CodeFormer: The best tool for restoring and enhancing facial features
CodeFormer recovers degraded images without clear references, overcoming challenges of mapping degraded inputs to high-quality outputs. CodeFormer captures subtle details in low-quality facial images, providing a robust solution for face restoration.
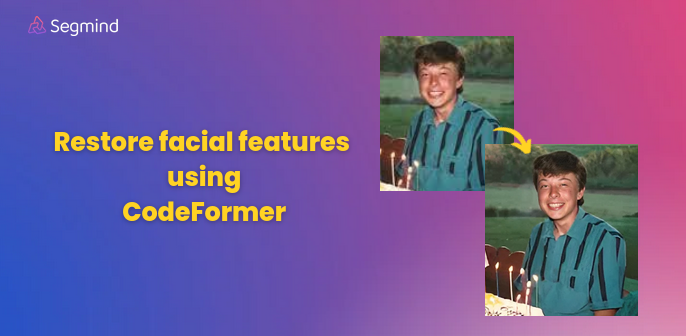
The process of blind face restoration through which we would be able to recover images that have undergone degradations is extremely complex and it does it without access to clear references.
The challenges in the restoration process often stem from the lack of well-defined mappings from degraded inputs to desired outputs, leading to a noticeable loss of high-quality details from the original images. To tackle these intricacies head-on, CodeFormer was introduced. This model uniquely captures the subtle compositions and details present in low-quality facial images, establishing itself as a robust solution for the complexities associated with face restoration.
In the previous blog, we got to take a look at how the model can be used to enhance old photos that are degraded and improve their quality. We will get to look at various examples of how CodeFormer can be used to transform blurry images and enhance them.
We will get to use the best settings to restore the old photographs using the insights we gained while experimenting with the mode.
The Architecture of CodeFormer:
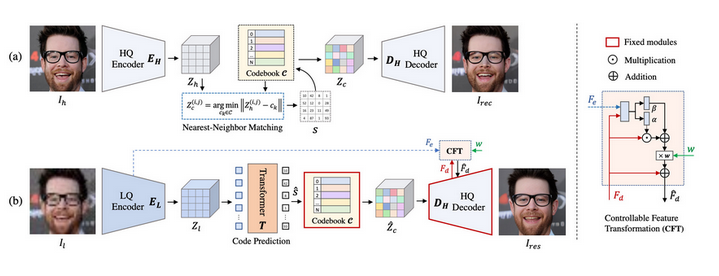
A discrete codebook is first established and a decoder learns through the process of self-reconstruction learning to store the high-quality visual components of the facial images. Once the codebook and decoder are fixed, the transformer module is introduced which helps in predicting the code sequence thus enabling face composition for low-quality inputs.
A controllable feature transformation module is present which helps in regulating the information flow from the low-quality encoder to the decoder. It's crucial to note that this connection is optional and can be disabled to prevent adverse effects, particularly in cases of severely degraded inputs.
Problems with blurry images:
Blurry images present a variety of challenges. The foremost problem lies in the loss of sharpness and fine details, blurring the boundaries between objects and essential details. This lack of clarity affects not only the aesthetics but also the interpretability of the image, making it challenging for viewers to pick out details.
Blurring can occur due to various factors such as motion, defocus, or lens aberrations, each posing a unique set of challenges. Additionally, the ambiguity in blurry images makes it challenging to establish clear reference points for restoration. Unlike well-defined mappings in other image enhancement tasks, the lack of distinct features in blurry images complicates the process of predicting and reconstructing details accurately.
In essence, the restoration process for blurry images requires navigating through a maze of uncertainties.
Restoring blurry images using CodeFormer:
Take this image of actor Gary Oldman, where the image is a bit blurry, and the challenges are evident in the loss of sharpness and fine details. However, CodeFormer helps in resolving this problem.
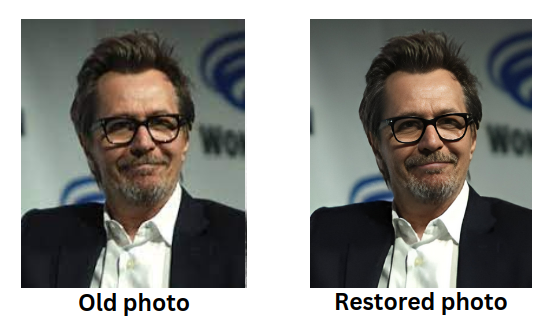
We can see how the lack of details that are submerged in the old photo, the texture of the skin, and clarity near the face have improved due to the ability of CodeFormer which consists of the transformer module that helps in global face composition. Not only does this improve the intricate details but it also enhances the details such as texture, and depth while preserving the quality of the original image as well.
Here we get to take a look at an old photograph of the Indian tabla player and Grammy award winner Zakir Hussain, the image has a noticeable loss in quality, leaving behind a diminished visual representation where intricate details seem to have faded. The challenges inherent in low-quality images are particularly evident in this case.
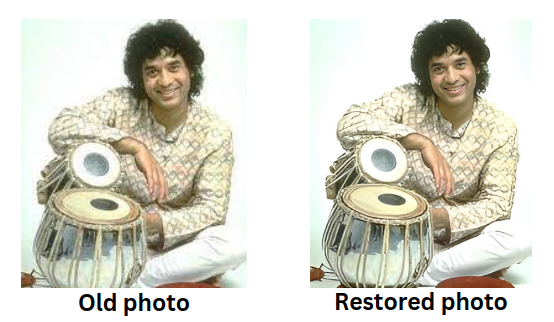
CodeFormer offers a promising solution to the old photograph. We can witness how it can reconstruct the areas around his face and the faded details that were once present around his face now seem to be reconstructed with more clarity and have a more sharper and refined presence. The lighting around the table has also improved and the contours are well-defined.
In this disfigured and poor-quality old image of the legendary rockstar Elvis Presley, the image is marred by distortions and diminished quality obscuring the charisma and vibrancy that defined Elvis Presley's rock 'n' roll era.
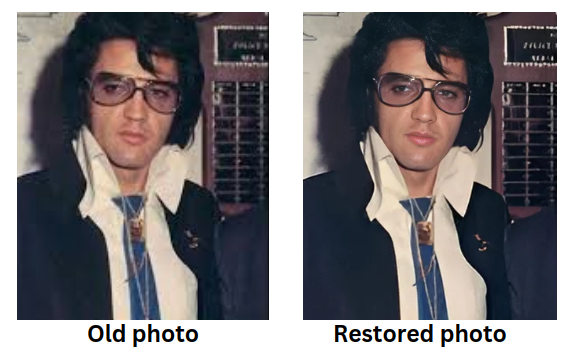
In the restored image, the disfigured features of the rockstar begin to regain their original form. The transformation extends to the texture of the hair, where the restoration process brings forth a heightened level of detail and sharpness compared to the original, worn photograph.
This image capturing a young Diana, presents a low-resolution depiction of her youth. The photograph seems to have been captured during an era with constrained technological improvements suffering from pixelation and color grading.
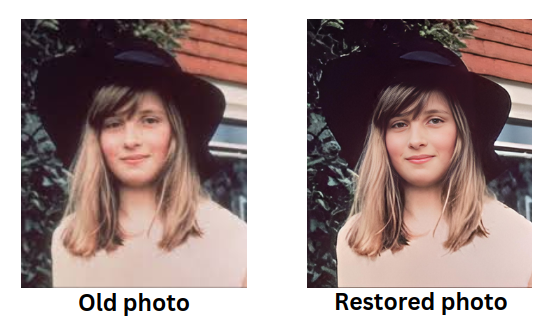
We can witness how the restored image cannot only enhance but also restore the originality of the era in which it has been captured. The restored image has improved the blurry effect present in the old photo but has also enhanced the texture of her hair and skin thus capturing the youthfulness and the charm of the subject.
Here is a vintage image of the iconic actress Marilyn Monroe bears the mark of time with a dull and lackluster presentation. The features, once vibrant and captivating, now appear subdued and faded, with blurry textures affecting the essence of Marilyn's signature hairstyle.
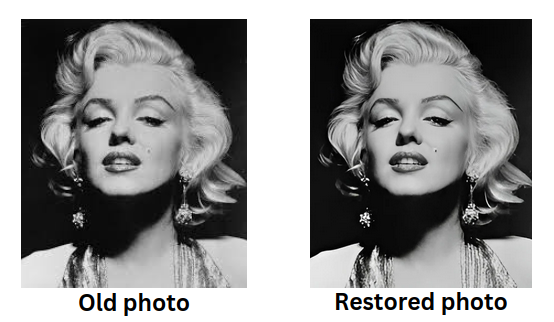
In the restoration process, Codeformer delicately addresses the dullness, breathing new life into the image. The blurry textures that once masked the intricacies of Marilyn's hair are refined. The contours of her jawline and the sparkle in her eyes are enhanced ensuring that the essence of Marilyn’s beauty is not just maintained but elevated.
Finally, let's take this image of a young Elon Musk captured smiling, the image consists of blurry and saturated colors. The blurred details and excessive color saturation contribute to a diminished visual quality.
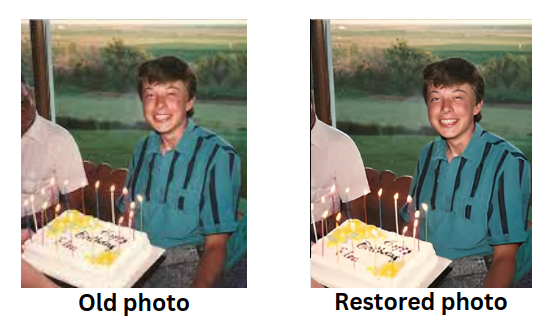
The restored image enhances the clarity and depth of the old photograph while simultaneously increasing the details, the once faded lighted candles now radiate casting a warm glow.
Conclusion :
CodeFormer helps in the restoration of blurry images, seamlessly combining both preservation as well as improvement of the existing image. By addressing blurriness and enhancing visual clarity, Codeformer not only preserves the authenticity of historical or iconic visuals but also elevates them to a new level of vibrancy. The model's advanced algorithms and self-reconstruction learning adapt to the challenges posed by photography.
Moreover, Codeformer excels in handling inconsistencies within images such as variations in lighting, contrast, or color saturation.