Best settings for Stable Diffusion SDXL 1.0
This article delves deep into the intricacies of the SDXL model, its architecture, and the optimal settings to harness its full potential.
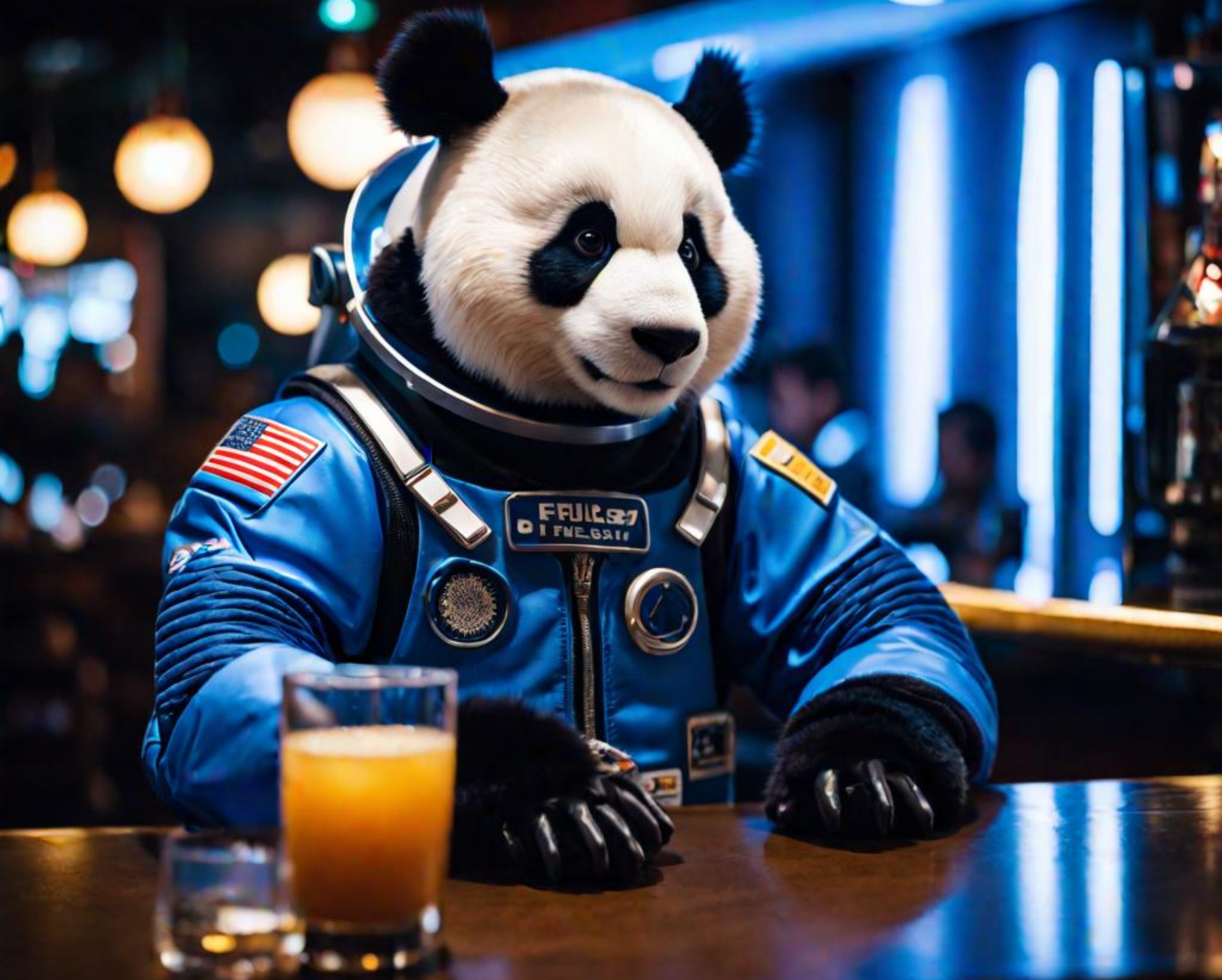
The introduction of Stable Diffusion SDXL 1.0 by Stability marks a significant milestone. This article delves deep into the intricacies of this groundbreaking model, its architecture, and the optimal settings to harness its full potential.
A successor to the Stable Diffusion 1.5 and 2.1, SDXL 1.0 boasts advancements that are unparalleled in image and facial composition. This capability allows it to craft descriptive images from simple and concise prompts and even generate words within images, setting a new benchmark for AI-generated visuals in 2023.
SDXL 1.0: Technical architecture and how does it work
The architecture of SDXL has undergone some major upgrades. It employs a larger UNet backbone, which houses an increased number of attention blocks and an extended cross-attention context. This is made possible due to its second text encoder. The model operates on a mixture-of-experts pipeline for latent diffusion. Initially, the base model generates noisy latents, which are then refined in the subsequent denoising steps.
The essence of the Stable Diffusion model lies in its unique approach to image generation. Unlike traditional methods that rely on labeled data, Stable Diffusion focuses on enabling models to learn the intricate details of images. This is achieved through a two-phase diffusion process:
- Forward Diffusion: Here, an image is taken and a controlled amount of random noise is introduced.
- Reverse Diffusion: The aim here is to denoise the image and reconstruct its original content.
The U-Net plays a pivotal role in this process. It is trained to predict noise from a randomly noised image and calculate the loss between the predicted and actual noise. Over time, with a large dataset and multiple noise steps, the model becomes adept at making accurate predictions on noise patterns.
So what's new in SDXL 1.0?
With SDXL, an additional text encoder was introduced, which is trained against more linguistic prompts, and higher resolutions in comparison to the old one. The base model always uses both encoders, while the refiner has the option to run with only one of them or with both. Other improvements include:
Enhanced U-Net Parameters: SDXL 1.0 has a larger number of U-Net parameters, enabling more intricate image generation.
Heterogeneous Distribution of Transformer Blocks: Unlike its predecessors, SDXL 1.0 adopts a non-uniform distribution, paving the way for improved learning capabilities.
Advanced Text Conditioning Encoders: With the inclusion of OpenCLIP ViT-bigG and an additional text encoder, CLIP ViT-L, SDXL 1.0 effectively integrates textual information into the image generation process.
Innovative Conditioning Parameters: The introduction of "Size-Conditioning", "Crop-Conditioning", and "Multi-Aspect Conditioning" parameters allow the model to adapt its image generation based on various cues.
Specialized Refiner Model: This model is adept at handling high-quality, high-resolution data, capturing intricate local details. The Refiner model is designed for the enhancement of low-noise stage images, resulting in high-frequency, superior-quality visuals. The Refiner checkpoint serves as a follow-up to the base checkpoint in the image quality improvement process.
Overall, SDXL 1.0 outshines its predecessors and is a frontrunner among the current state-of-the-art image generators.
Best Settings for SDXL 1.0: Guidance, Schedulers, and Steps
To harness the full potential of SDXL 1.0, it's crucial to understand its optimal settings:
Guidance Scale
Understanding Classifier-Free Diffusion Guidance
Diffusion models are powerful tools for generating samples, but controlling their quality and diversity can be challenging. Traditionally, "classifier guidance" was used, which employs an external classifier to guide the sampling process, ensuring better sample quality. However, this method introduced complexity by necessitating an additional classifier's training. Enter "CLASSIFIER-FREE DIFFUSION GUIDANCE." This innovative approach uses a duo of diffusion models: a conditional one (tailored to specific conditions) and an unconditional one (for freeform generation). By merging the outputs of these two models, we strike a balance between sample quality and diversity, all without the need for an external classifier. This method is not only simpler, as it sidesteps the need for an extra classifier, but it also evades potential adversarial attacks associated with classifier guidance. The trade-off? It might be a tad slower since it involves two forward model passes.
Choosing the Right Guidance Weight
The guidance weight is pivotal in determining the quality and alignment of generated images to the given prompt. Think of it as the dial controlling how closely the generated image adheres to your input. A value of 0 will yield random images, disregarding your prompt entirely. Opt for lower values if you're in the mood for more "creative" outputs, albeit with elements that might stray from your prompt. On the flip side, higher values produce images that mirror your prompt more accurately but might be less imaginative. For those using the SD model, a sweet spot lies between 5-15. Lean towards the lower end for creativity and the higher end for sharper, more precise images.
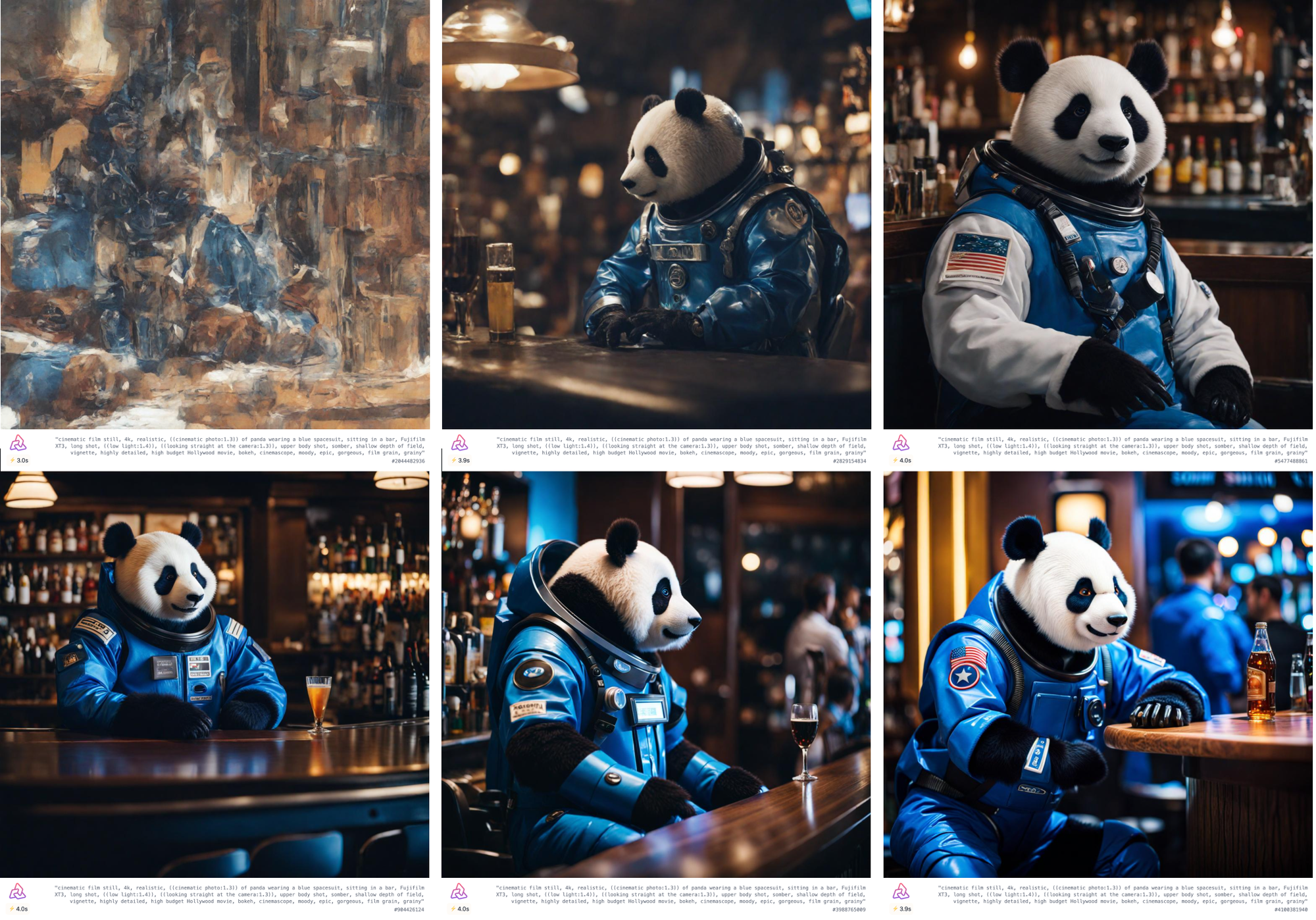
Steps
This refers to the number of denoising steps. Diffusion models are iterative processes. They involve a repeated cycle that begins with random noise generated from a text input. As the process progresses, some of this noise is removed with each step, leading to a progressively higher-quality image.
The "steps" parameter determines how many iterations or cycles the model will undergo. More denoising steps usually lead to a higher quality image at the expense of slower (and more expensive) inference. While a larger number of denoising steps enhance the output quality, it's essential to strike a balance.
For SDXL, around 30 sampling steps are sufficient to achieve good-quality images. After a certain point, each step offers diminishing returns. Above 50, it may necessarily not produce images of better quality.
As mentioned above, SDXL comes with two models. On Segmind, we have a 0.5 factor for the base vs refiner model, and hence the number of steps given as input will be divided equally between the two models. Refer to the high noise fraction section below for more info.
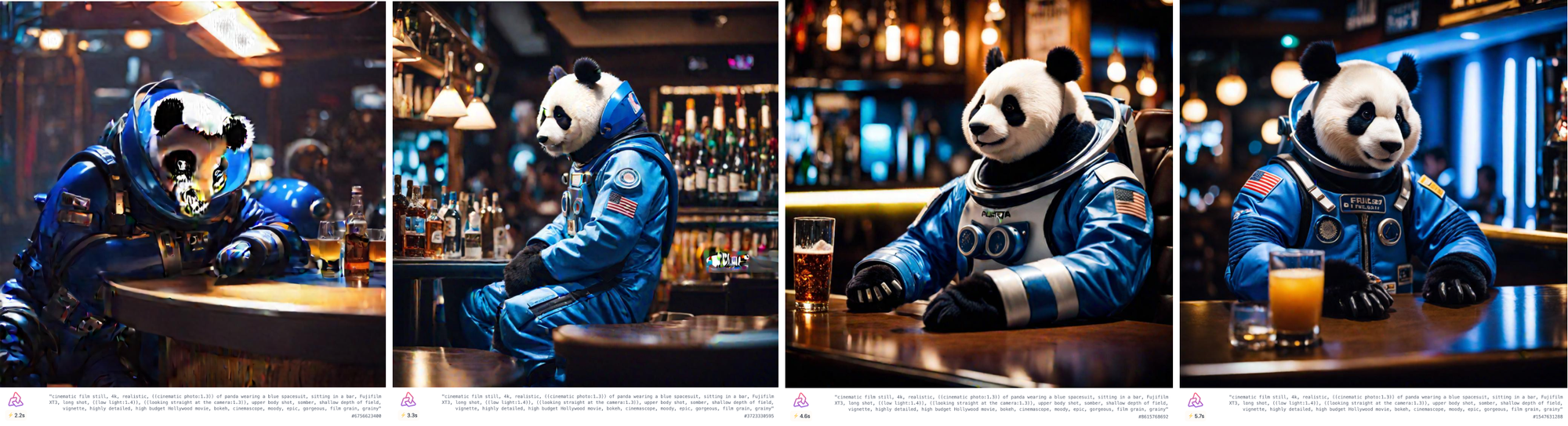
High Noise Fraction: It defines how many steps and what % of steps are to be run on each expert (model), i.e. base and refiner model. Segmind sets this at 0.5 which means 50% of the steps run on the base model and 50% run on the refiner model.
Schedulers
Schedulers in the context of Stable Diffusion are algorithms used alongside the UNet component of the Stable Diffusion pipeline. They play a pivotal role in the denoising process and operate multiple times iteratively (referred to as steps) to produce a clean image from an entirely random noisy one. The primary function of these scheduler algorithms is to progressively perturb data with increasing random noise (known as the “diffusion” process) and then sequentially eliminate noise to generate new data samples. Sometimes, they are also termed as Samplers.
With SDXL 1.0, certain schedulers can generate a satisfactory image in as little as 20 steps. Among them, UniPC and Euler Ancestral are renowned for delivering the most distinct and rapid outcomes compared to their counterparts.
Negative Prompts
A negative prompt is a technique that allows users to specify what they don't want to see in the generated output, without providing any additional input. While negative prompts might not be as essential as the main prompts, they play a crucial role in preventing the generation of undesired or strange images. This approach ensures that the generated content aligns more closely with the user's intent by explicitly excluding unwanted elements.
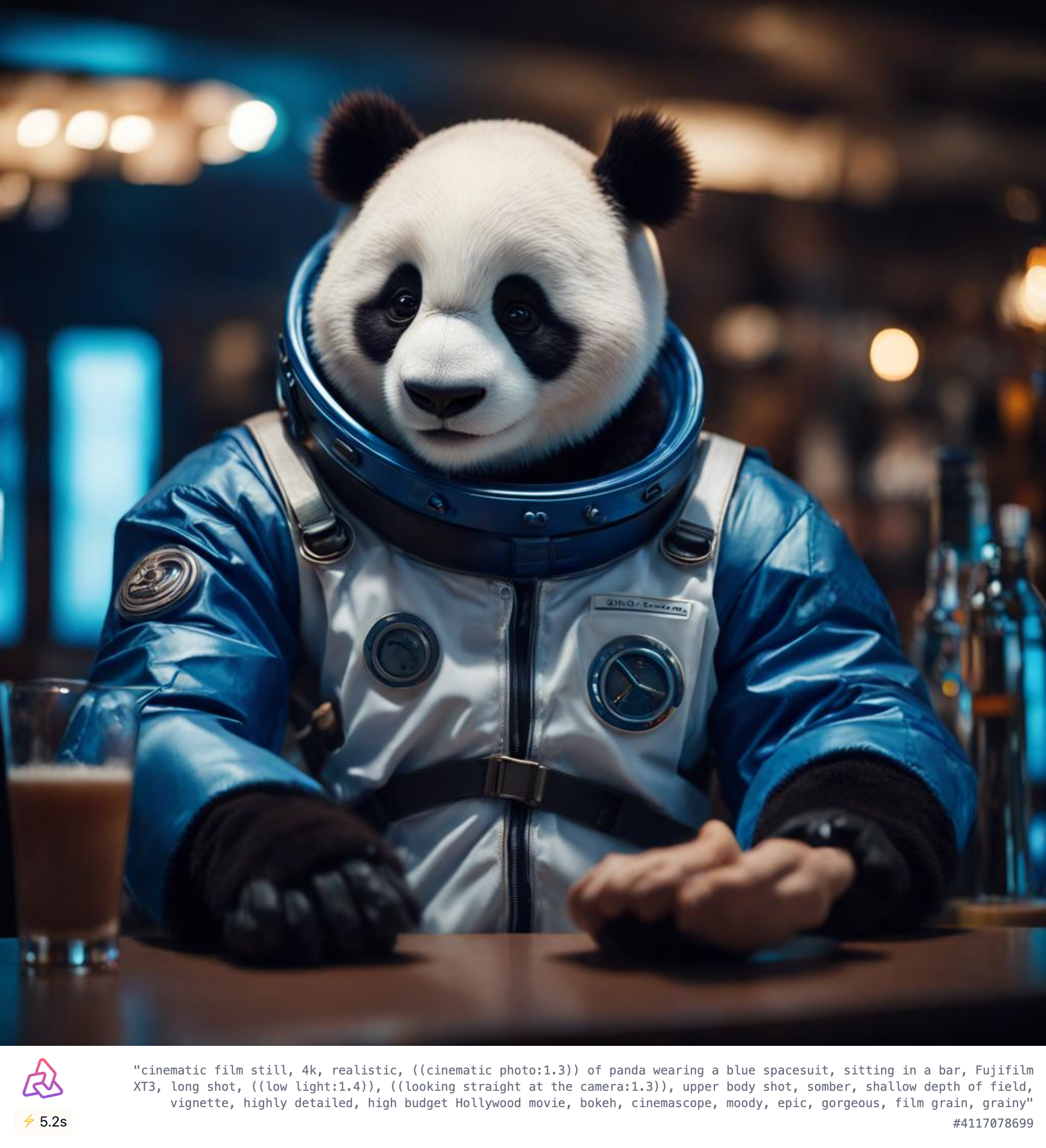
Examples of Commonly Used Negative Prompts:
- Basic Negative Prompts: worst quality, normal quality, low quality, low res, blurry, text, watermark, logo, banner, extra digits, cropped, jpeg artifacts, signature, username, error, sketch, duplicate, ugly, monochrome, horror, geometry, mutation, disgusting.
- For Animated Characters: bad anatomy, bad hands, three hands, three legs, bad arms, missing legs, missing arms, poorly drawn face, bad face, fused face, cloned face, worst face, three crus, extra crus, fused crus, worst feet, three feet, fused feet, fused thigh, three thigh, fused thigh, extra thigh, worst thigh, missing fingers, extra fingers, ugly fingers, long fingers, horn, realistic photo, extra eyes, huge eyes, 2girl, amputation, disconnected limbs.
- For Realistic Characters: bad anatomy, bad hands, three hands, three legs, bad arms, missing legs, missing arms, poorly drawn face, bad face, fused face, cloned face, worst face, three crus, extra crus, fused crus, worst feet, three feet, fused feet, fused thigh, three thigh, fused thigh, extra thigh, worst thigh, missing fingers, extra fingers, ugly fingers, long fingers, horn, extra eyes, huge eyes, 2girl, amputation, disconnected limbs, cartoon, cg, 3d, unreal, animate.
- For Non-Adult Content: nsfw, nude, censored.
At Segmind, our TensorRT pipeline for SDXL 1.0 offers twice the inference speed compared to the PyTorch version, ensuring blazing-fast performance.
In conclusion, Stable Diffusion SDXL 1.0 is a testament to the strides AI has made in the realm of image generation. With its advanced architecture, innovative features, and optimal settings, it promises to be a game-changer in the visual arts landscape.