sharpness and detail preservation span various applications, especially in creative domains such as digital artistry and user interface design where improved visuals have the potential to redefine aesthetic possibilities, offering more immersive and engaging user experiences. These challenges are notably pertinent in sectors like E-commerce, Real Estate, App/Website Graphics, Social Sharing, and Photo Prints, where enhanced image quality can significantly impact product sales, property listings, user engagement, social media reach etc.
In this blog article, we delve into the crucial role played by AI-based image enhancement methods within stable diffusion workflows. Specifically, we explore the integration of models like ESRGAN (Enhanced Super-Resolution Generative Adversarial Network) and Codeformer to enhance the quality of images generated by stable diffusion. Both models bring a set of techniques aimed at improving image clarity and detail, grounded in advanced deep learning methodologies. In the context of our exploration, it's worth noting that the Segmind team has actively delved into stable diffusion workflows, leveraged the capabilities of ESRGAN and Codeformer to significantly enhance image quality.
In the following sections, we'll explore the intricacies of the convergence between stable diffusion and AI image enhancement, highlighting its pivotal role in real-world applications. Additionally, we'll investigate how ESRGAN and Codeformer can seamlessly integrate into stable diffusion workflows, bridging the gap between generated images and the demands of practical applications.
Increasing Image Size and Resolution
ESRGAN, or Enhanced Super-Resolution Generative Adversarial Network, is a deep learning model designed to enhance the size and resolution of images. It's especially useful when you have a low-resolution or small image that you want to make larger and more detailed without losing quality. ESRGAN achieves this by learning from high-resolution images during its training process. When you feed it a low-resolution image, it uses its learned knowledge to generate a higher-resolution version. This process, known as super-resolution, involves creating new image details that weren't present in the original low-resolution image. The result is a larger, crisper, and more detailed image that can be valuable in various applications like upscaling old photos, improving image quality in video frames, or enhancing the visual appeal of graphics and artwork.
T-shirt design workflow:
Here is our blog on our T-shirt design work flow: How to design and mock up t-shirt designs using stable diffusion
Suppose you're a designer working on a T-shirt design, and you have generated variations of the design. Unfortunately, you've encountered a common problem: the images you have are grainy and lack the sharpness and detail you need. In the context of your T-shirt design, this means you can transform those grainy images into high-resolution visuals that showcase your design with greater clarity and precision. The sharpness and fine details that ESRGAN adds to your images will not only make your T-shirt design stand out but also ensure that the printed design looks crisp and visually appealing, whether it's on clothing or any other medium where image quality is paramount. ESRGAN, in this scenario, acts as a valuable tool to elevate the visual quality of your T-shirt design and make it more impactful.
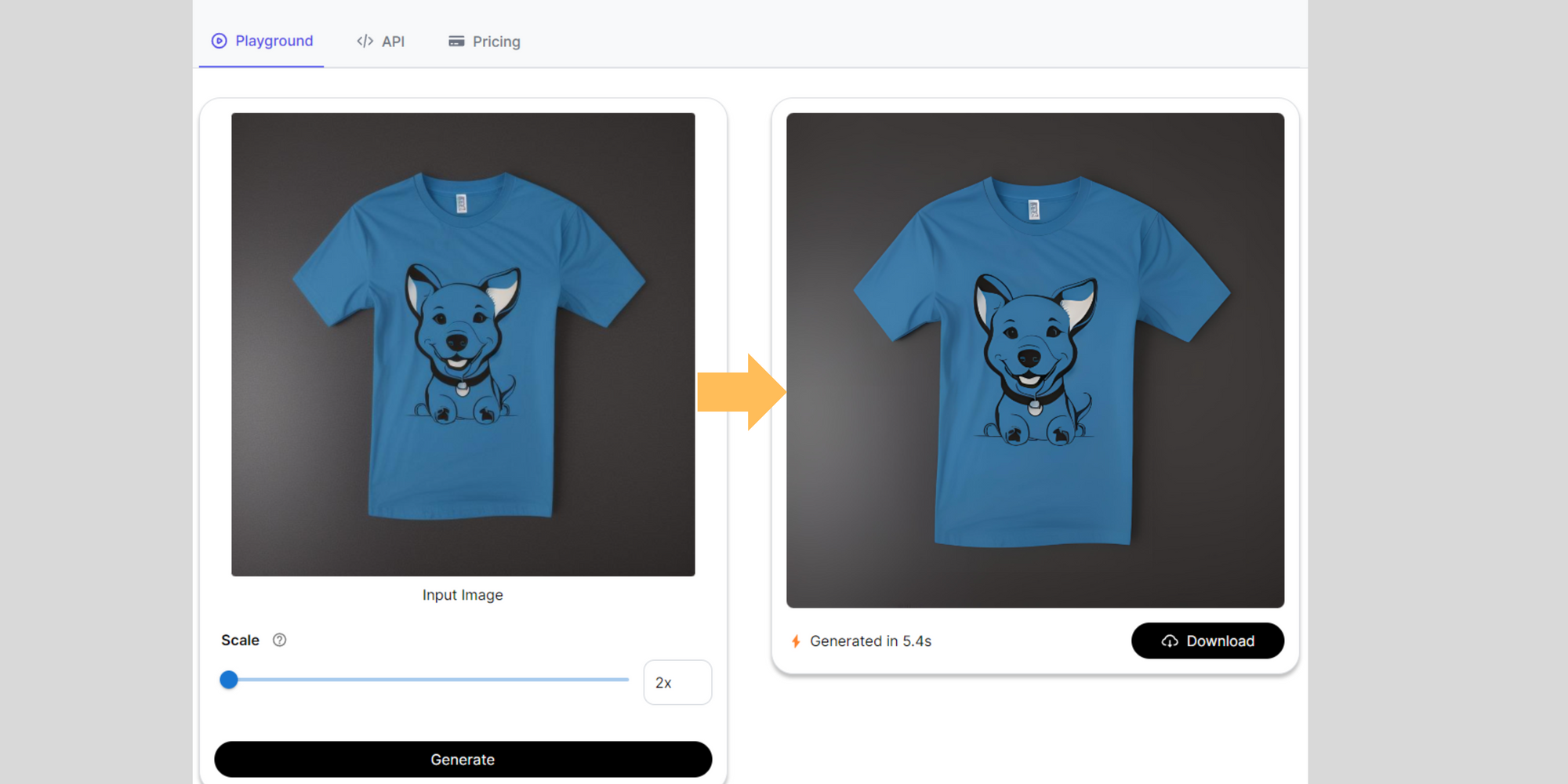
Interior and Architecture workflow:
Here is our blog on our interior and Architecture work flow: Transforming Interior Design and Architecture with Stable Diffusion
- Reference image of a room or building exterior: Begin by obtaining a reference image of the room or building exterior that serves as your starting point. This reference image will be used as a guide for the desired look and style in your project.
- ControlNet Canny to generate variations: With ControlNet Canny, you can experiment with different architectural elements, interior designs, or structural variations while maintaining the core aesthetics of your reference image. This allows you to explore creative possibilities and generate multiple iterations to choose from.
- Upscaling the images with ESRGAN: Once you have your design variations generated with ControlNet Canny, you may find that some of the resulting images are of lower resolution or appear blurry due to the transformation process. ESRGAN enhances image sharpness and increasing resolution while preserving crucial details.
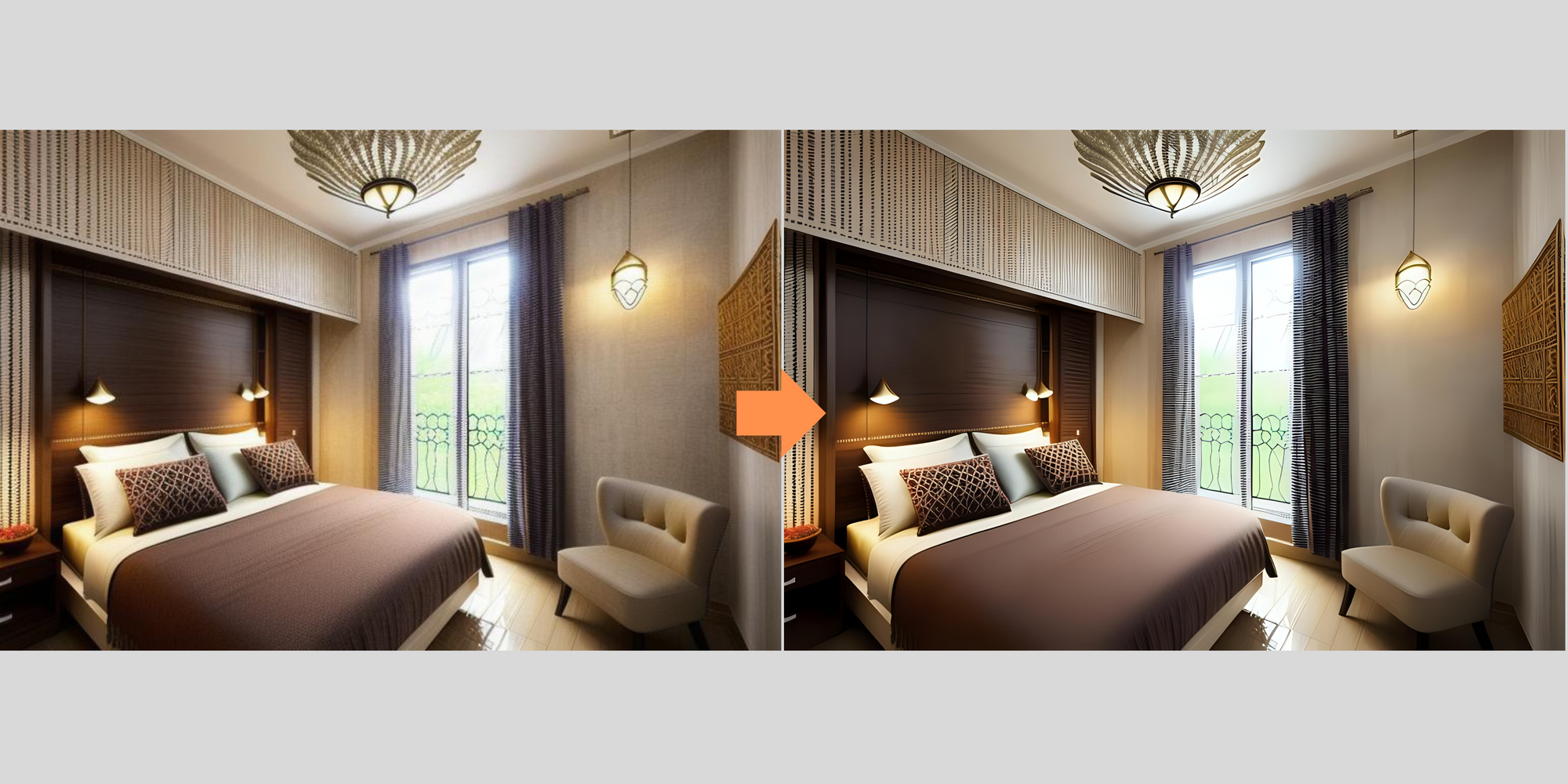
Facial Image Restoration
CodeFormer is a powerful face restoration algorithm primarily designed to enhance the quality of old or deteriorated photographs containing human faces, as well as AI-generated faces. Codeformer leverages advanced deep learning techniques to recognize and address various issues commonly found in such images. These issues may include blurriness, loss of fine details, color fading, and other forms of degradation that occur over time or during the AI generation process. By analyzing and understanding facial features and patterns, CodeFormer works to restore and improve the overall quality and appearance of faces in these images. This has practical applications in photo restoration, digital archiving, and the enhancement of AI-generated content, contributing to the preservation and enhancement of visual data in various contexts.
Sketch to Image workflow:
Here is our blog on our sketch to image work flow: The Sketch to Image using stable diffusion
- Sketch with SDXL: Sketching a rough outline or draft of the human figure using SDXL 1.0.
- Turning Sketches to real humans with ControlNet Scribble: ControlNet Scribble convert sketches into more realistic human images. Input your sketch into ControlNet Scribble, and the model will utilize its deep learning capabilities to flesh out the sketch into a more detailed and human-like representation.
- Face restoration and improving the image quality: After generating a human image from your sketch, you might find that certain aspects, such as the face, require further refinement. CodeFormer addresses any imperfections or issues in the generated image by denoising the image, improve facial details, and enhance overall image quality. This step ensures that the final human image is not only realistic but also visually pleasing and free from artifacts.
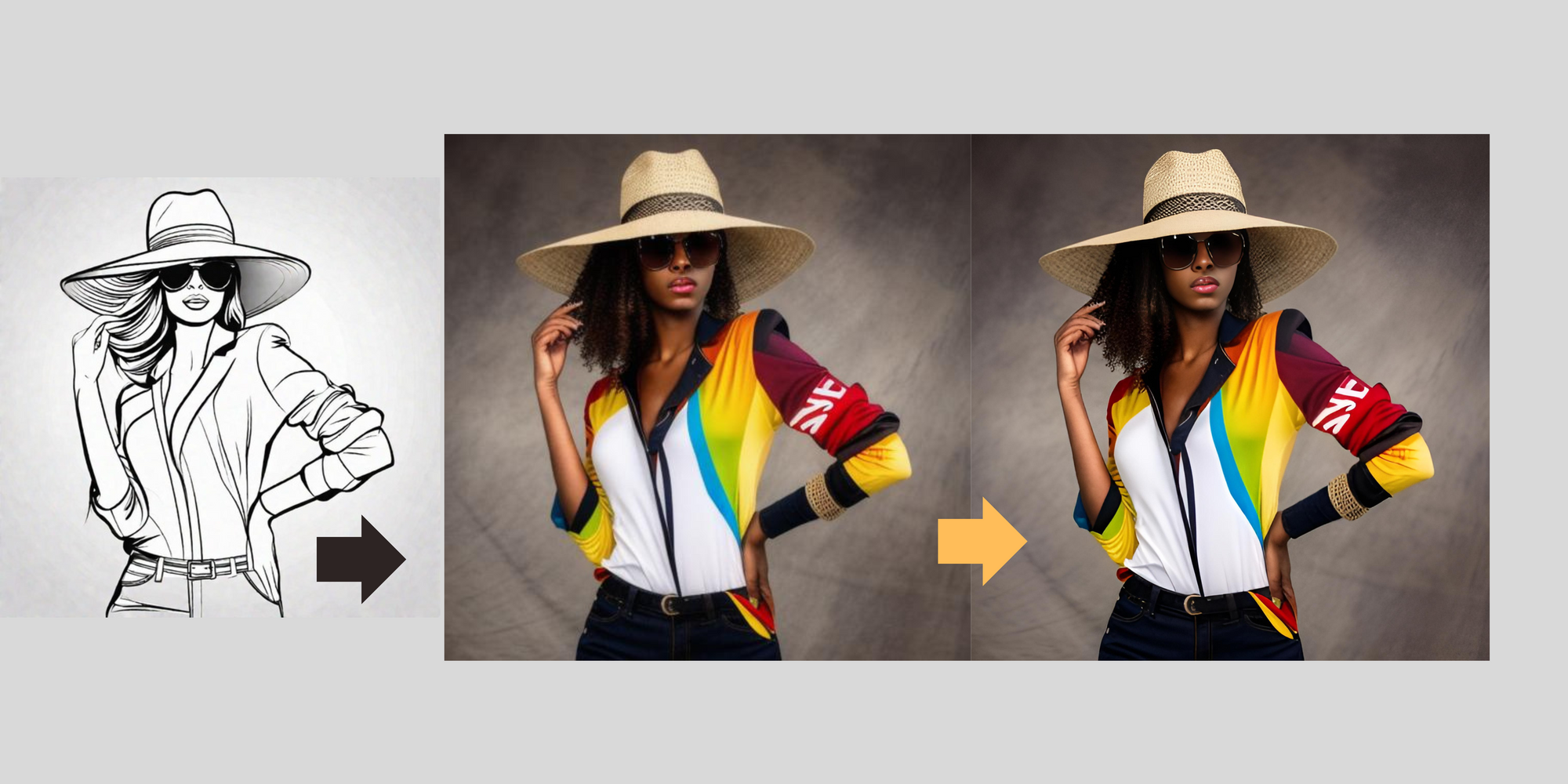
In the context of our blog post, let's explore some scenarios where image quality plays a pivotal role, particularly for visuals:
1. E-commerce: In the world of online shopping, image quality is paramount. Enhanced visuals can showcase products in finer detail, aiding potential buyers in making informed decisions.
2. Real Estate: High-quality images are essential for property listings. Crisp, sharp visuals can provide prospective buyers with a better understanding of the properties they are considering.
3. App/Website Graphics: In the digital sphere, engaging visuals are crucial for user retention. Sharper images can enhance the overall user experience and make apps and websites more appealing.
4. Social Sharing: Social media relies heavily on visual content. Images that are clear and vibrant can garner more attention and engagement, benefiting individuals and businesses alike.
5. Photo Prints: For those who cherish physical photographs, image quality is of utmost importance. Enhanced visuals can ensure that printed photos capture memories in the best possible way.
Conclusion:
As we've seen, the need for image enhancement transcends industry boundaries, finding relevance in a myriad of practical applications. The importance of a clear image is paramount in our digital age. Whether it's online shopping, real estate, or various digital platforms, a high-quality image can make all the difference. If your stable diffusion workflow operates in these domains, integrating models like ESRGAN and Codeformer into Stable Diffusion can be a game-changer, significantly enhancing your image quality. In conclusion, it's not just about having good visuals; it's about optimizing them for real-world applications, ensuring the best user experience and interaction with digital content.