Exploring the Current Limitations of OpenAI’s Inpainting Capabilities
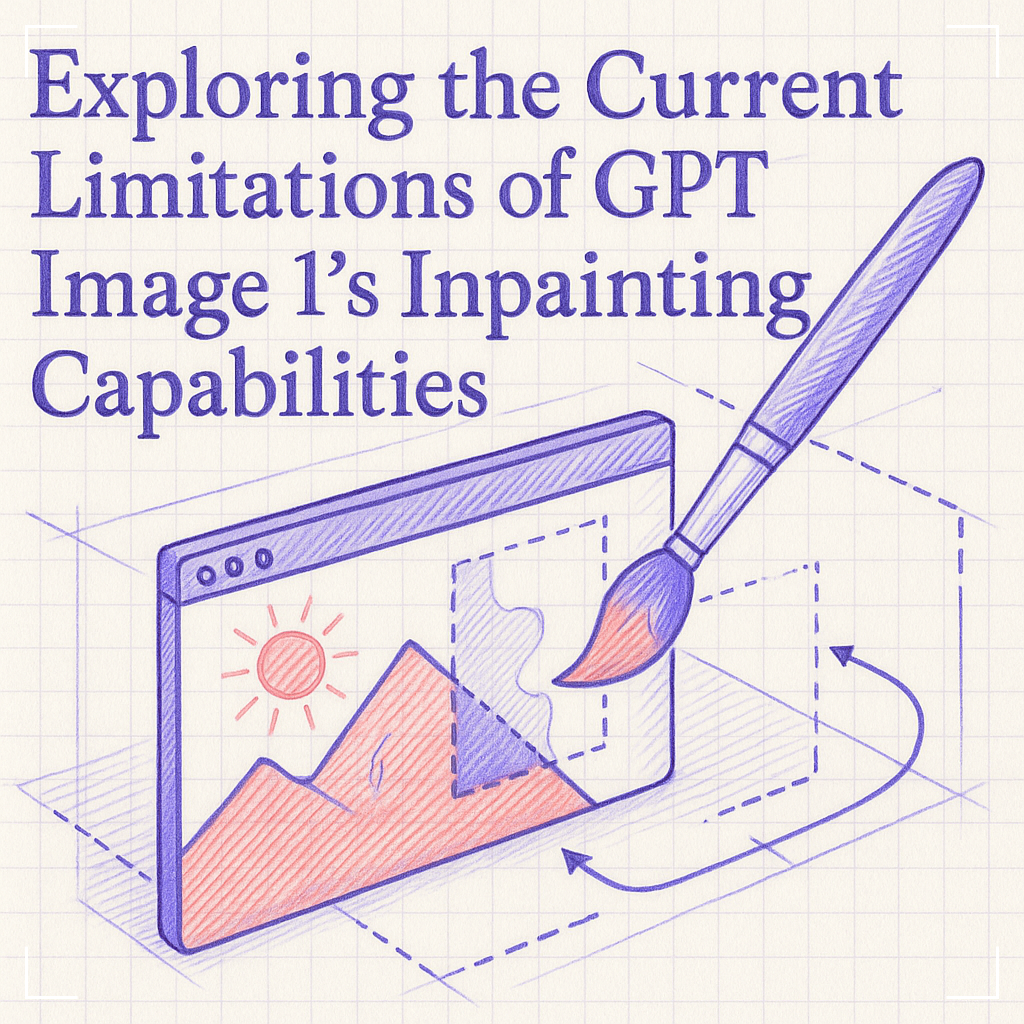
As generative AI technology continues to evolve, new capabilities like image editing, enhancement, and inpainting are opening doors for creative and professional workflows. However, at Segmind, our ongoing evaluations have uncovered important limitations in OpenAI's current GPT Image 1's inpainting capabilities that users and developers should be aware of.
Through internal testing on GPT Image 1 model, we observed a few consistent patterns where the inpainting functionality, designed to seamlessly modify or fill parts of an image, struggled to deliver the level of precision or contextual understanding users might expect and need for their workflows.
1. Low Fidelity in Matching the Original Image
One of the core promises of inpainting is the ability to modify a specific section of an image while completely preserving the style, structure, and realism of the surrounding areas. In practice, however, our tests found that inpainted regions often diverge significantly from the original image.
The GPT Image 1 model sometimes introduces subtle but noticeable inconsistencies in texture, shading, or even object structure, leading to a final image where the edited area looks "pasted" rather than organically blended. This can be especially problematic for use cases like product photography edits, portrait touch-ups, or any application where seamlessness is critical.
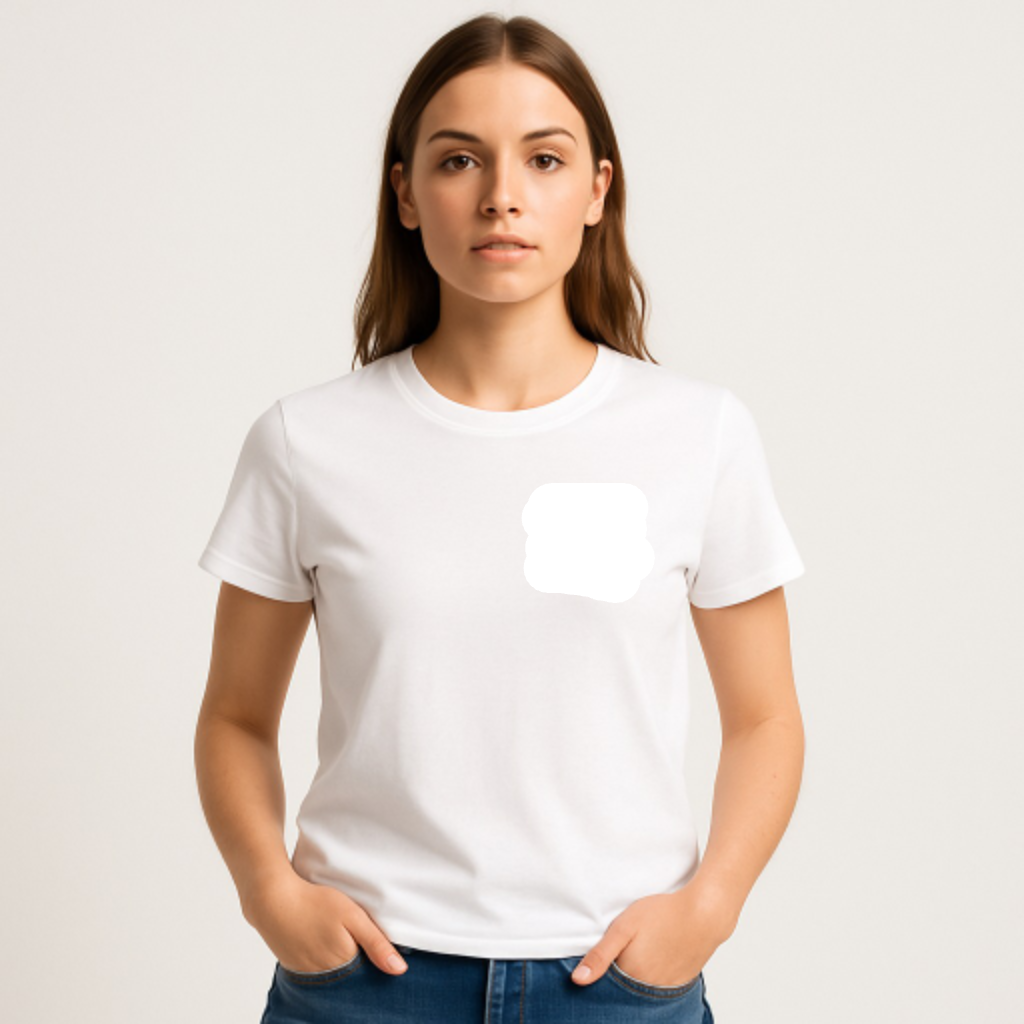
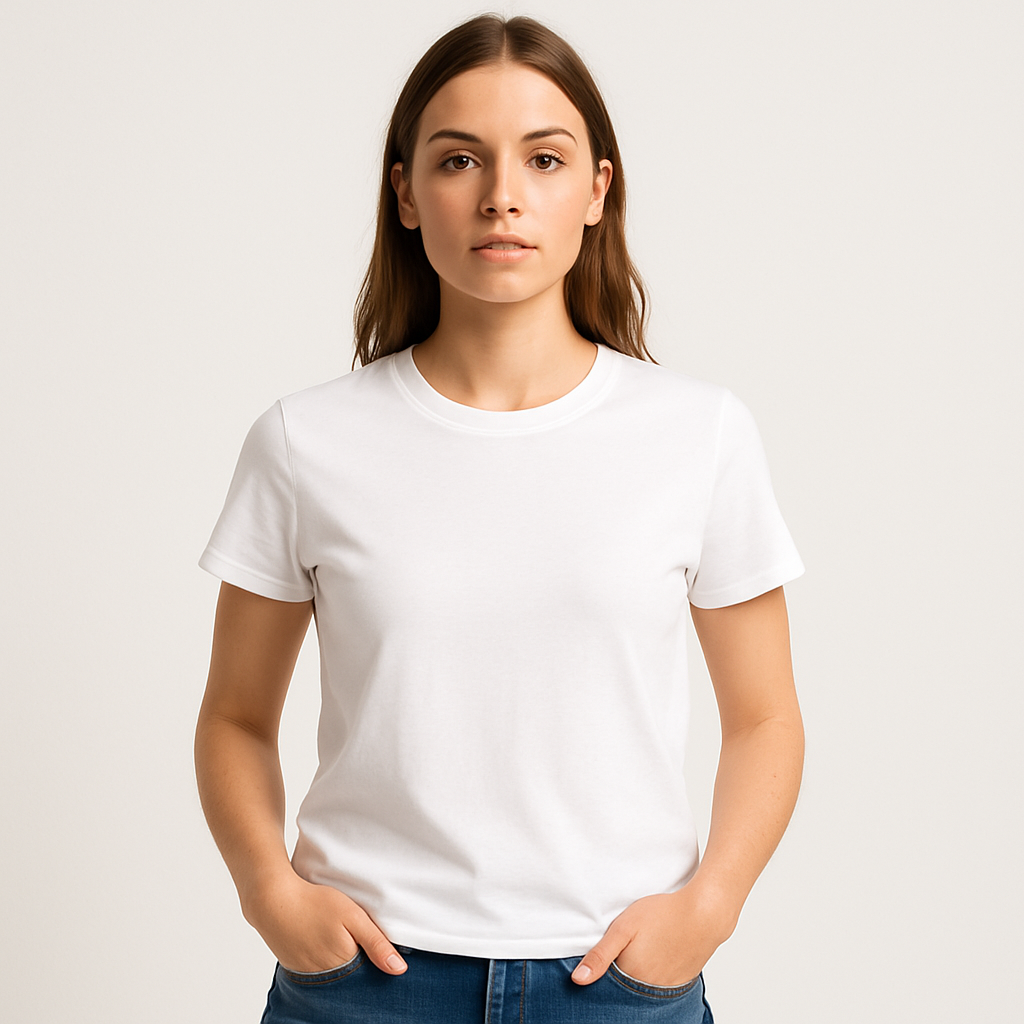
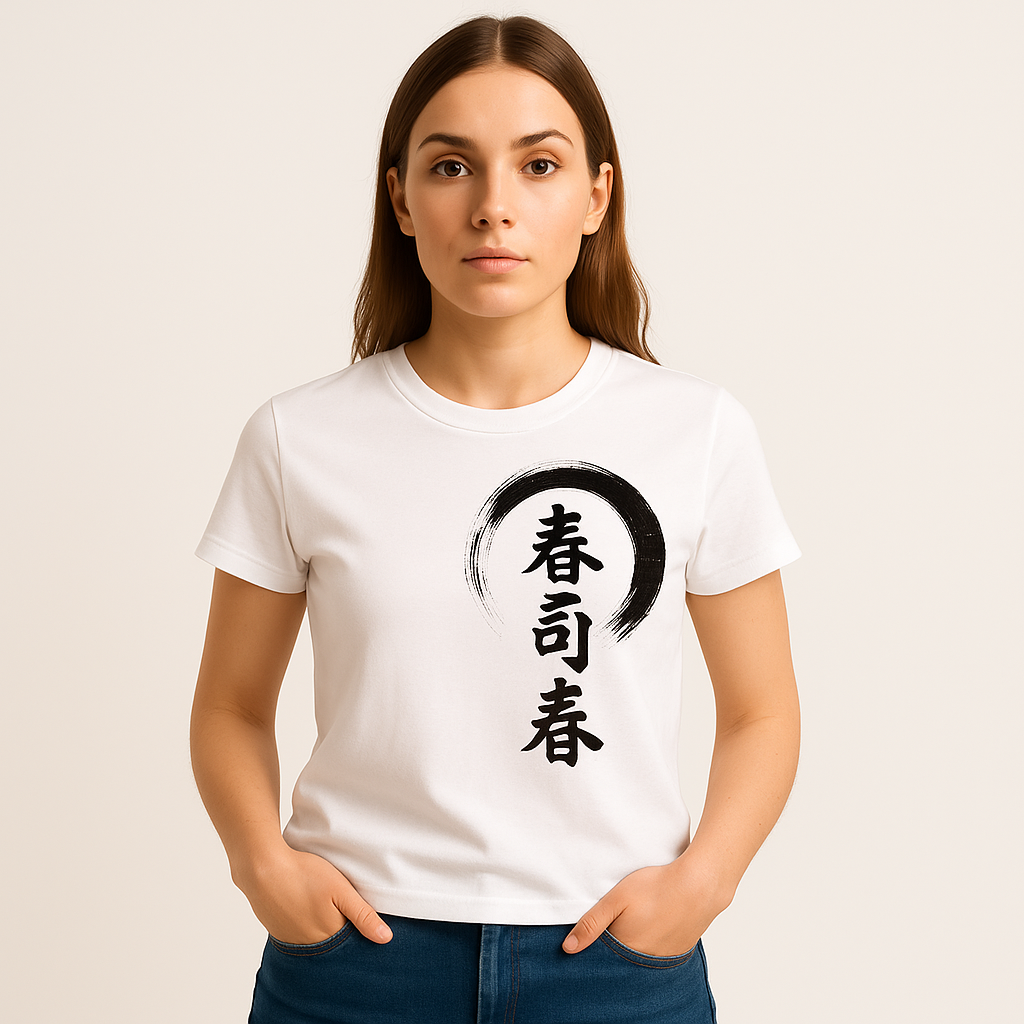
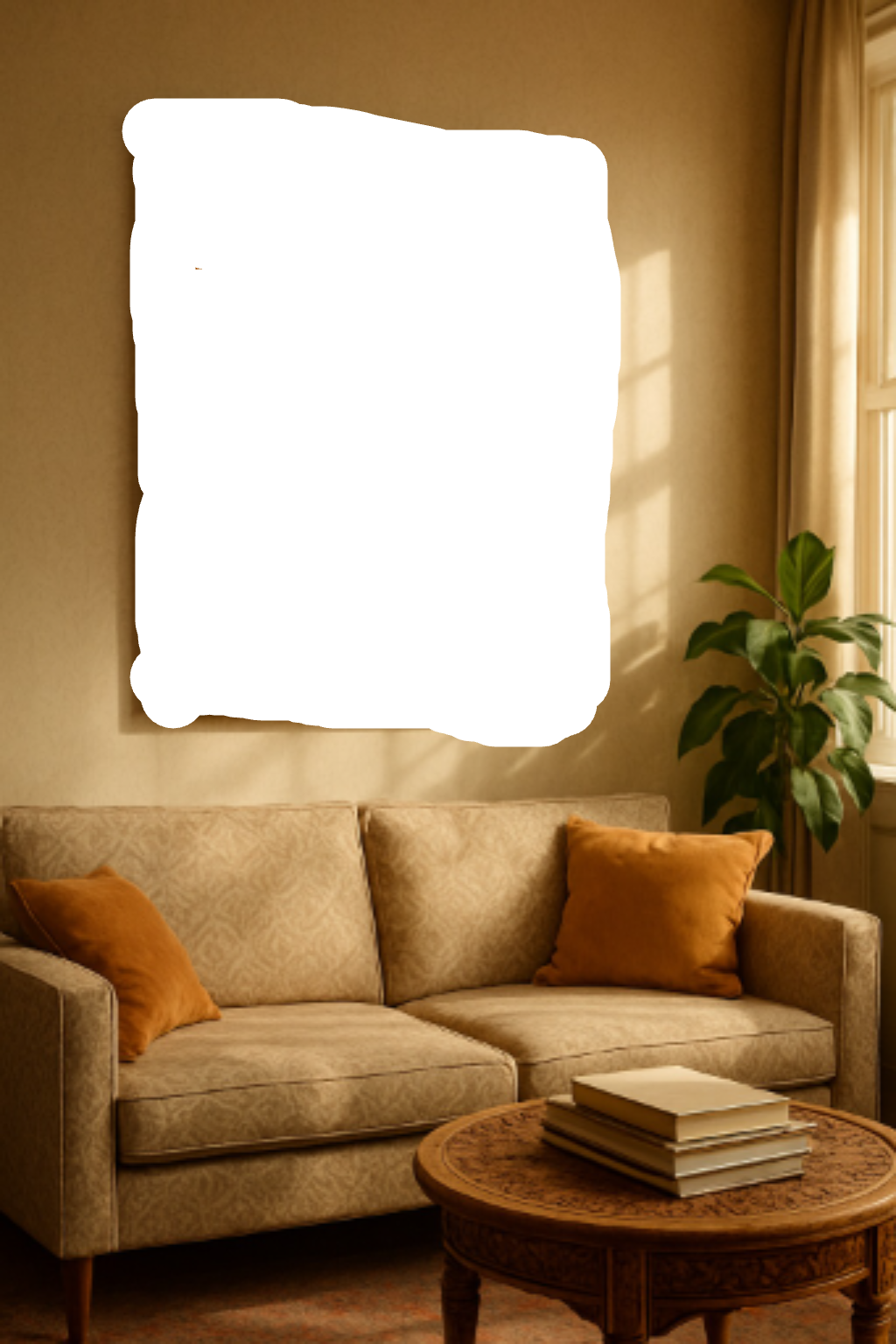
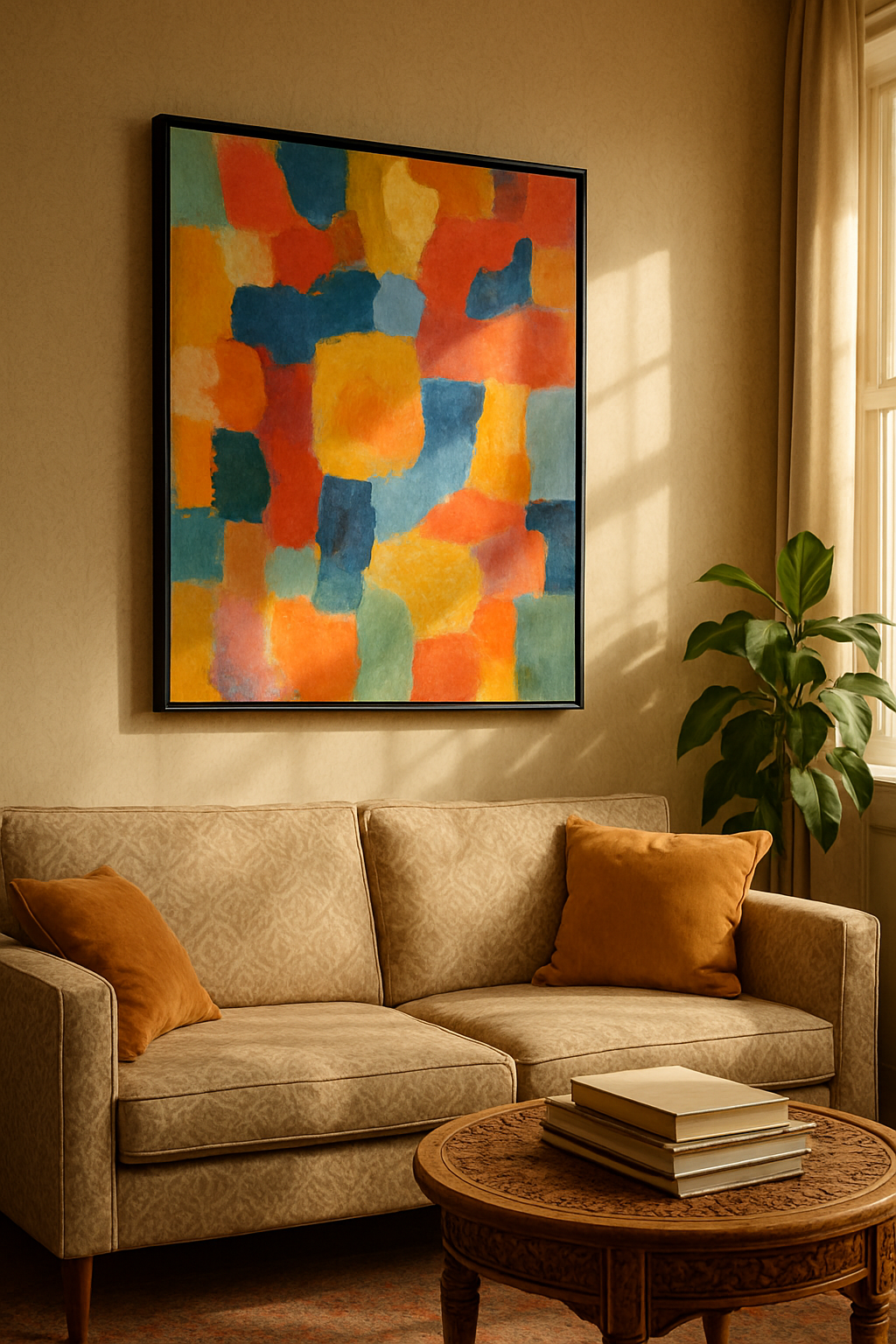
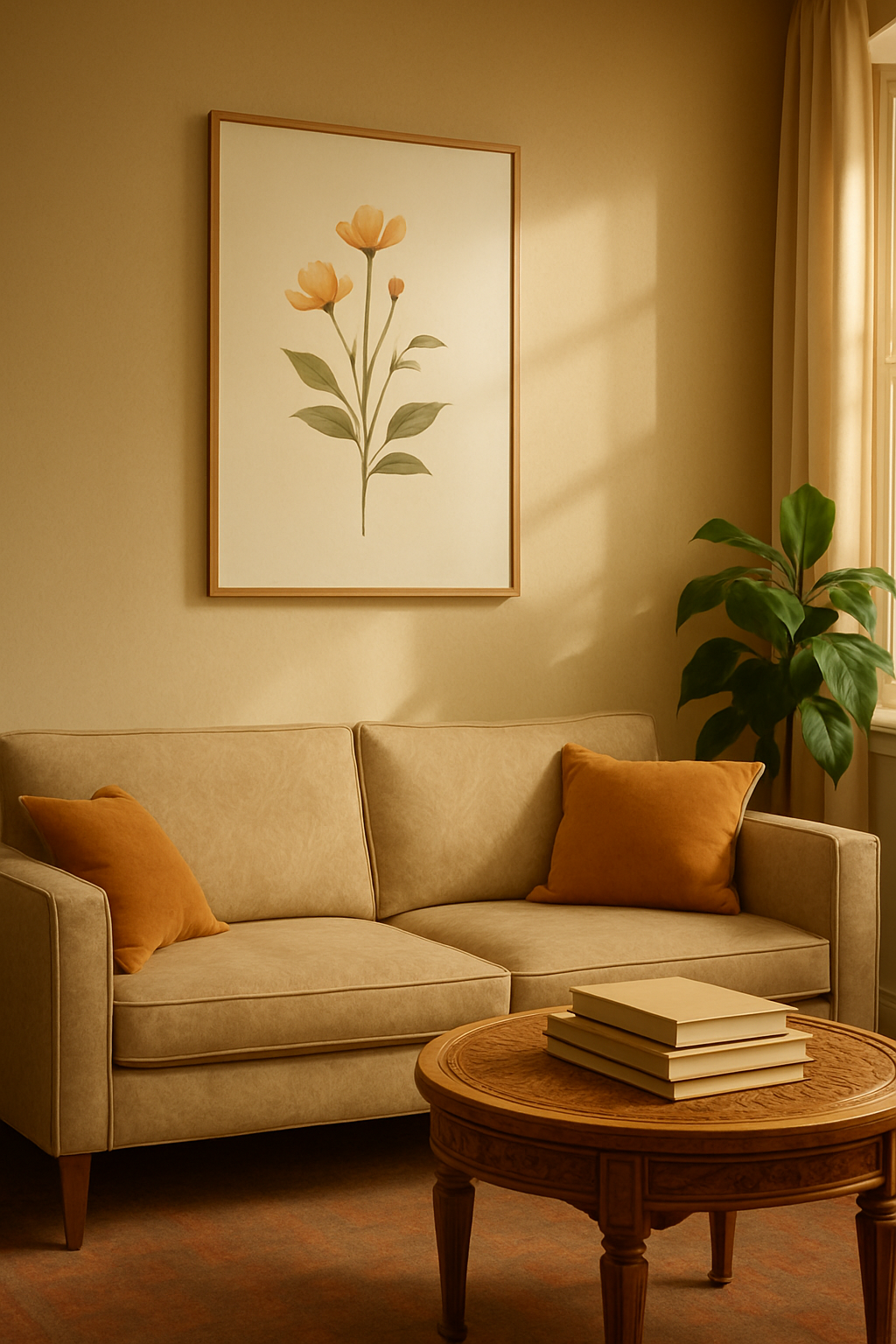
2. Struggles with Fine, Precise Edits
When tasked with minor or highly detailed adjustments, such as slightly changing an object's color, modifying fine accessories (like jewellery), or replacing a small background object, the model frequently overcompensates. Instead of making a minimal change, it tends to reinterpret or even replace surrounding elements, resulting in larger-than-intended modifications.
This behavior suggests that the current version of OpenAI's inpainting model may not yet have fine-grained spatial control at the pixel or object level, which is crucial for industries like design, advertising, and media production where micro-adjustments are routine.
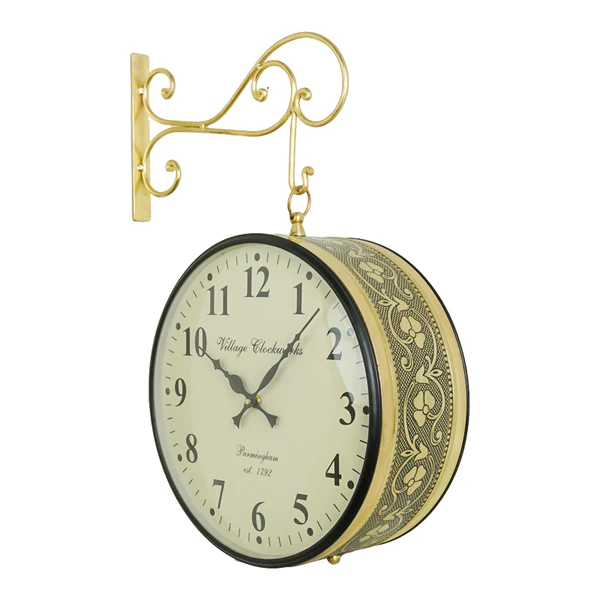
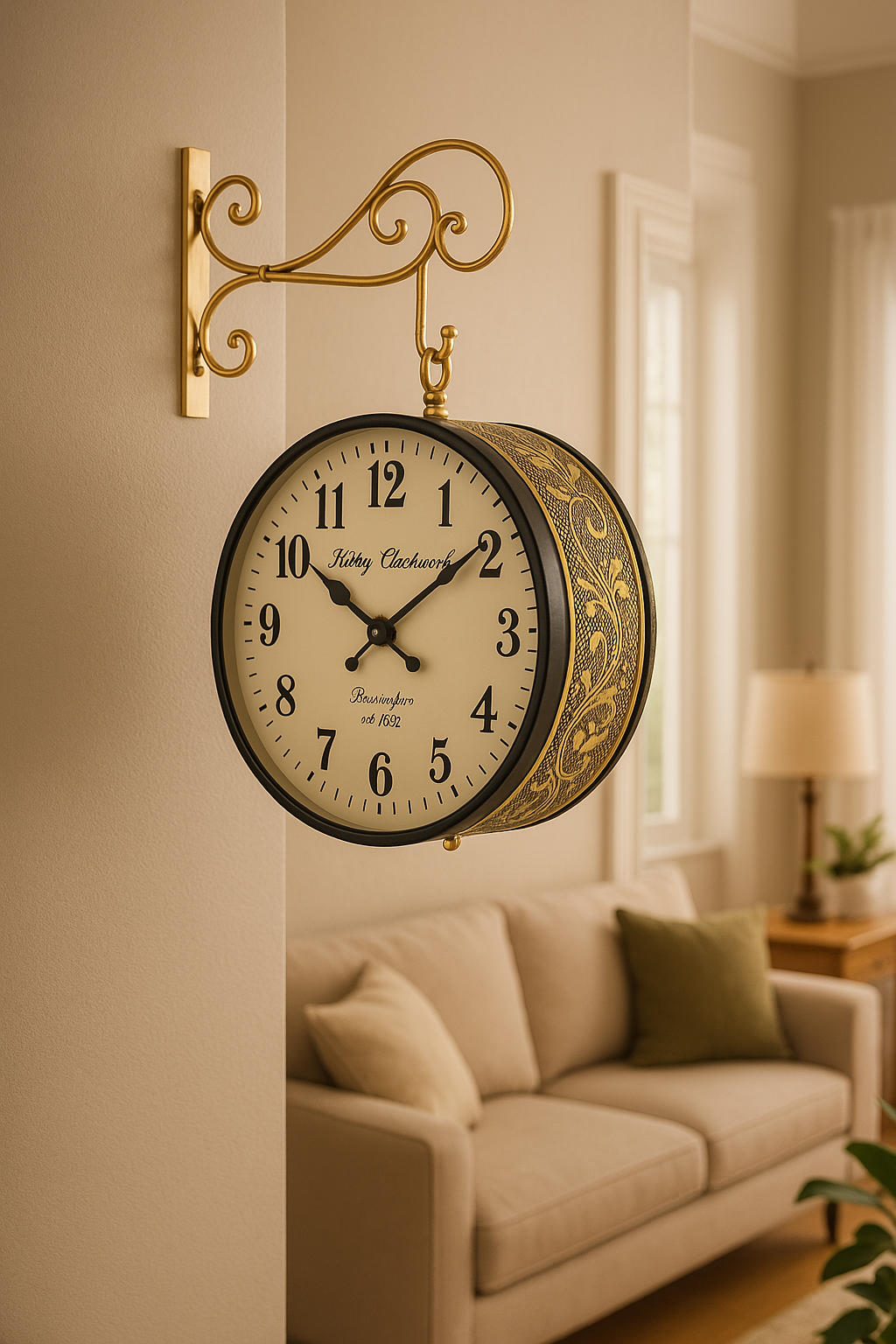
Prompt: Can you create a beautiful image of this clock in a home. I would like to use this image for creating online advertising campaigns.
3. Contextual Misunderstandings in Edits
Another limitation we observed was the model’s difficulty in maintaining contextual coherence when editing a portion of an image. For example, asking the model to modify a character’s clothing could inadvertently lead to changes in the background, lighting, or pose and vice-versa. Even though the prompt was narrowly focused on just one aspect, it tends to change the other.
This suggests that the model may be using broader heuristics based on the entire image when deciding how to inpaint, rather than truly isolating the user's intended region. As a result, small, specific edits can sometimes unintentionally trigger large contextual shifts.
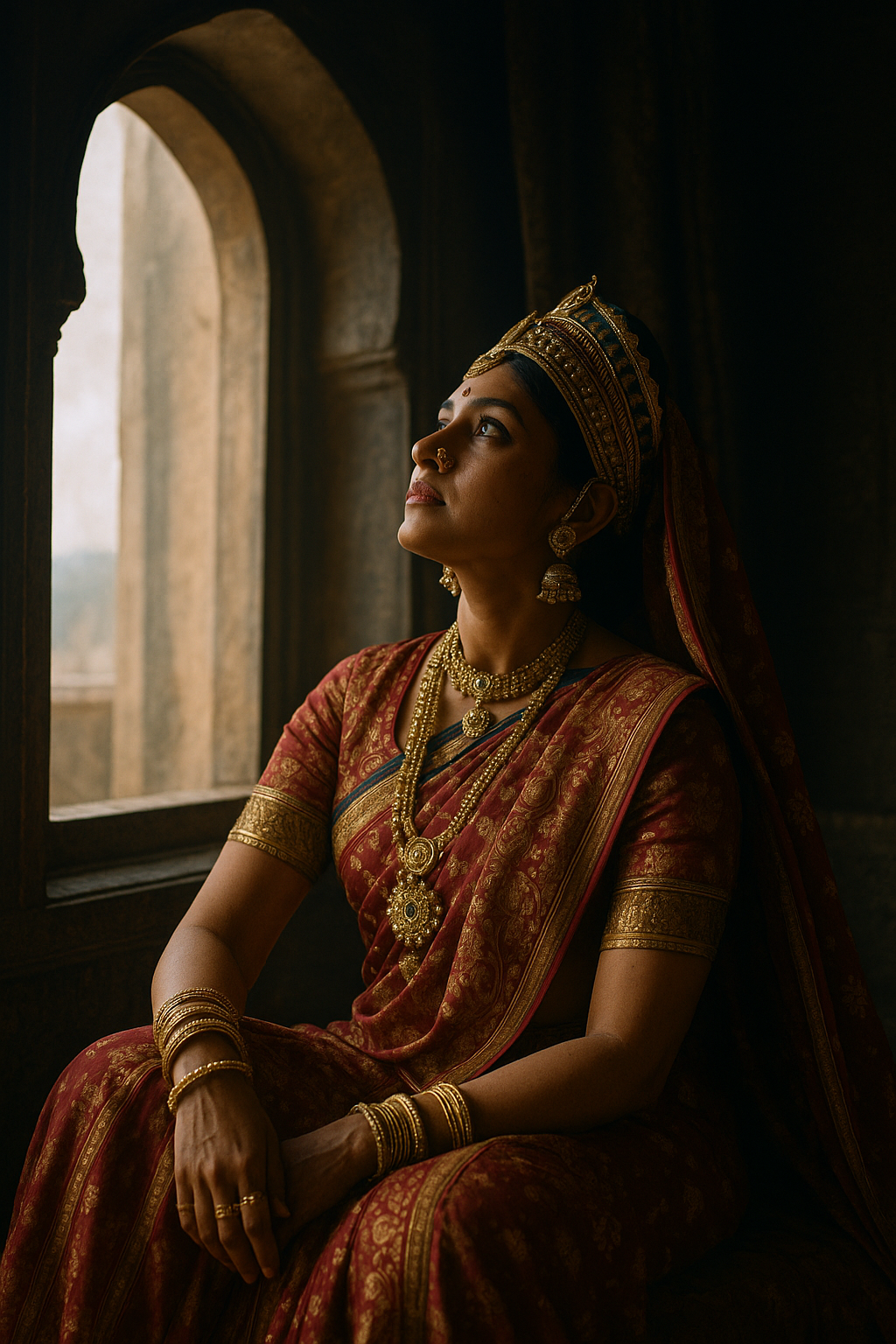
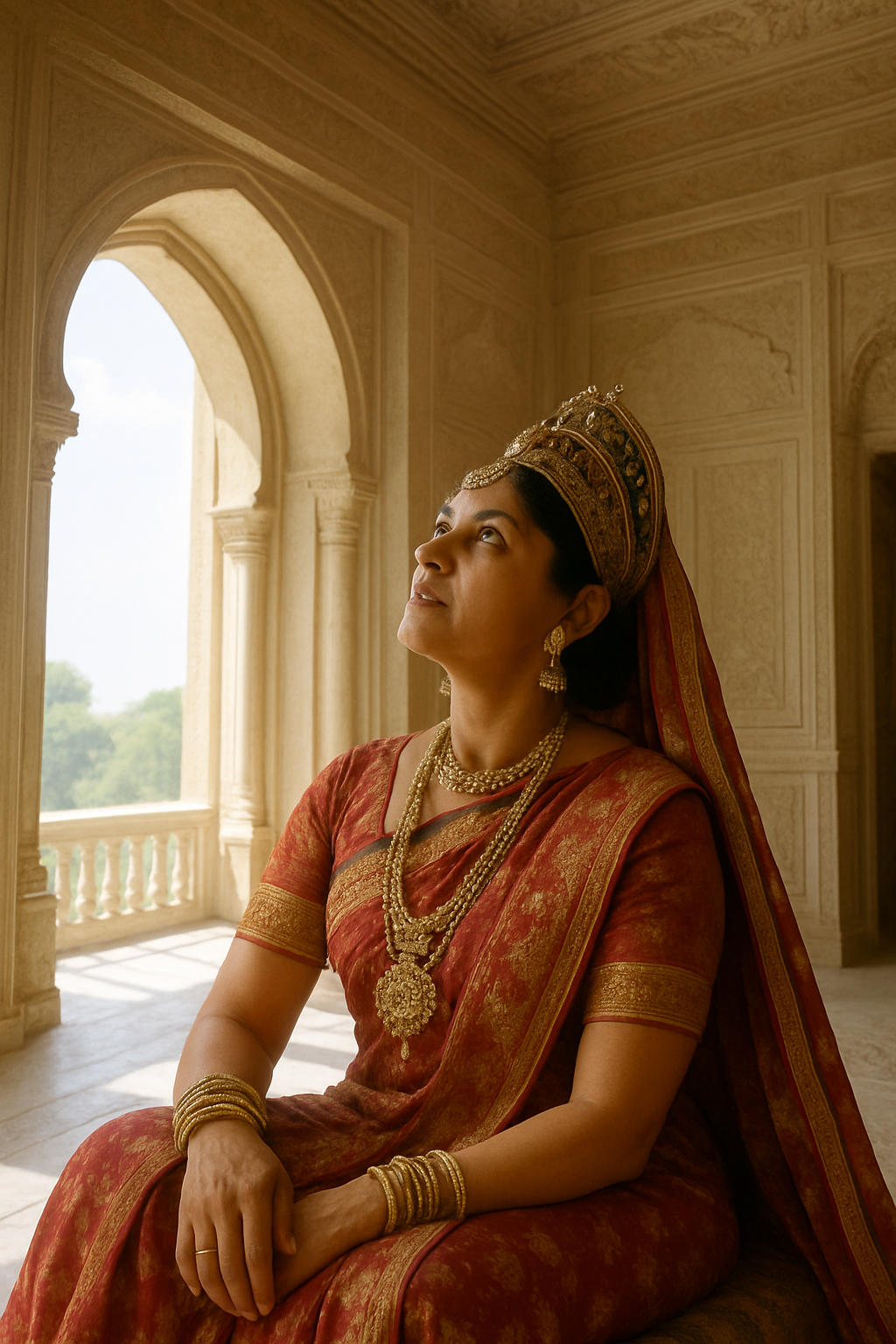
Prompt: Make the lighting brighter and make her room look much larger than what it is now.
4. Handling of Text Within Images Remains Challenging
Despite broader advances in image generation, text handling inside inpainted areas remains a pain point. When trying to edit images containing text, such as changing a signboard or modifying product packaging, the model often generates inaccurate, incomplete, or distorted text, even when provided with clear instructions.
This limitation is particularly critical for brand marketers, e-commerce companies, and content creators who rely on accurate text representation within visual assets.
Where OpenAI’s Focus May Be Headed
To be clear, these findings don’t diminish the impressive strides OpenAI has made in expanding multimodal capabilities. Inpainting itself represents a complex intersection of image understanding, prompt following, and generative consistency , and solving these challenges at scale is non-trivial.
Based on the observed limitations, we believe future improvements from OpenAI might focus on:
- Enhancing fine-grained control: Giving users more precise adjustment tools or input parameters to constrain the model’s edits.
- Better contextual anchoring: Enabling inpainting to truly respect the unedited areas without large interpretational shifts.
- Improved text generation inside images: A known industry-wide challenge, but critical for professional workflows.
- Smarter masking assistance: Tools that help users define and refine edit masks easily, without needing pixel-level manual precision.
At Segmind, we are excited about the pace of innovation in the generative AI in media space — and believe that openly understanding the current strengths and gaps of these tools helps developers, creators, and businesses make smarter decisions about adoption and integration.
We’ll continue to share our research findings as the technology evolves.