How Seed Parameter Influences Stable Diffusion Model Outputs
The seed parameter in Stable Diffusion plays a crucial role in ensuring reproducibility of AI results. It introduces a controlled randomness, enabling the generation of diverse yet replicable outcomes. Understanding its function is key to harnessing the full potential of Stable Diffusion.
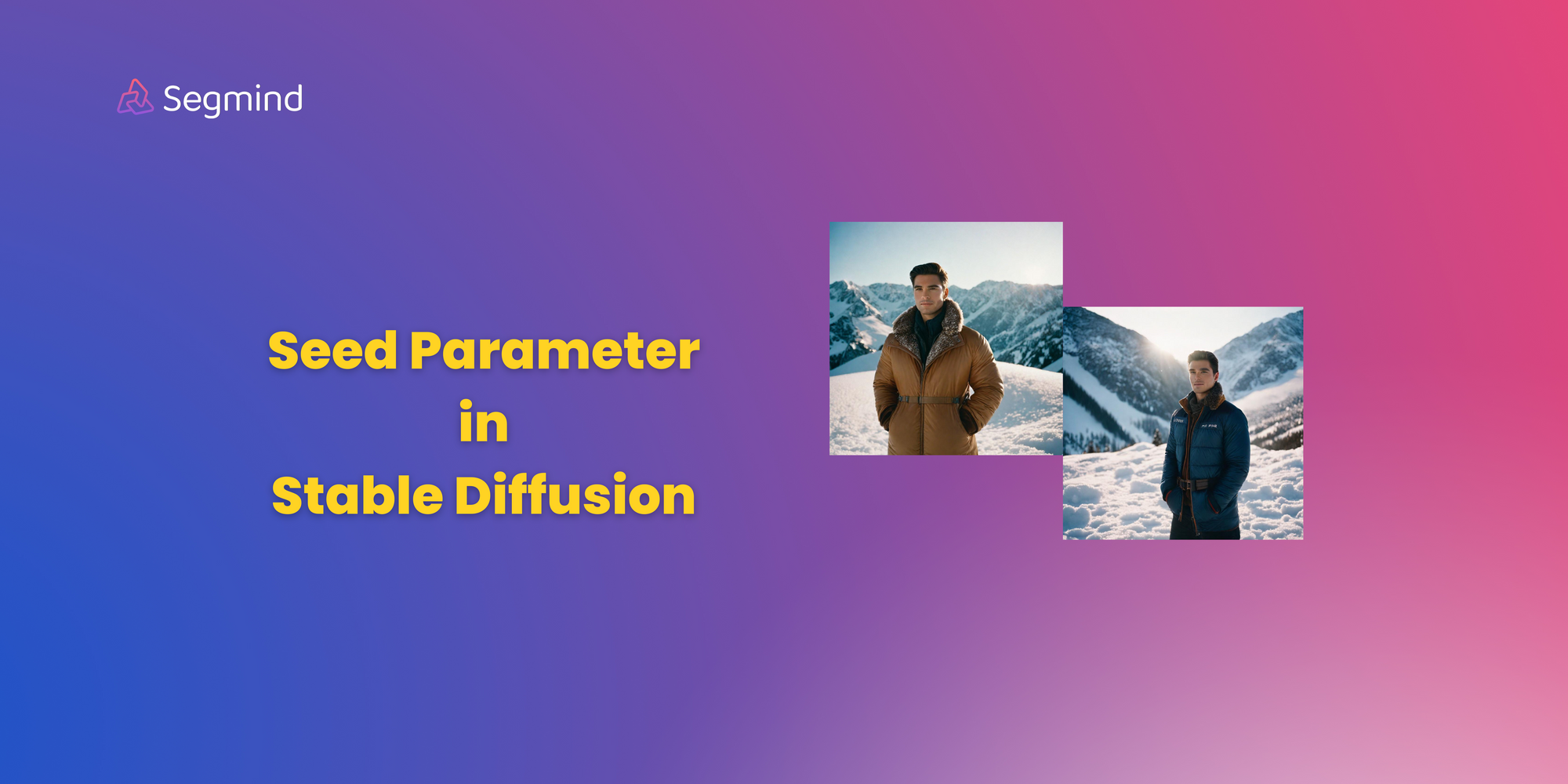
The seed parameter plays a pivotal role in the process image generation with Stable Diffusion. The seed parameter is a numerical value that initializes the generation of an image. It is randomly generated when not specified, but controlling the seed can help generate reproducible images, experiment with other parameters, or prompt variations.
The seed parameter’s most significant attribute is its ability to reproduce the same image when used with the same parameters and prompt. This reproducibility is crucial in AI, especially when experimenting with different parameters or variations. For instance, with a specific seed and a prompt for a ‘portrait of a girl’, one can modify the same character by changing only the text prompt modification, thereby altering the facial expression while keeping the character consistent.
How Seed Parameter Influences Stable Diffusion Model Outputs
The seed parameter in Stable Diffusion models plays a crucial role in influencing the output of the model. It acts as an initial input for the random number generator used in the model, which in turn influences the randomness in the image generation process. This randomness is what allows the model to generate a wide variety of images from a single prompt.
The seed parameter’s influence on the model’s output can be seen when generating multiple similar variations of an image. For instance, by changing the look of the whole image, one can generate a ‘tree’ during each of the four seasons or apply different artistic styles to a ‘sunset’ painting. This is possible because the seed parameter controls the randomness in the image generation process, allowing for the creation of diverse images from a single prompt.
Optimizing Seed Parameter for Consistent Results
To optimize the seed parameter, one approach is to perform a grid search or a random search over a range of seed values. This allows you to observe the impact of different seeds on the generated images. By comparing the images generated with different seeds, you can identify the seed values that produce the most desirable results.
Another approach is to use a technique called ‘seeding’. This involves starting the image generation process with a pre-existing image rather than a random seed. The pre-existing image acts as a ‘seed’, guiding the Stable Diffusion process towards generating images that are similar to the initial image.
It’s also important to note that while the seed parameter can help achieve consistency, it’s not the only factor that influences the outcome. Other parameters, such as the prompt and the model’s hyperparameters, also play a significant role in the image generation process.
Comparative Analysis of Different Seed Settings
Let’s consider an example where we are using the Stable Diffusion model to generate images of a ‘sunset’. We’ll use three different seed settings for this comparative analysis.
Prompt : Sunset
- Seed 1: With the first seed setting, the model looks like natural photograph captured during a beautiful sunset over a vast landscape. The foreground tends to feature a field of meadow filled with lush greens and is bathed in a warm golden light of the setting sun. The sun itself is prominently visible casting long shadows across the scene.
- Seed 2: Using the second seed setting with the same ‘sunset’ prompt, the model might generate a slightly different image. The foreground now consists of tall palm trees surrounded by lush greens against a vibrant sunset sky . The scene also features a body of water with rocky outcroppings.
- Seed 3: With the third seed setting, the image generated different again. The image shows a vibrant orange sun low on the horizon, partially obscured by clouds. The sky is filled with scattered clouds tinged in shades of orange, pink, and grey from the setting sun's rays.
The important point which needs to be noted here is the fact that the different values of seed produces different images.
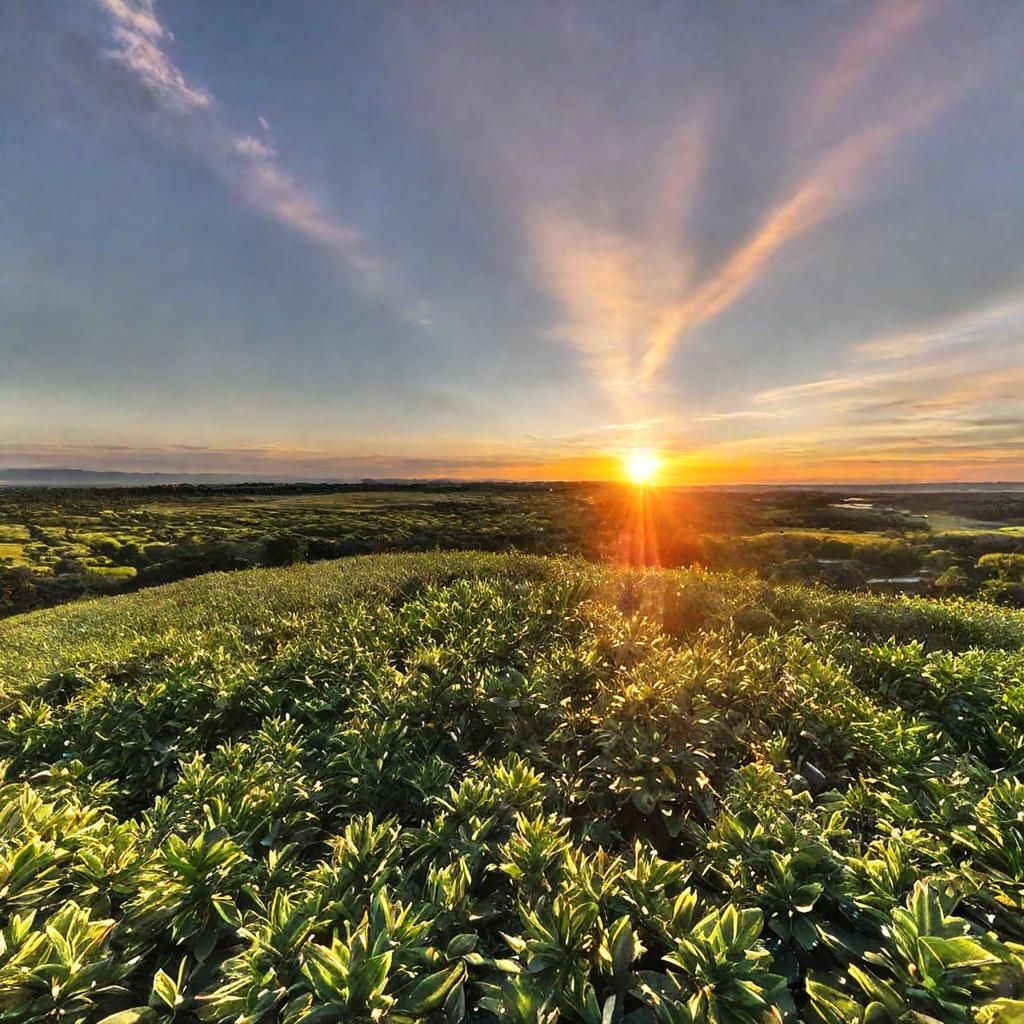
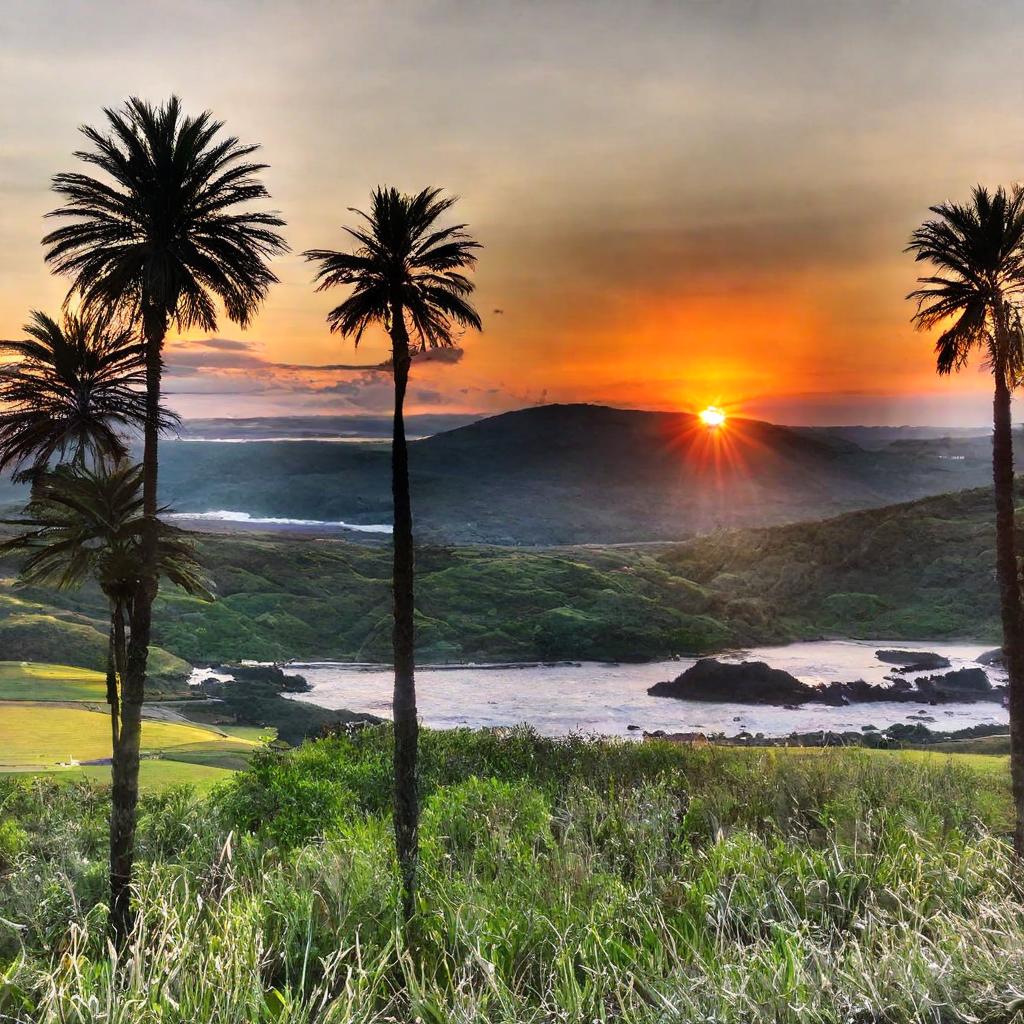
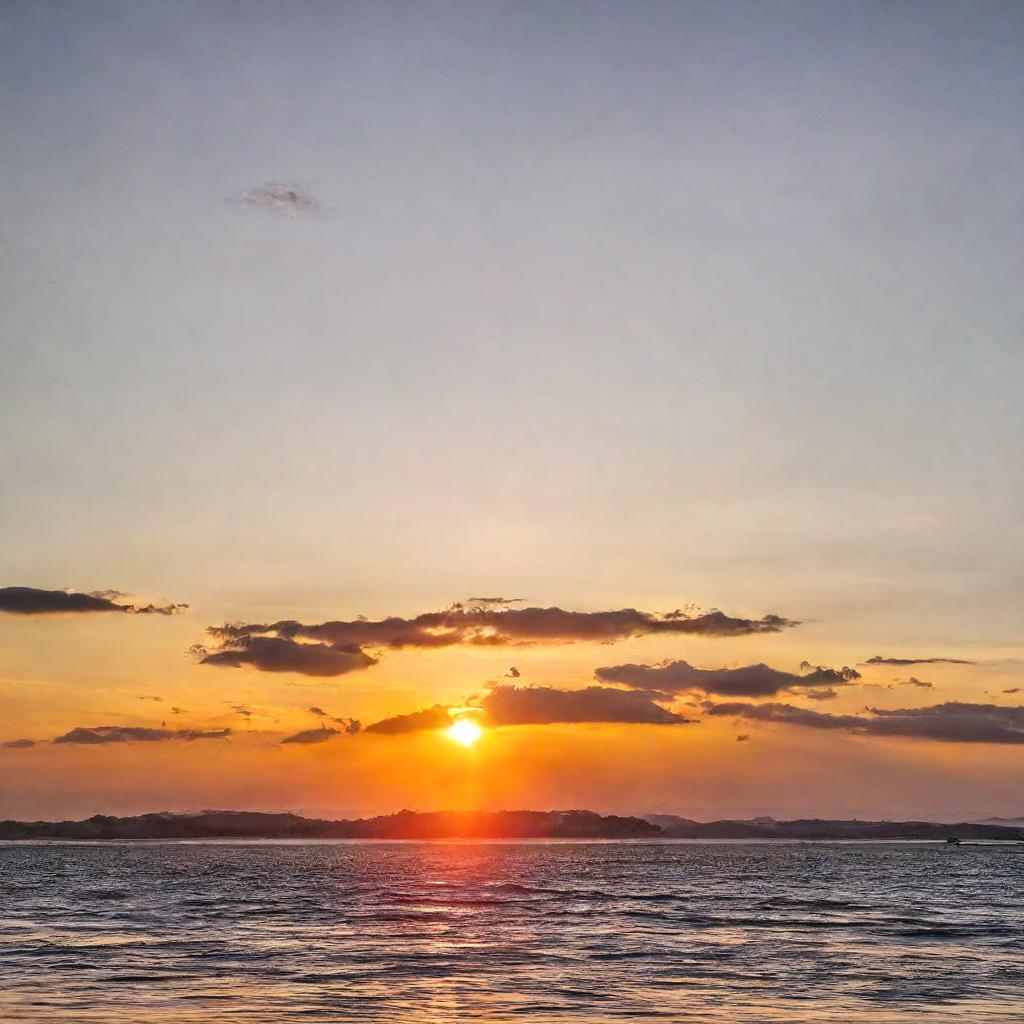
In each of these examples, the seed parameter influences the output of the Stable Diffusion model, resulting in different images from the same prompt.
Let us take an another example where we get to generate an image of a woman walking down the down the road wearing a hoodie. Let us add few more details to the prompts such that we get a image filled with more details.
Prompt: photo portrait of a stunning woman wearing a hoodie and walking down the road , wet reflections , highly detailed studio Ghibli, acrylic palette.
- Seed 1: For the first seed setting we get a image with only the side profile visible. The tonality of the picture goes with the prompt specified consisting of wet reflections , with even the hoodie having a glossy look due to the specification of the acrylic palette.
- Seed 2: The image generated for this particular seed settings is different from one before , we get a full portrait image of a woman in a hoodie walking down the street . The composition of the image is also slightly symmetrical with the subject present in the center of the screen
- Seed 3: This image is different from the other images which were generated , the subject gets to present her side profile . However the depth of the subject varies from the first two images considerably .
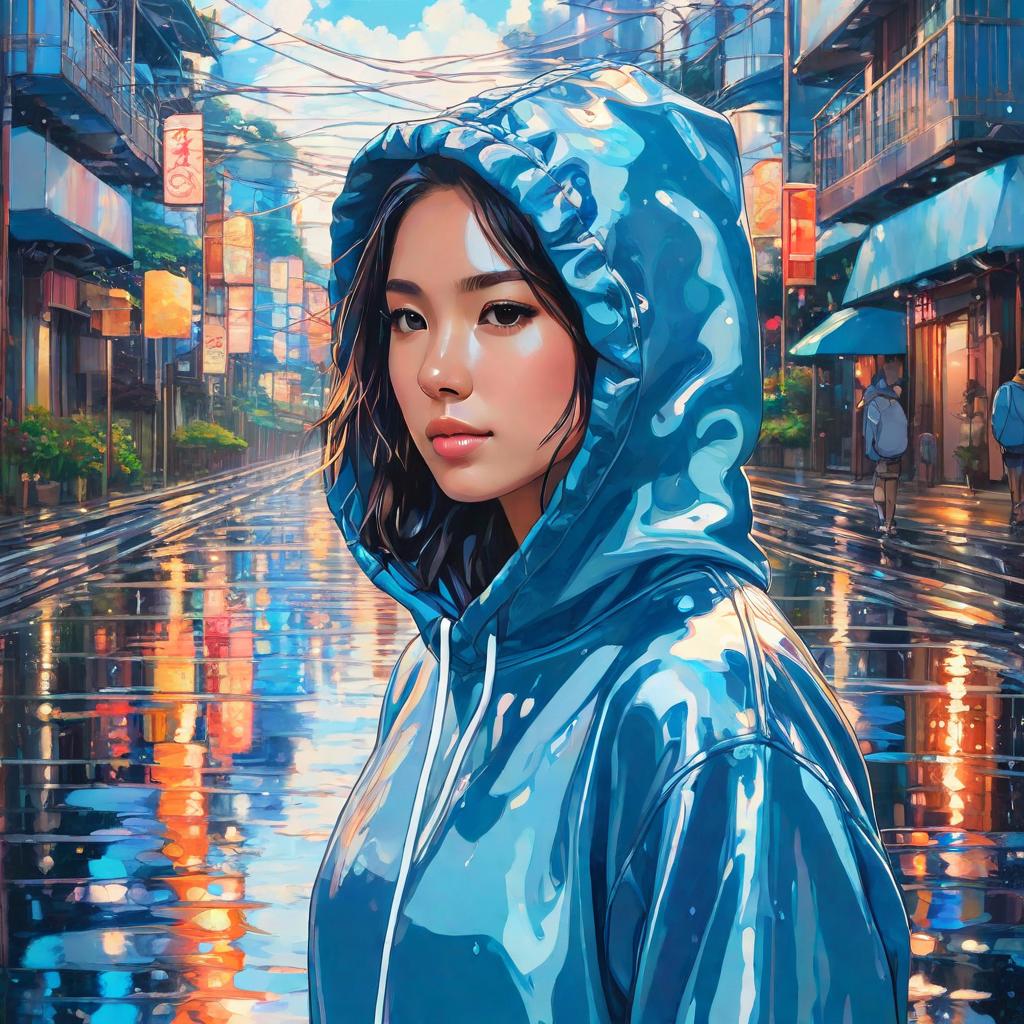
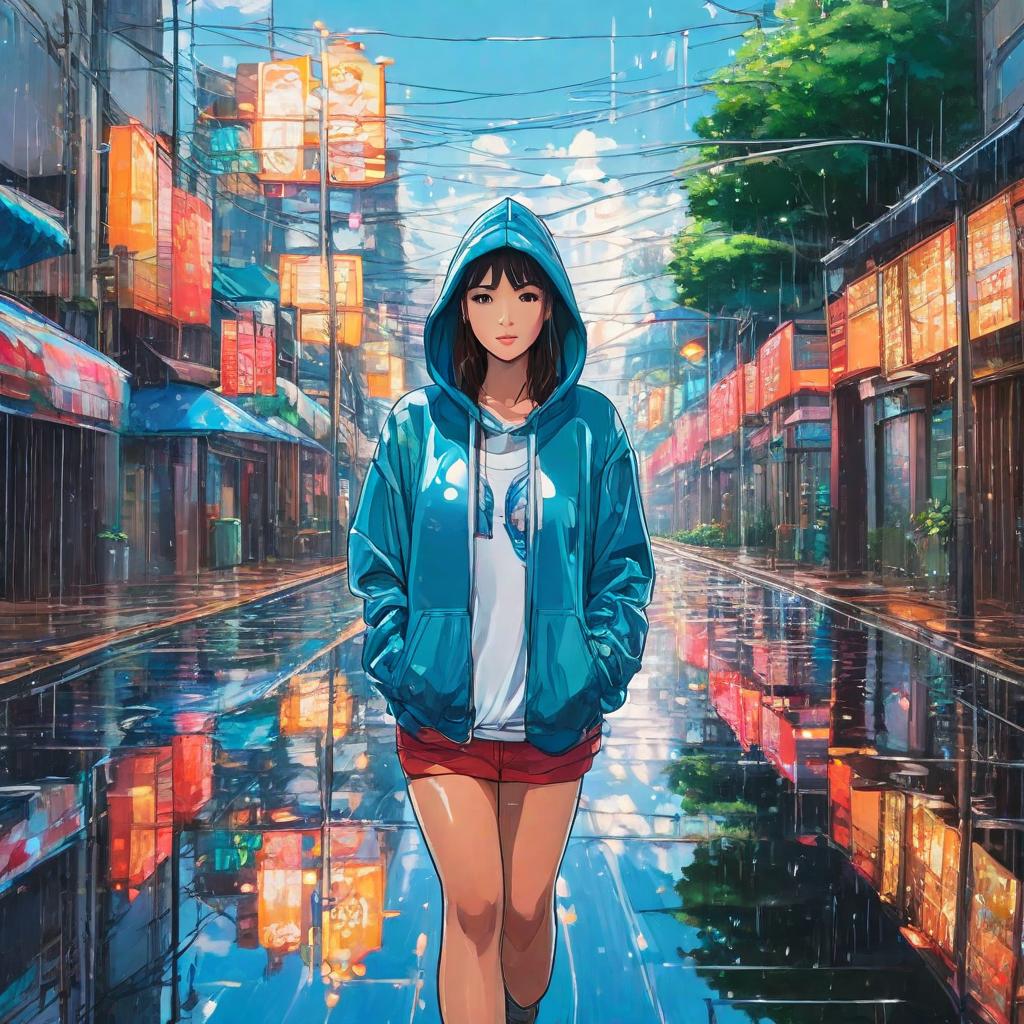
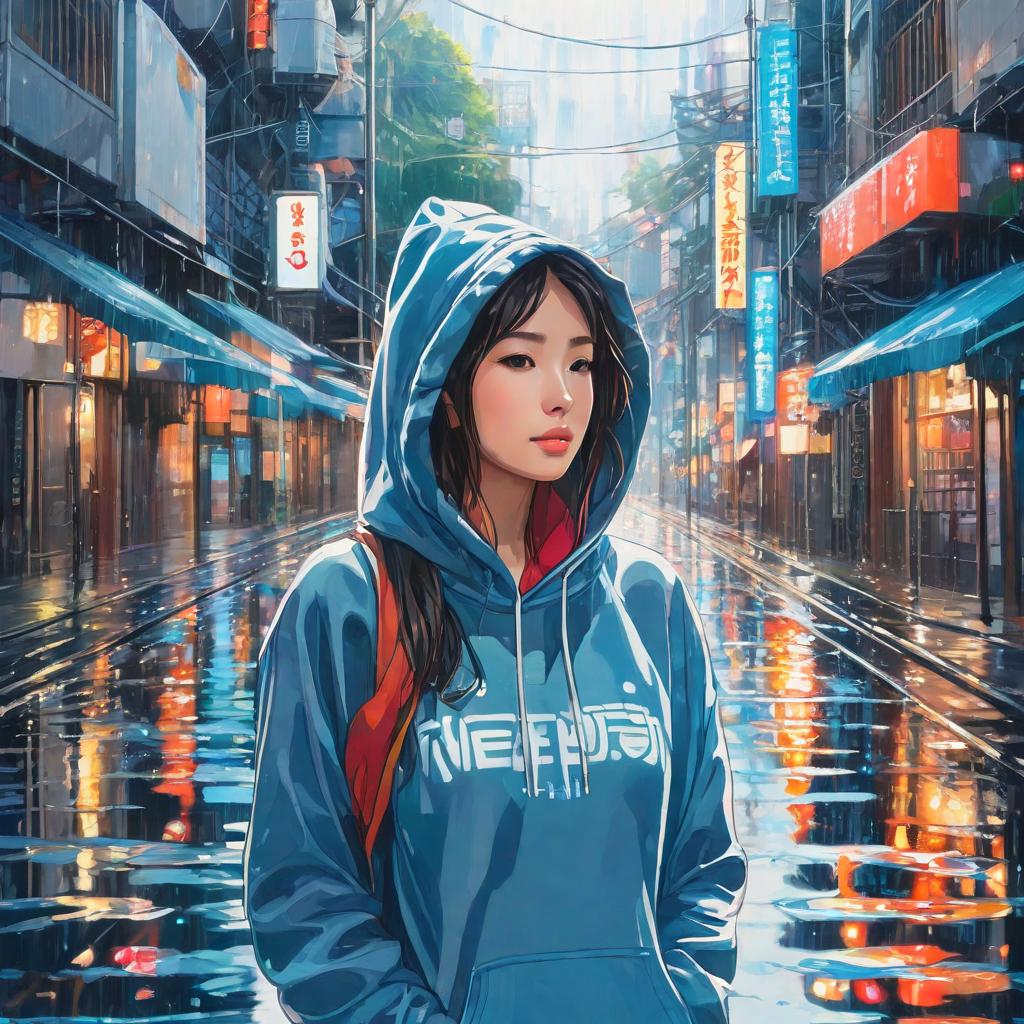
Let us take one more image of a handsome guy standing in snowy mountains , we can add more details to see the kind of effect it has in our final result
Prompt: Handsome man standing in snowy mountain with perfect lighting , shot on Arri alexa 35 in kodak potra 400 film
- Seed 1: The subject is well-dressed for the chilly conditions, wearing a tan-colored insulated coat complemented by a fur-lined hood that frames his styled dark hair.
- Seed 2: This image settings leads to a more close up frame for the subject present in the image , where get to take a much more closer look at the model . n contrast, the previous image only provided a glimpse of the subject up to their waist.
- Seed 3: With this third image setting we get to see the subject in a different angle from the first two images which were generated. We get to see the subject composed differently and also the lighting in the image is different compared to the rest.
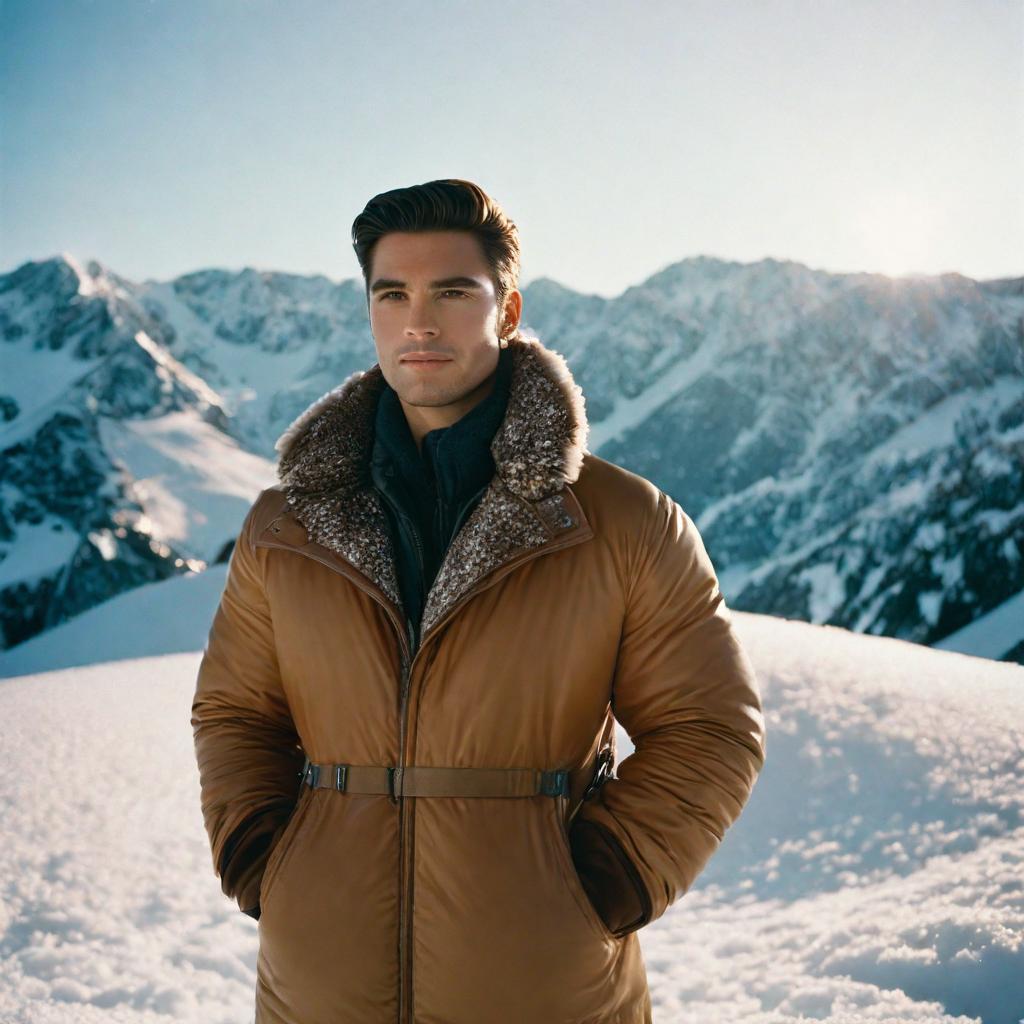
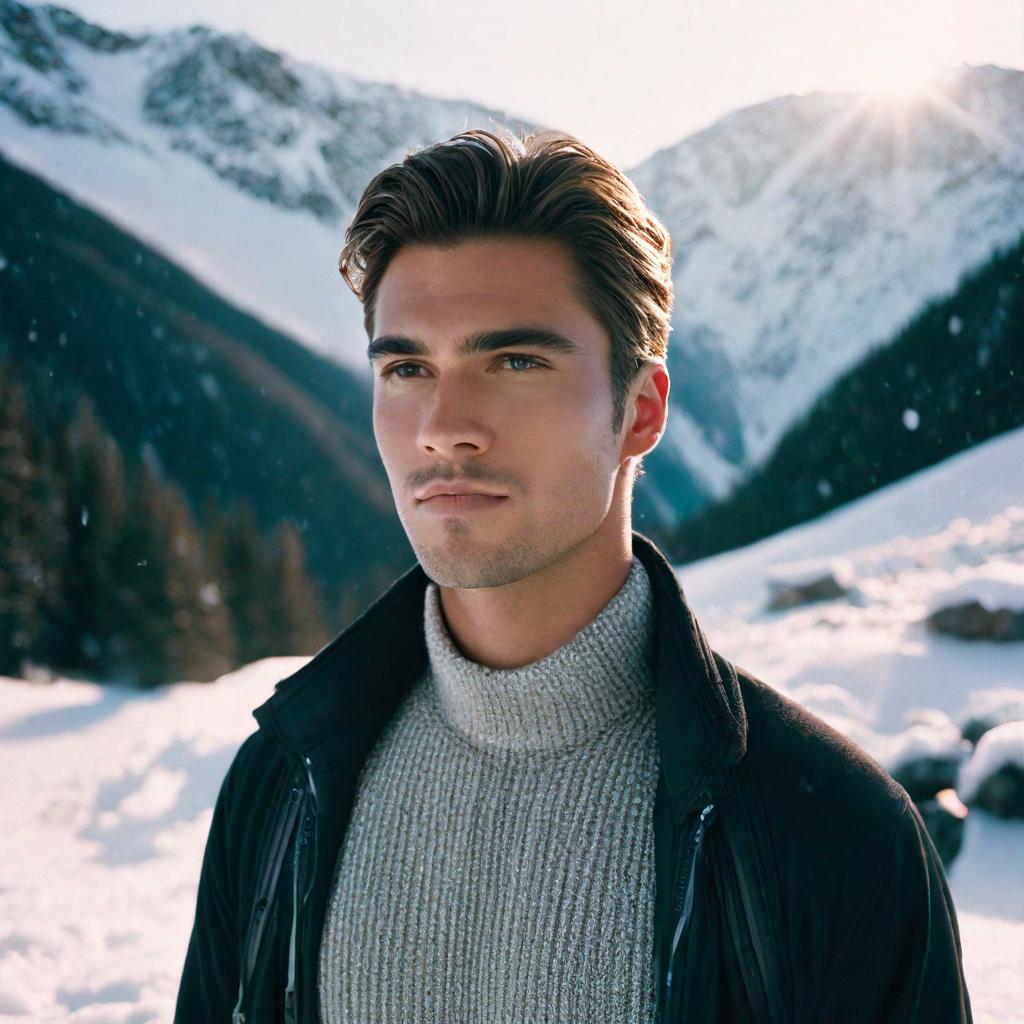
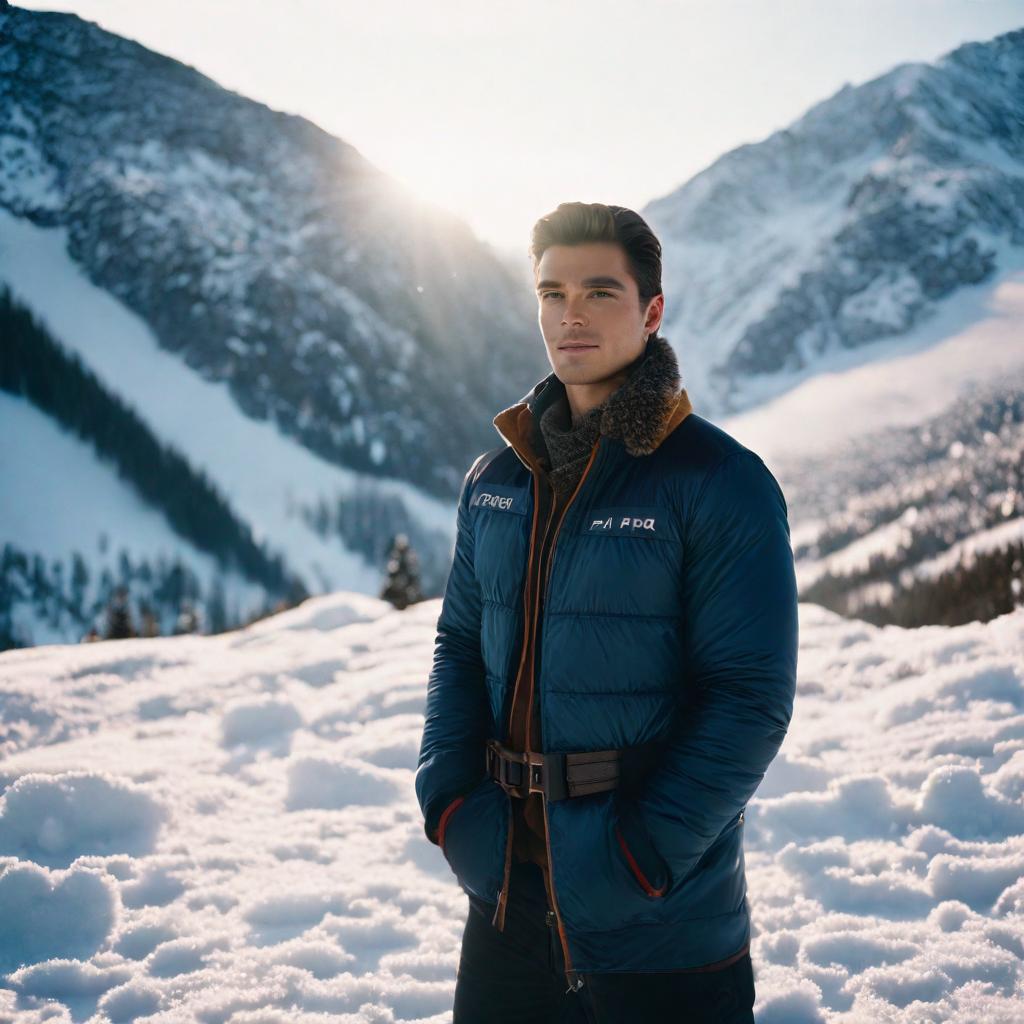
Conclusion:
Thus in this blog we got to take a comprehensive look at the usage of the seed parameter in the generation of images. Managing the seed parameter plays an important role in the generation workflow. After generating a few images and identifying a favorable composition and structure, freezing the seed while refining the prompt allows for altering the image while maintaining the same composition.. This helps in changing the picture while keeping the composition same. Also the seed parameter helps in reproducing the same image when used with the same parameters and prompt.