The Lifecycle of a Generative AI Model: From Idea to Deployment
The Lifecycle of a Generative AI Model: From Idea to Deployment
Generative Artificial Intelligence (AI) is a subfield of AI that focuses on creating new content, such as music, images, and texts, using deep learning algorithms. It is a rapidly growing area, with applications ranging from art and entertainment to scientific discovery and beyond. In this blog post, we will explore the lifecycle of a generative AI model, from idea to deployment.
A Brief History of Generative AI
Generative AI has its roots in the 1950s, with the creation of the first AI systems. Early AI systems were rule-based and had limited capabilities, but the advent of deep learning algorithms in the late 20th century enabled the creation of more sophisticated generative AI models. In the last decade, advances in hardware and software have enabled the creation of large-scale generative AI models that can produce high-quality content in real-time.
What does this mean?
As AI models have gotten progressively larger, they have begun to surpass major human performance benchmarks. This matters because the fields that generative AI addresses—knowledge work and creative work—comprise billions of workers. Generative AI can make these workers at least 10% more efficient and/or creative: they become not only faster and more efficient but more capable than before. Therefore, Generative AI has the potential to generate trillions of dollars of economic value.
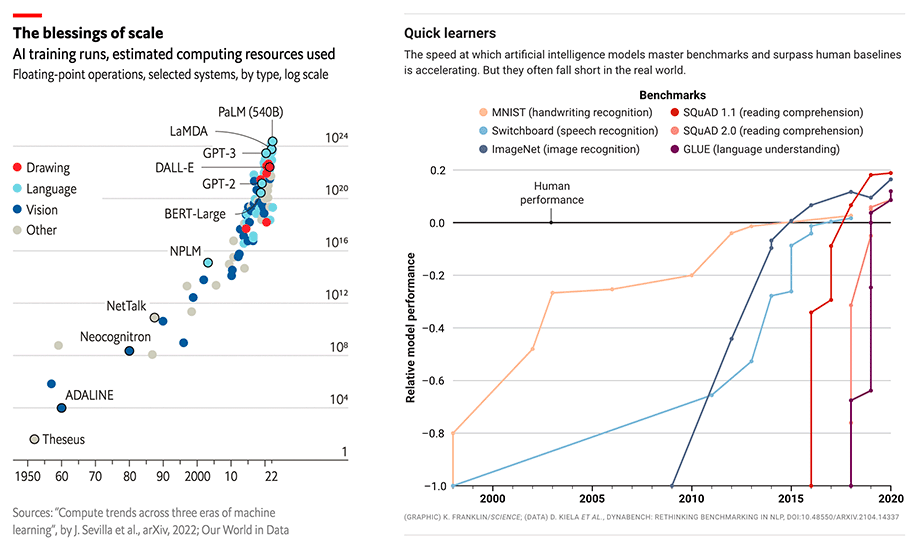
The Lifecycle of a Generative AI Model: From Idea to Deployment
Step 1: Ideation
The first step in the lifecycle of a generative AI model is to formulate an idea. This could be inspired by a specific problem you want to solve or a creative idea that you want to explore. It's important to consider the goals of your model and to research existing models to determine if your idea has already been explored.
Step 2: Data Collection and Preparation
Once you have a clear idea of what you want to achieve, the next step is to gather and prepare the data that your model will use to generate content. This involves selecting a dataset that is relevant to your goal and pre-processing it so that it can be used to train the model.
Step 3: Model Design and Training
Once you have your data ready, the next step is to design and train your model. This involves selecting the appropriate deep learning architecture, such as a Generative Adversarial Network (GAN) or a Variational Autoencoder (VAE), and using your pre-processed data to train the model. This step can take several weeks or even months, depending on the complexity of your model and the size of your dataset.
Step 4: Model Evaluation
After your model has been trained, the next step is to evaluate its performance. This involves using quantitative metrics, such as accuracy and precision, to determine the quality of the content generated by your model. Additionally, you may also use qualitative methods, such as user testing or expert review, to gain a more in-depth understanding of the strengths and weaknesses of your model.
Step 5: Deployment
Once you are satisfied with the performance of your model, the final step is to deploy it. This involves integrating your model into a larger system, such as a website or a mobile app, so that it can be used by end-users. Additionally, you may also need to perform ongoing maintenance and updates to your model to ensure that it continues to perform well over time.
Conclusion
The lifecycle of a generative AI model is a complex and iterative process that requires careful planning and execution. However, the end result can be a powerful tool for creating new and innovative content that has the potential to transform entire industries. Whether you are exploring new creative avenues or solving real-world problems, the potential of generative AI is virtually limitless.
About Segmind
Segmind is the first serverless platform for optimized generative AI models.
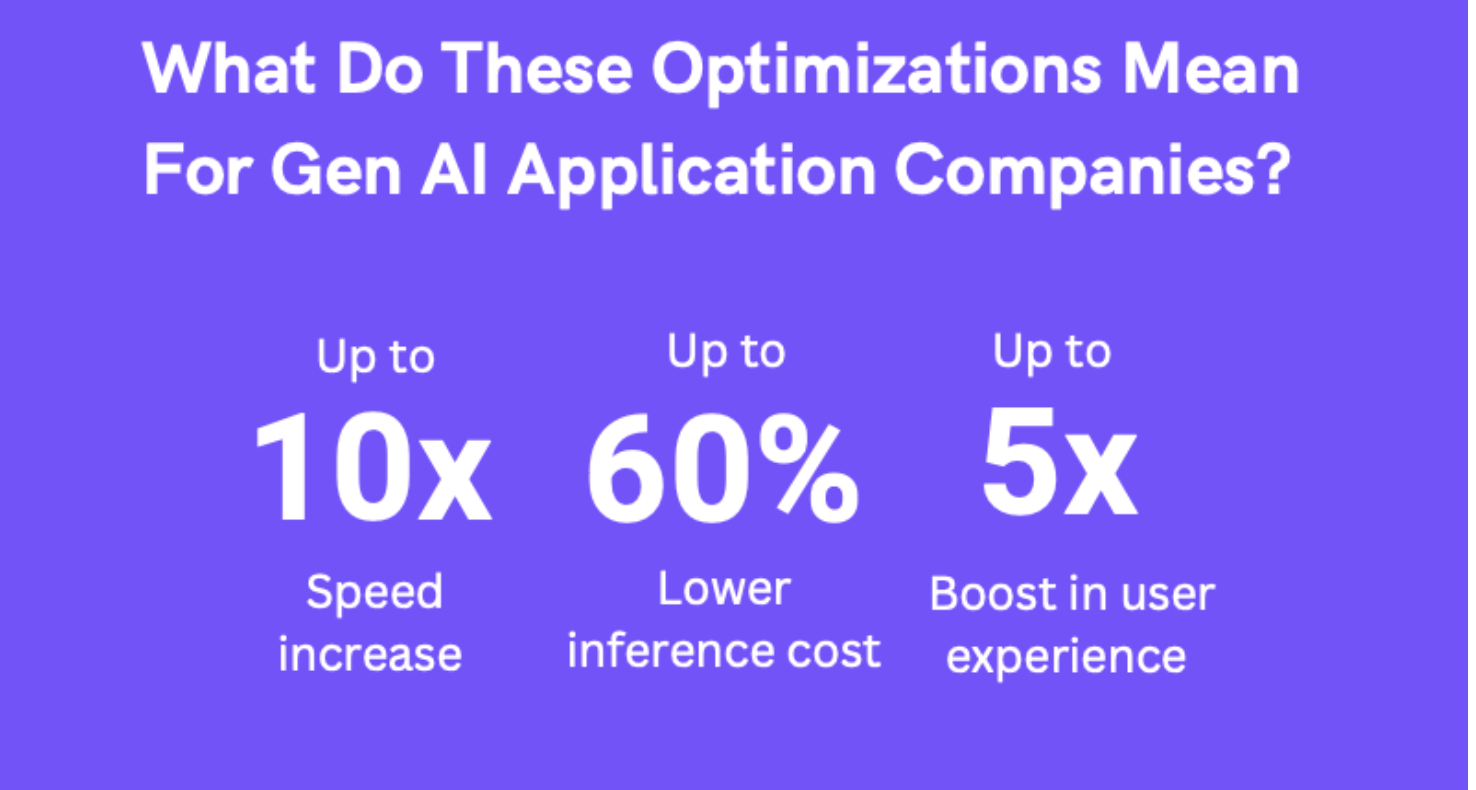
About voltaML
VoltaML is a lightweight library to convert and run your ML/DL deep learning models in high-performance inference runtimes like TensorRT, TorchScript, ONNX, and TVM.
Today voltaML offers the fastest stable diffusion inference library with 0.8s generation time. Check out more information about voltaML here.