Fine-tune Your Own Flux.1 LoRA Models
Learn how to fine-tune Flux.1 on Segmind to generate stunning, tailored images for your specific needs. Create your own flux.1 LoRA models in just a few clicks.
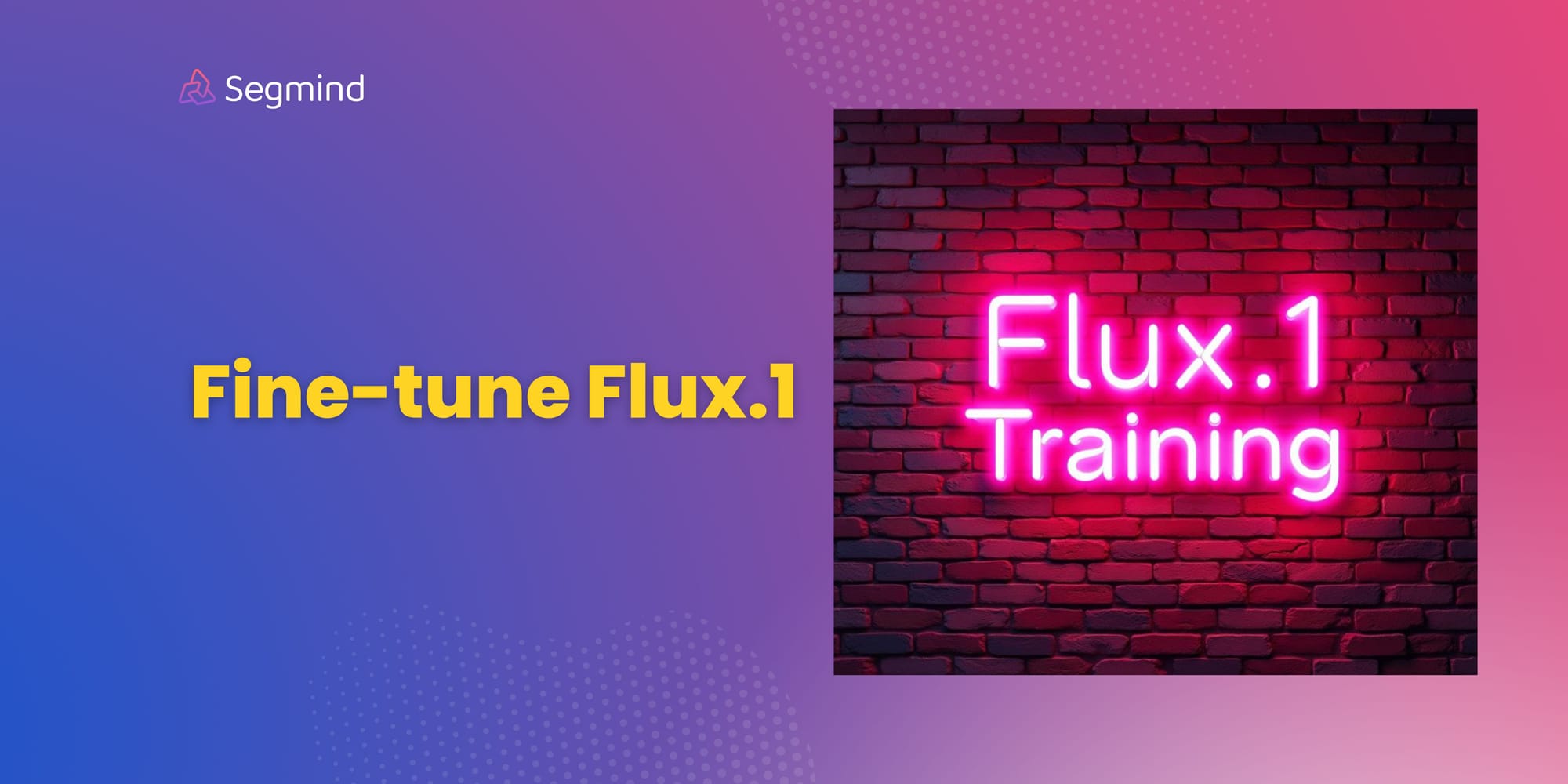
Flux.1 is a suite of text-to-image models, based on novel transformer architecture, developed by Black Forest Labs. These models redefine the state-of-the-art in image detail, prompt adherence, style diversity, and scene complexity for text-to-image synthesis.
To adapt Flux.1 to specific domains or artistic styles, fine-tuning is necessary. This process involves training the model on a custom dataset to align its output with desired characteristics.
Segmind provides a user-friendly web interface for fine-tuning Flux.1. You can create your own Flux.1 LoRA models for a wide range of applications in just a few clicks.
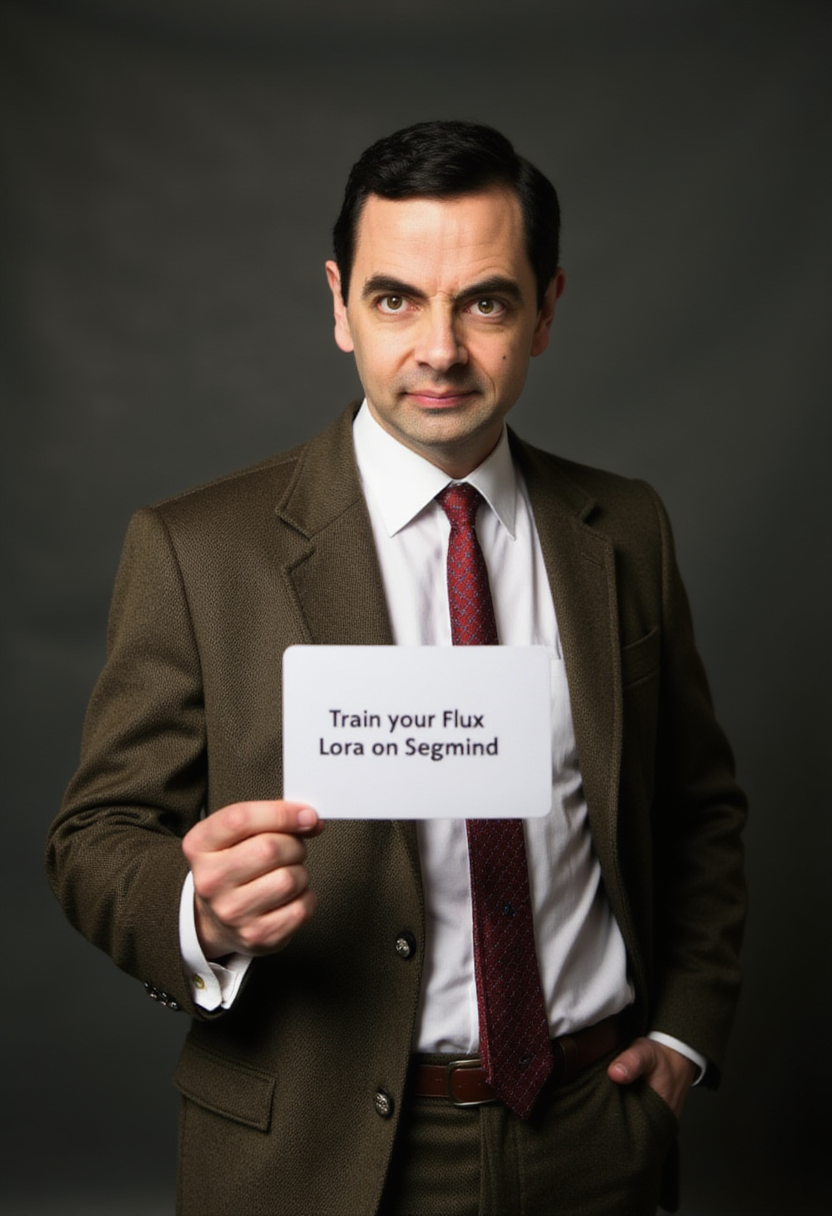
Why fine-tune Flux.1?
Fine-tuning is the process of customizing a pre-trained AI model to generate images that align with specific styles, concepts, or objects. By training the model on a custom dataset, you can enhance its ability to create images that closely match your desired prompts and attributes.
By introducing a dataset of images and corresponding captions, we can refine Flux.1's understanding of concepts. The model adjusts its internal parameters to better correlate specific textual descriptions with visual representations. This process is akin to teaching an artist to paint in a particular style.
Compared to training a model from scratch, fine-tuning consumes fewer computational resources and time. This translates to faster results and lower costs, making it an efficient approach to customizing image generation.
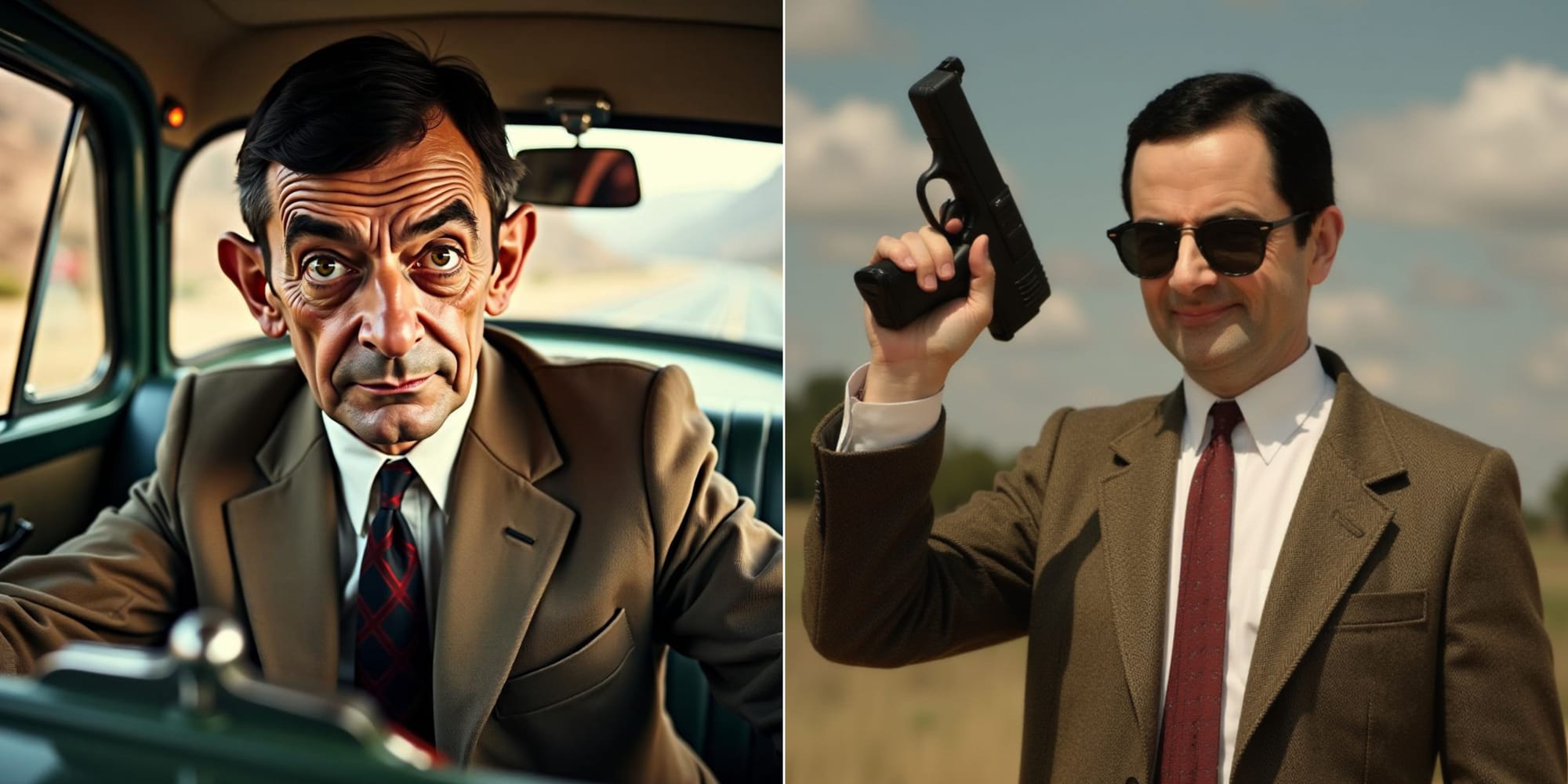
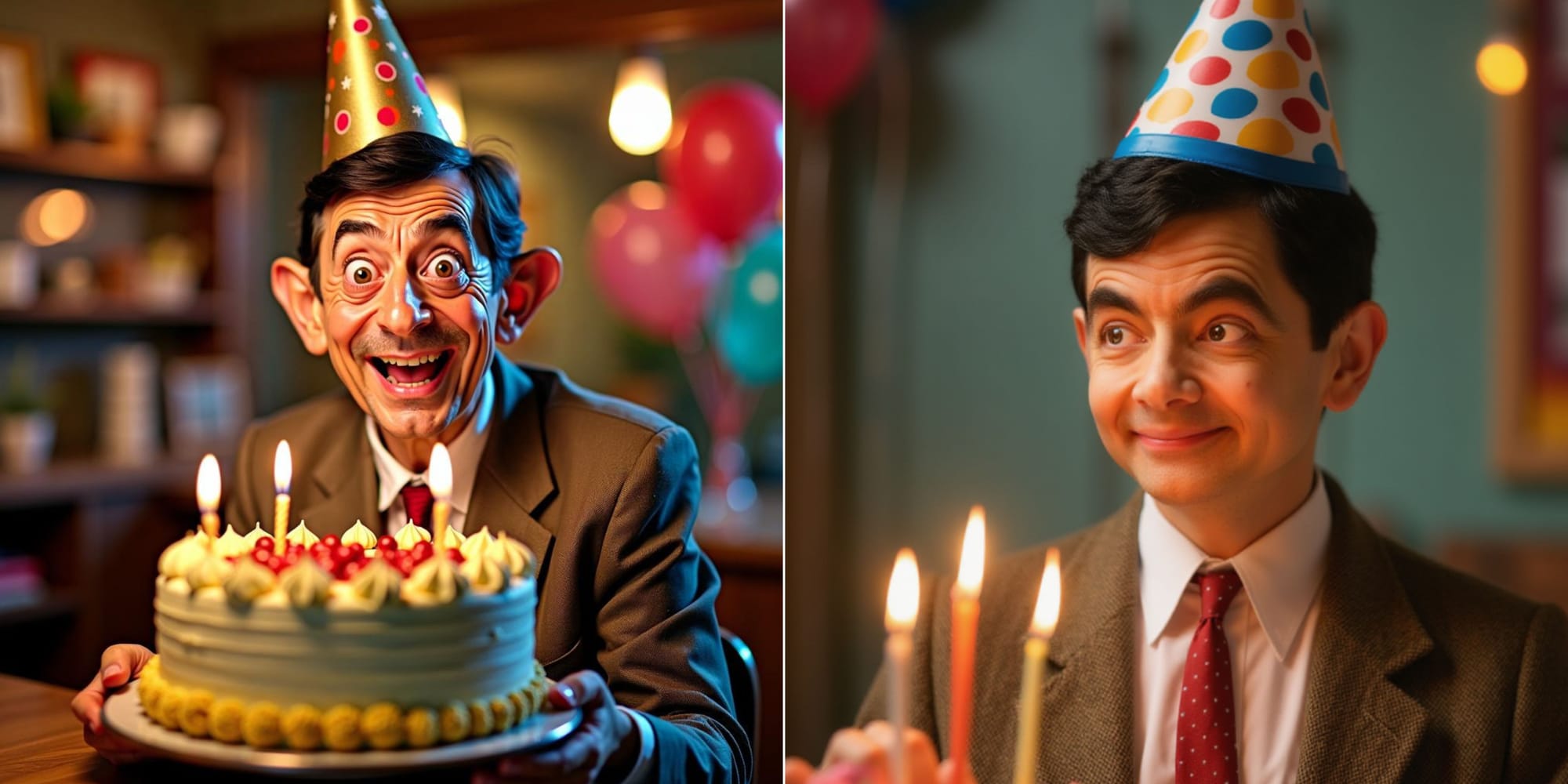
How to fine-tune Flux.1 on Segmind?
Segmind offers a user-friendly platform to fine-tune Flux.1 with just a few clicks. Go to model training on your dashboard. Choose Flux.1 training.
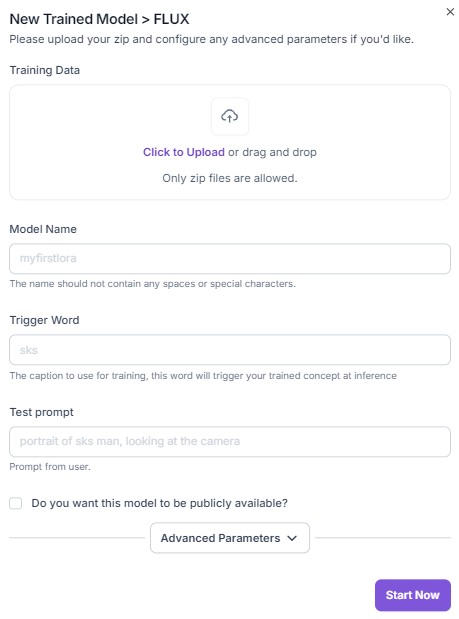
Preparing Your Dataset
- Image Quality: Use high-resolution JPEG or PNG images.
- Quantity: Aim for 10-15 images for optimal results.
- Diversity: Include variations of your subject to enhance model understanding.
- Captions: Segmind automatically generates captions for your images. No need to create captions for images in your training data.
Training Your Model
- Upload Training Dataset: Compress your images into a ZIP file and upload.
- Model Details:
- Model Name: Choose a descriptive name for your fine-tuned model.
- Trigger Word: Select a unique word to activate your concept. The trigger word serves as a conditional activation token within the fine-tuned model. It functions as a specific input that prompts the model to access and apply the learned concept embedding. By incorporating the trigger word into a text prompt, you can explicitly direct the model to generate outputs aligned with the custom dataset. For instance, if your subject is a majestic tiger, you could choose a trigger word like "tgr." When the prompt "photo of tgr in the jungle" is provided to the fine-tuned model, the model will process the "tgr" token as a conditional input, activating the learned concept embedding for a tiger. This will influence the image generation process, increasing the likelihood of producing an image featuring a tiger within a jungle environment.
- Test Prompt: This will appear in the model preview on the fine-tuned model playground on Segmind.
- You have the option to set your model's privacy to public or private. Making your model public allows other Segmind users to utilize it for inference.
- Training Parameters
We recommend to start with default settings for best results. But feel free to experiment with parameters like learning rate, batch size etc.
Using Your Trained Model
Once training is complete, you can use your fine-tuned FLUX.1 model to generate images. You can download your model weights or alternatively inference on Segmind. Simply include the trigger word in your prompt to activate your custom concept. Example: Trigger Word: "mychar", Prompt: "mychar as a superhero, digital art".

Understanding Training Hyper Parameters
Training parameters significantly influence the outcome of a fine-tuned model. By understanding these parameters, you can make informed decisions to optimize your fine-tuning process and achieve desired outcomes. Here's a breakdown of key parameters:
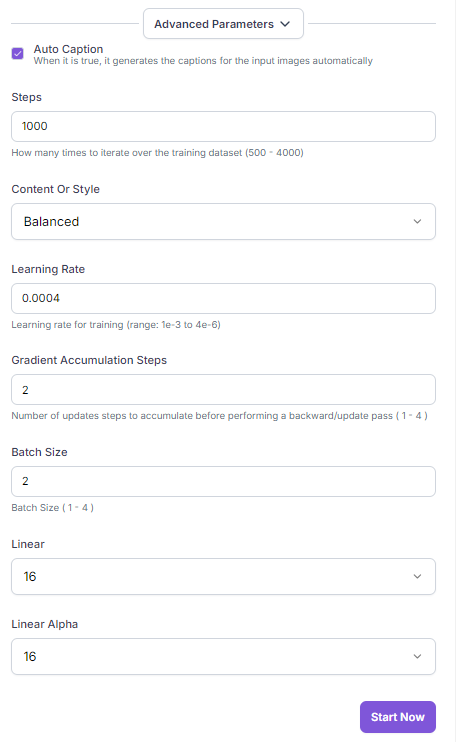
- Steps: The number of training iterations. More steps generally improve model performance but increase training time.
- Learning Rate: Controls the step size during optimization. A lower rate ensures stability but can slow down training, while a higher rate risks overshooting the optimal solution.
- Grad Accumulation Steps: Processes multiple mini-batches before updating model weights, effectively increasing batch size without extra memory.
- Batch Size: The number of samples processed simultaneously. Larger batch sizes can stabilize training but require more computational resources.
- Linear: This refers to Linear adaptation, which is a basic technique for adapting a pre-trained model to a specific task. It involves using low-rank matrices to efficiently update the model's parameters.
- Linear-Alpha: Linear-Alpha adaptation extends the linear approach by introducing a scaling parameter, alpha. This parameter offers greater control over the impact of the adaptation on the original model. By adjusting alpha, you can fine-tune the balance between preserving the original model's knowledge and incorporating the new information from the adaptation dataset.
- You can also choose the training focus: content, style, or balanced. Content prioritizes preserving the image's details and structure, while style focuses on replicating and adapting visual aesthetics and artistic elements. We recommend using the default balanced setting. Balanced combines both content and style considerations for versatile image generation.
Pricing
Check fine tuning pricing here.
Need help? Join our Discord channel for immediate support.