SSD-1B ControlNets Comparison
In this blogpost, we do a comparision of ControlNet models - Canny & Depth, based on Segmind Stable Diffusion (SSD-1B) framework.
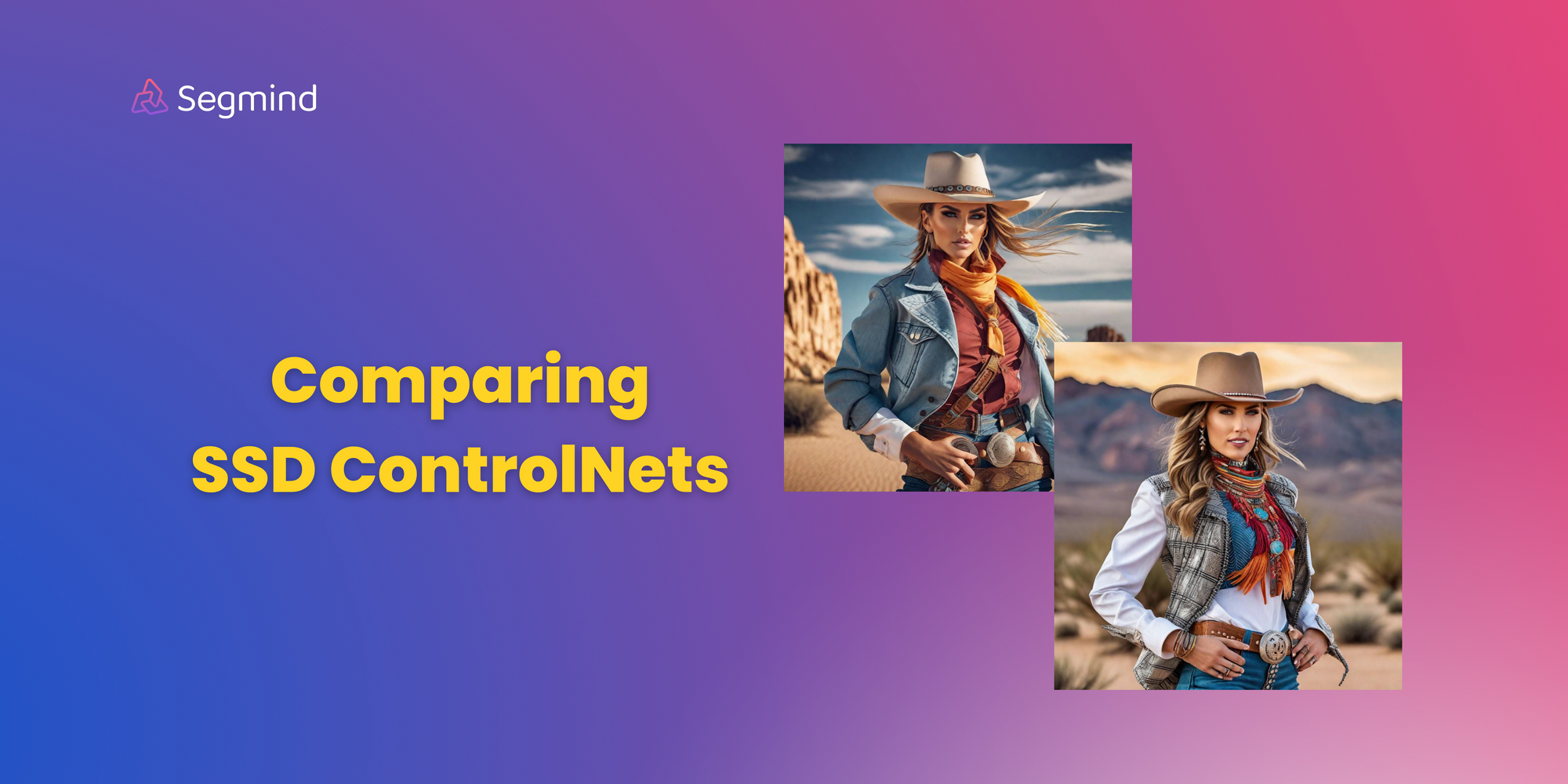
In this blog post, we'll delve into two in-house ControlNet models: SSD-Depth and SSD-Canny. These models are designed to elevate your images and create immersive visual experiences.
The SSD Depth models bring about transformations using a depth map, while the SSD Canny utilizes canny edge detection for image transformations. By the end of this post, you'll gain valuable insights to help you choose the ideal model for enhancing your images.
Working of SSD Canny:
The training algorithm of SSD-1B relies on Canny edges as a fundamental element to instruct the model in creating images with corresponding edges.
Essentially, SSD-1B Canny learns from Canny edges data, aiding the model in recognizing crucial image features and seamlessly incorporating them into Stable Diffusion model outputs. This process not only enhances the model's precision in replicating edges but also refines its comprehension of essential image characteristics.
As a result, the SSD-1B model excels in generating images that faithfully replicate provided edges, demonstrating a heightened level of accuracy and relevance to the input data's underlying features.
Working on SSD Depth:
SSD Depth enhances the model by integrating SSD-1B with the ControlNet model, combining the efficient architecture of SSD-1B to reduce inference time with the ControlNet architecture.
The SSD-1B Model serves as the foundation for producing high-quality images, while the ControlNet layer enhances the pictures by incorporating depth information. This involves introducing random fuzziness or noise to a clear picture, gradually turning it into a blurry state. The process, known as denoising, involves repeatedly removing the introduced fuzziness or noise. Ultimately, the depth maps introduce layers to a flat image, creating a more realistic sensation. Throughout each denoising step, the depth map, working in tandem with a text prompt, plays a crucial role in the enhancement process.
Parameters of both these models:
- Negative prompts: Negative prompts enable users to specify the type of image they want to avoid during the generation process without providing any input. These prompts act as a guide for image generation, directing the process to exclude certain elements based on the user-provided text. By using negative prompts, users can efficiently prevent the generation of specific objects and styles, address image abnormalities, and improve overall image quality.
- Inference Steps: This parameter focuses on the number of denoising steps, representing the iterations in a process initiated by random noise from the text input. In each iteration, the model refines the image by gradually removing noise. A higher number of steps leads to the increased production of high-quality images.
- Guidance scale: They introduce layers to a flat image, creating a more realistic sensation. Throughout each denoising step, the depth map, working in tandem with a text prompt, plays a crucial role in the enhancement process.
- ControlNet scale: This parameter shapes how closely the image generation process follows both the input image and the given text prompt. A higher value strengthens the alignment between the generated image and the provided image-text input. Nevertheless, setting the value to the maximum isn't always recommended. While higher values may impact the artistic quality of the images, they don't necessarily capture the intricate details required for a comprehensive output.
- Scheduler: In the Stable Diffusion pipeline, schedulers play a crucial role alongside the UNet component, particularly in the denoising process conducted iteratively across multiple steps. These steps are essential for transforming a randomly noisy image into a clean, high-quality image. Schedulers systematically eliminate noise from the image, generating new data samples in the process. Noteworthy schedulers in this context, such as Euler and UniPC, are generally highly recommended and may vary for specific contexts.
Image comparisons between both models:
Now, let's assess how both of these models compare based on a couple of key factors:
- Prompt Adherence: Evaluating how well the models adhere to the given instructions.
- Art Styles and Functionality: Examining the variety and quality of artistic styles the models can produce.
This analysis will provide a comprehensive understanding of the strengths each model brings to the table. It's worth noting that all parameter values in both models are fixed according to their optimal settings.
Prompt Adherence:
Prompt adherence gauges how effectively a model follows given text instructions to craft an image. It's crucial to understand that there isn't a specific framework or precise metric for evaluating prompt adherence in text-to-image generation models. Thoughtfully crafted prompts act as a roadmap, guiding the model to produce images aligned with the intended vision.
Let's begin with simple prompts for images that don't specify a lot of details.
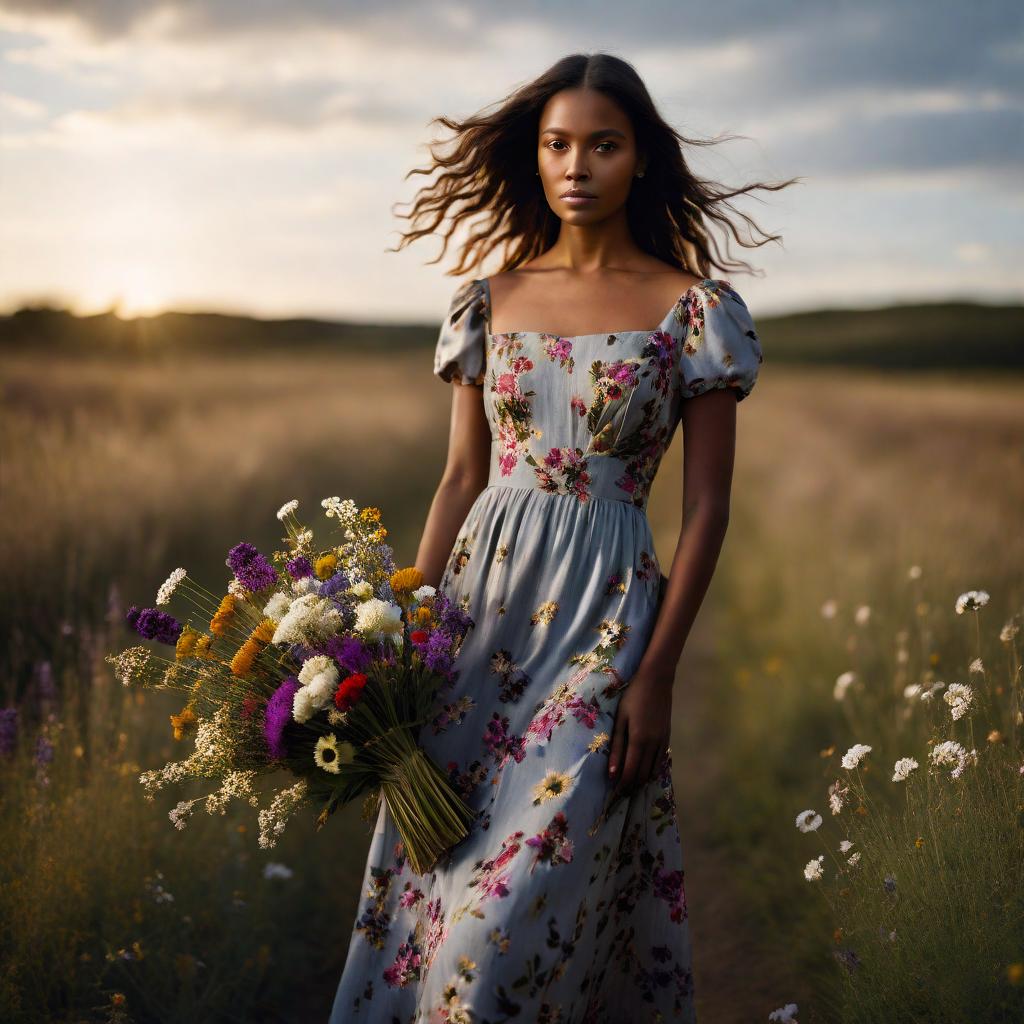
Prompt : An ultradetailed stylish girl wearing streetwear in New York
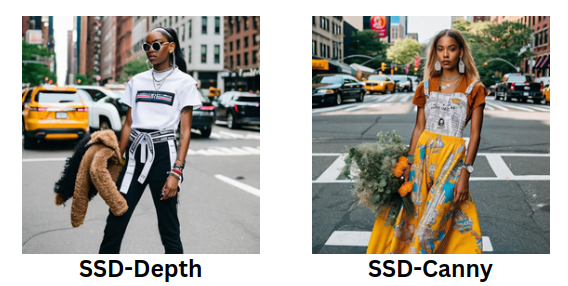
We observe the variations generated by both models to the input image. Notably, the subject and the surroundings change both models. However, it's worth mentioning that the object held by the subject in the image differs only in SSD-Depth.
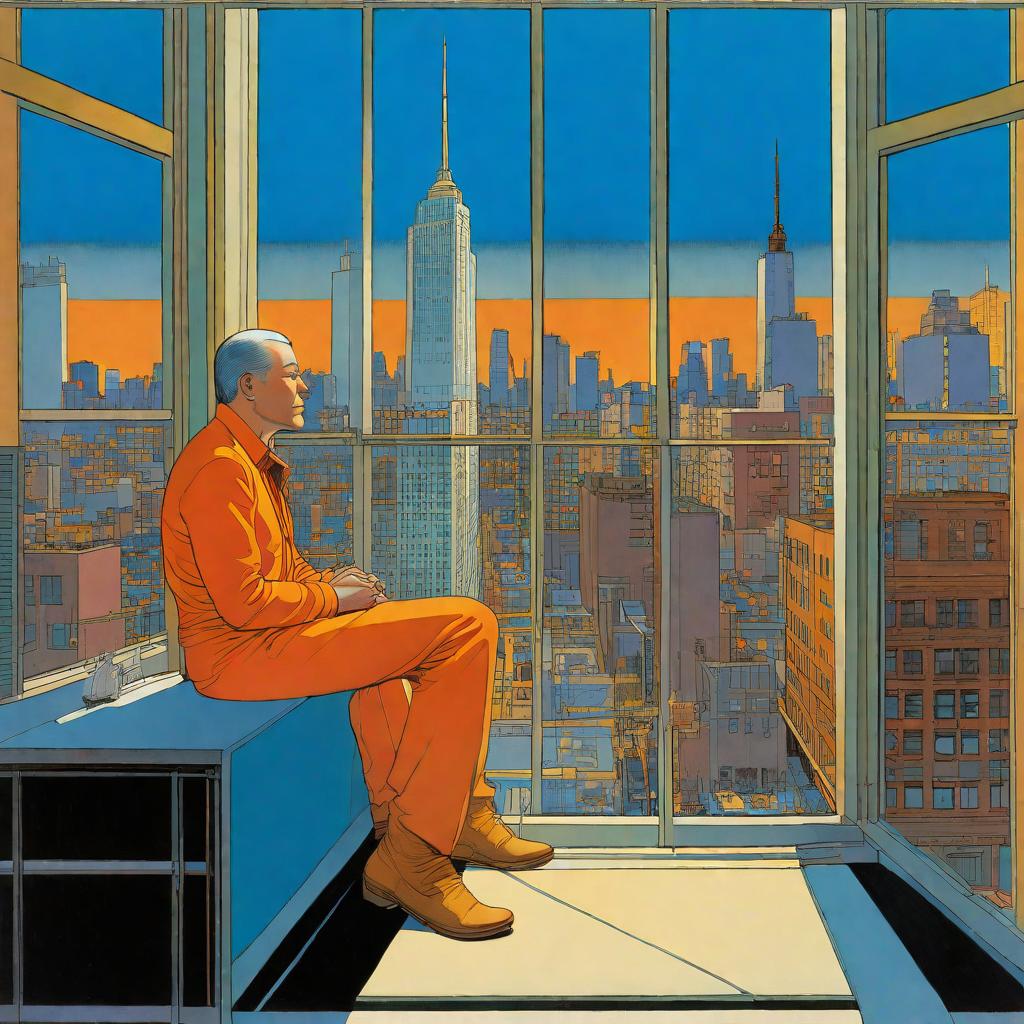
Prompt : A guy sitting alone in front of huge windows watching the sunset , cinematic lighting
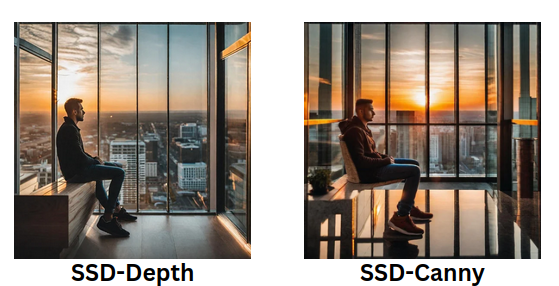
From the above two pictures, it is pretty clear as to the respective functioning of the models as mentioned in the working of the models. SSD-Canny uses canny edge detection i.e. generates boundaries of what is going to appear on the final image from the edges present in the input image. While the SSD-Depth model helps in understanding the distance at which parts of images are present.
Let us move on to a few complex prompts where we get to specify the details much more clearly
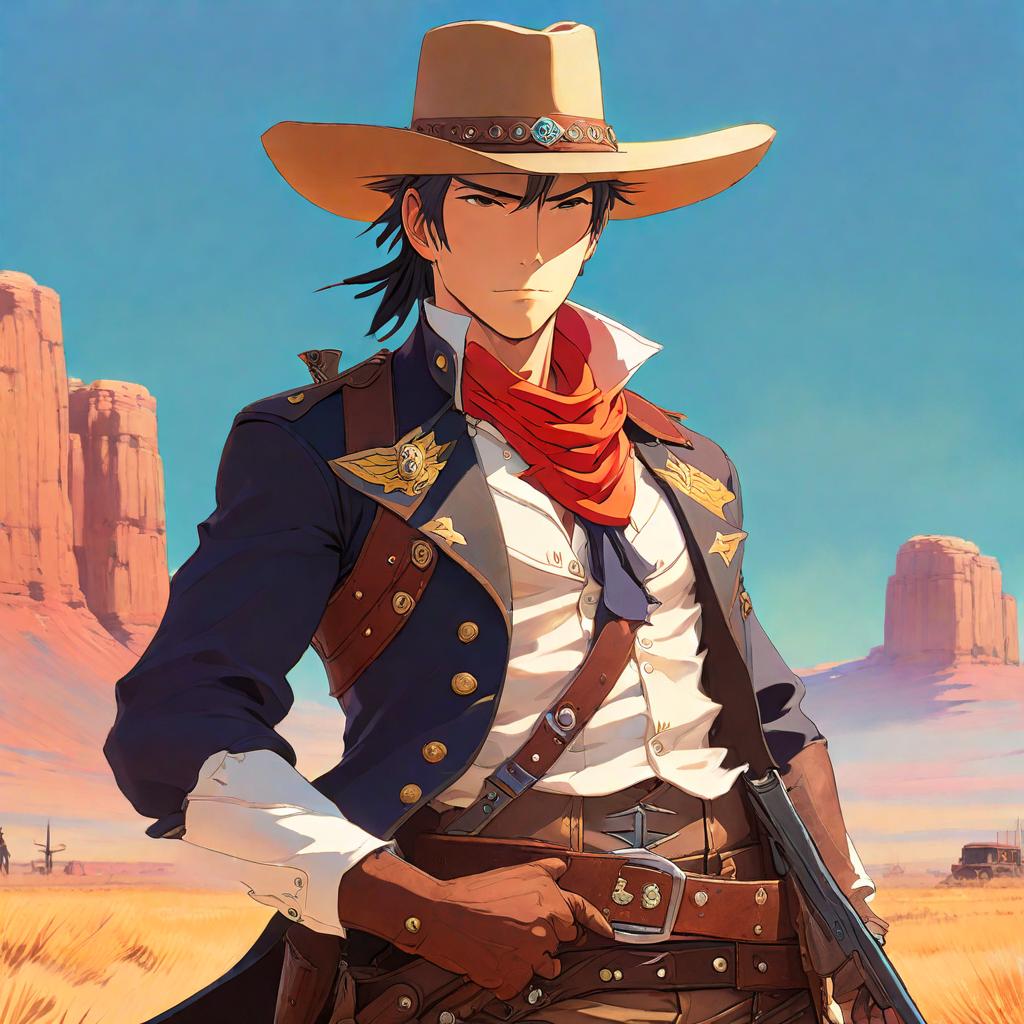
Prompt : Wild west Gunslinger holding a gun , cyberpunk , portrait , vibrant , neon lighting , 4k
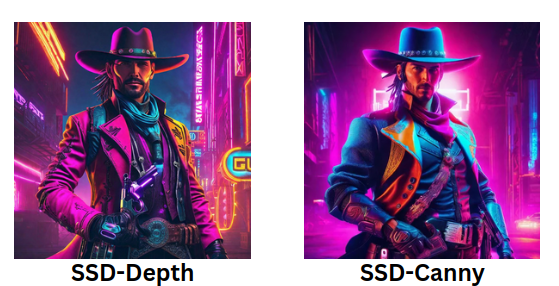
Here we notice that SSD-depth provides a more 3D-rendered image, adding a layer of sophistication.
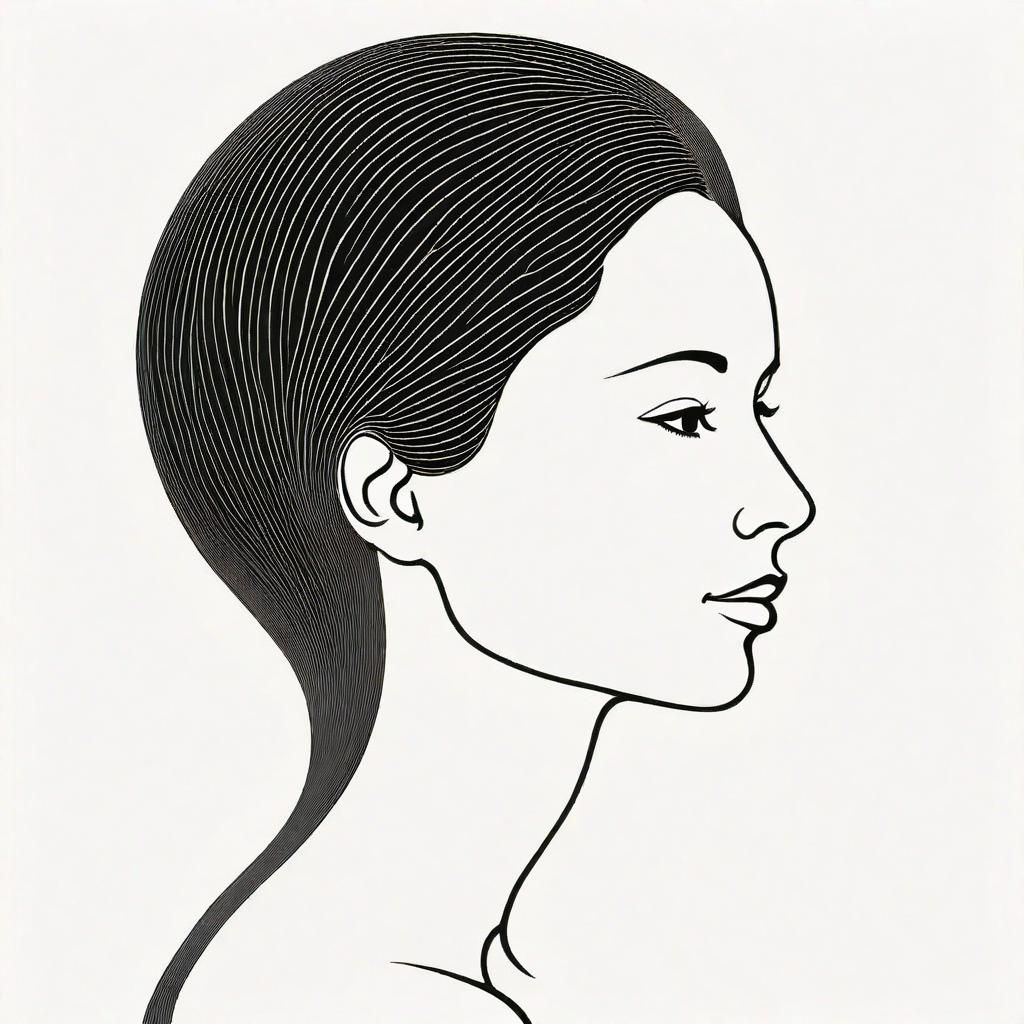
Prompt : rural african woman wearing traditional clothing. the woman should have dark skin and short, black hair. she should be wearing a brightly colored headwrap and a flowing, patterned dress. the background should be a rural African village
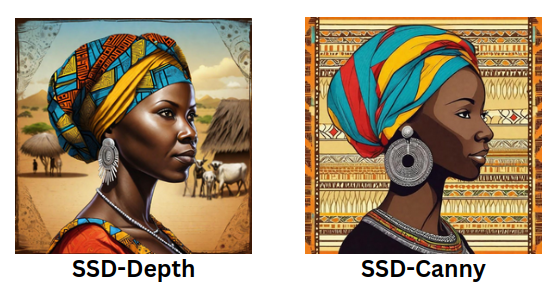
It's noticeable that SSD-Depth has captured and rendered the essence of the last line of the prompt related to the rural African village. In contrast, SSD-Canny excels in understanding and reproducing the edges present in the input image, aligning with its inherent strength in edge detection and transformation.
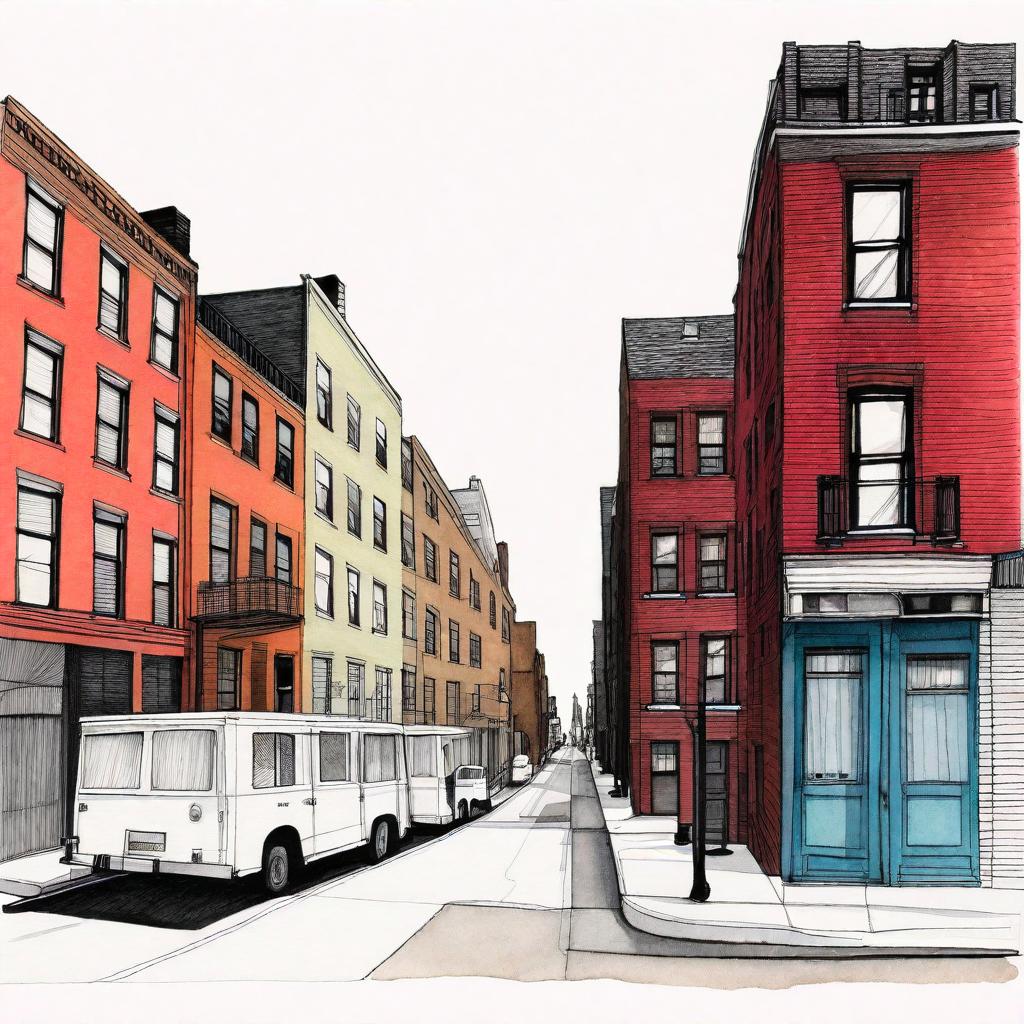
Prompt : American neighbourhood, digital painting, gorgeous, sharp focus , intricate , small and detailed houses
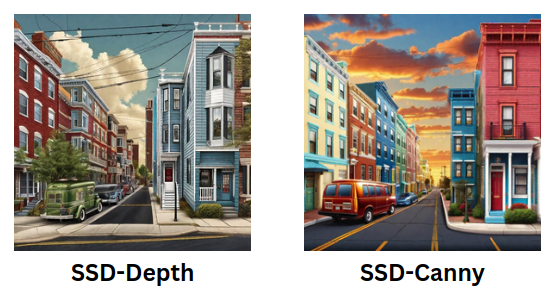
While the SSD-Canny images stand out with their abundance of details and structured appearance, creating a well-defined visual output. On the other hand, SSD-Depth tends to render images with more emphasis on texture and a smoother overall aesthetic.
Now, let's venture into exploring various art styles and prompts to evaluate how each model performs in different scenarios.
Art Styles and Functionality :
Exploring the diverse art styles and the models' proficiency in handling various prompts allows us to understand the full extent of their artistic skills. Continuing our exploration, we will present different prompts to the models, testing their abilities like our evaluation of the previous criteria. This approach enables a comprehensive understanding of how each model responds to different creative challenges and prompts.
Let us now try to modify this image into a rabbit wearing a jacket had it been drawn by Studio Ghibli
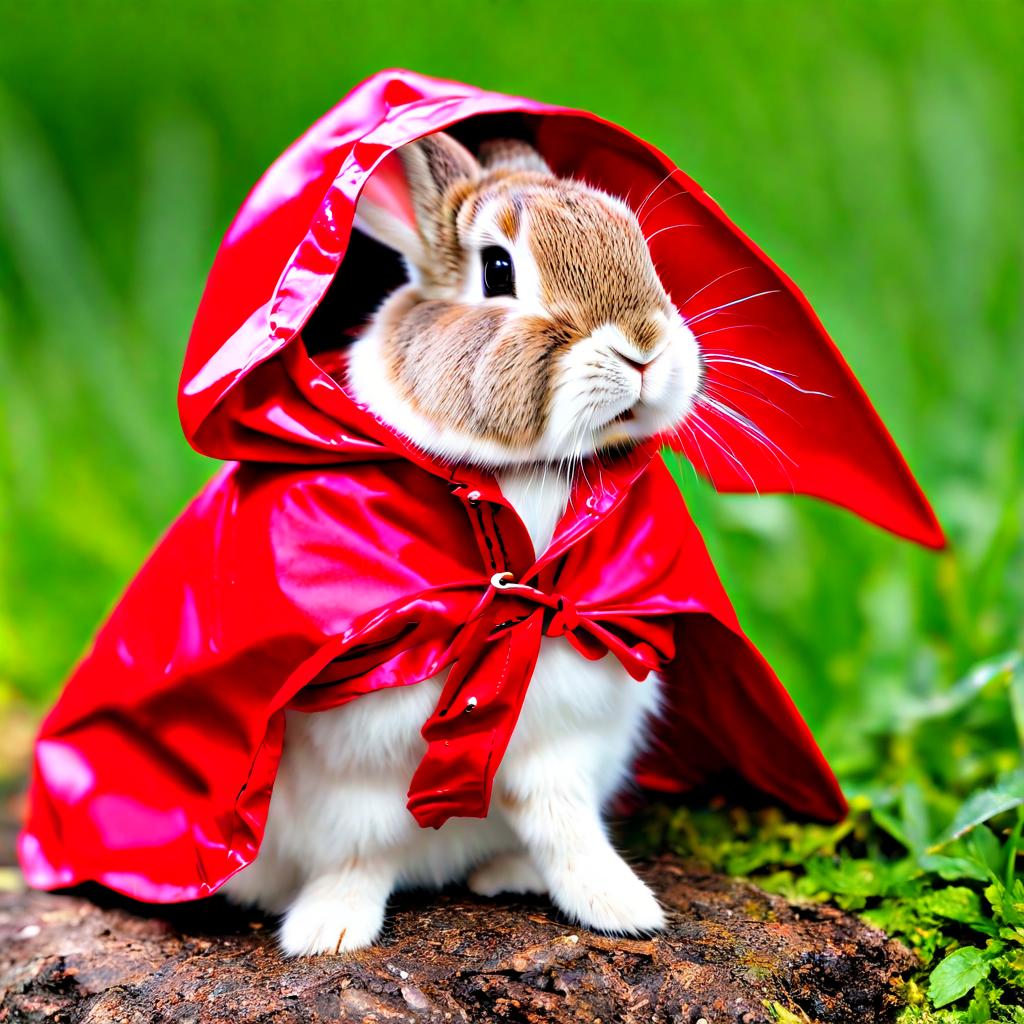
Prompt : Photo of the rabbit as a punk with a jacket , simple art inspired by studio ghibli's visual style
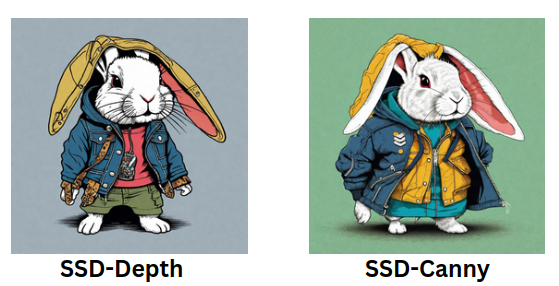
Interesting to note how the ears have been shaped in SSD-Canny to how the ear is inside the attire in SSD-Depth.
Moving on let us now modify this image such that the background is changed into a garden and it is also shot on ARRI
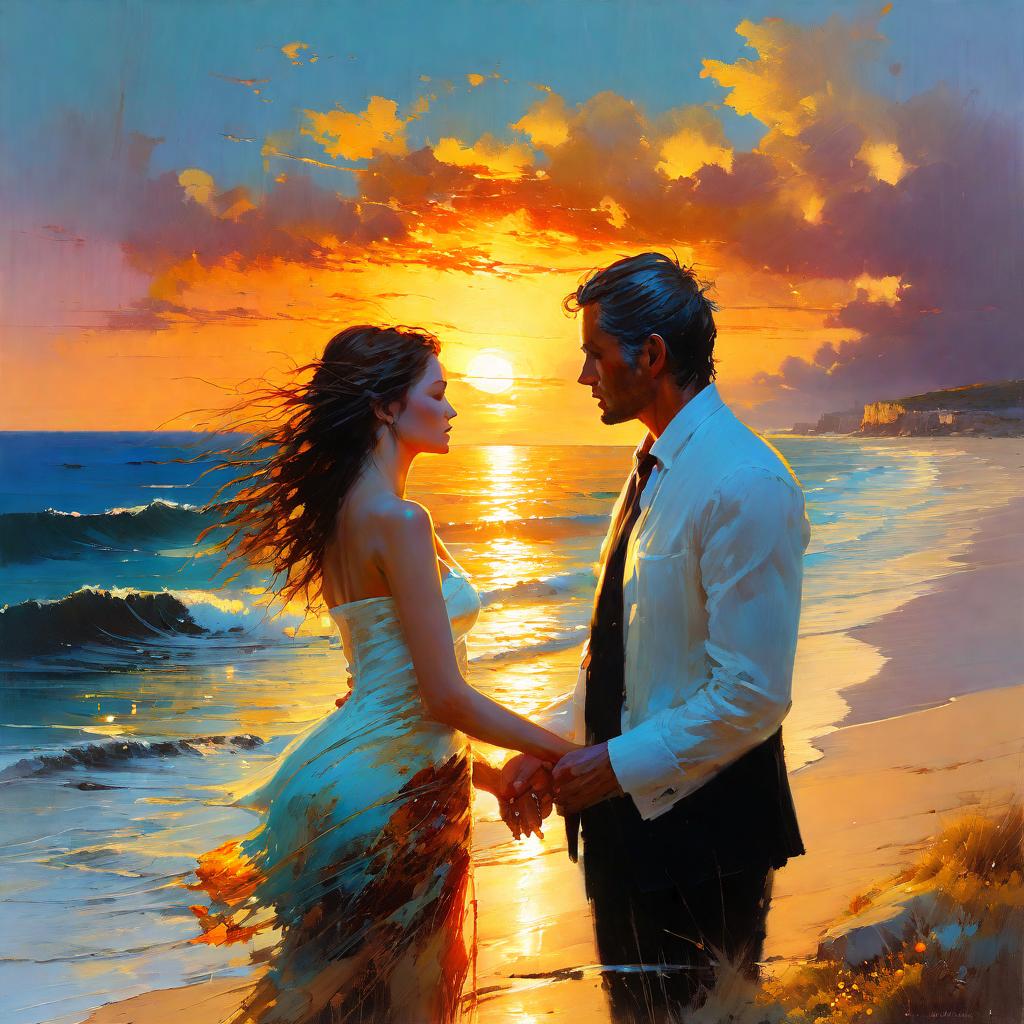
Prompt : Lovers standing together by the garden , photography ,photorealistic , deep and saturared colours , sharp focus, 4k uhd, cinematic lighting, relaxed , photos taken by ARRI
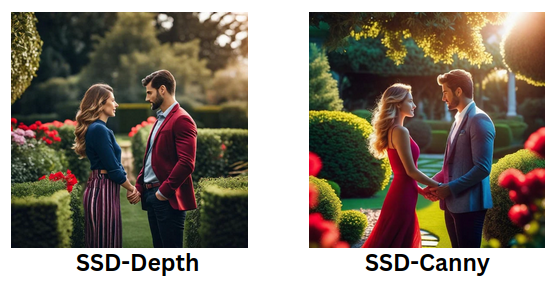
We can see how SSD-Depth here gets to render an image that is more saturated and smoothened.
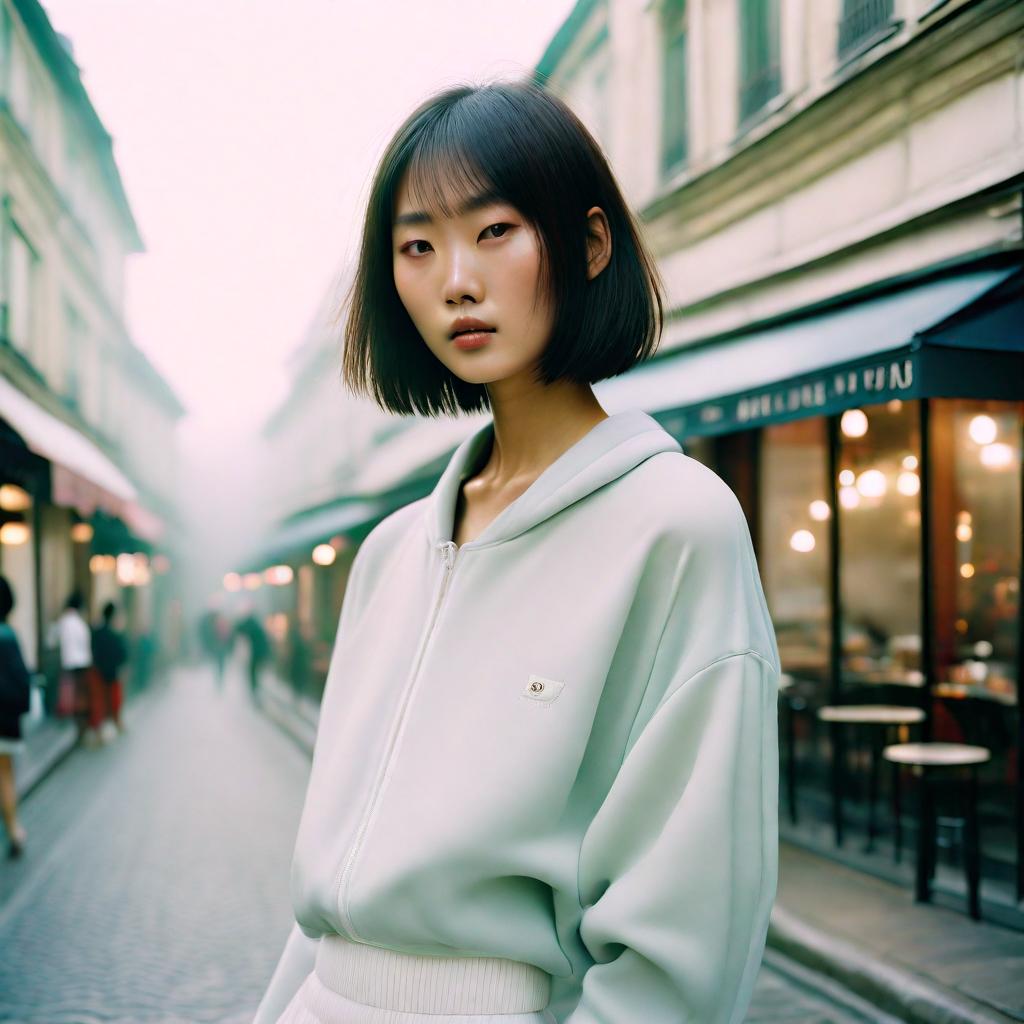
For the above image, we will try modifying it into a image consisting of a girl with a black complexion in a watercolor clip art style.
Prompt : Stylish black girl with trendy outfit, studio lighting highlighting her features, watercolor clip art, vector image, flat white background
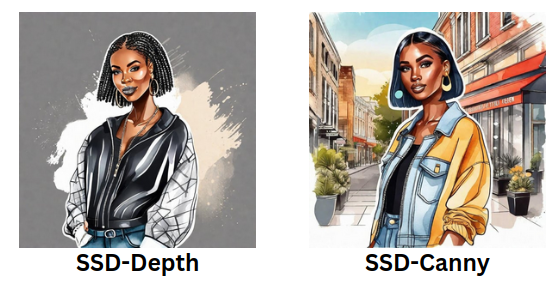
Conclusion
The rendered images from both models showcase their impressive ability to produce high-quality artistic outputs. It's fascinating to observe that these models employ distinct methodologies to achieve this visual excellence.
SSD-Canny relies on canny edge detection, allowing for precise highlighting of contours within the images. Conversely, the SSD-Depth model utilizes depth maps to delineate image boundaries, creating a unique approach to visual representation.
Ultimately, the choice between these models depends on your specific use case, with each offering limitless possibilities for creative expression and image transformation.