Comparing SSD-1B Canny and ControlNet Canny SD 1.5
This blog post covers a detailed side-by-side comparison of the SSD1-B ControlNet Canny with SD 1.5 ControlNet Canny, aiming to dissect their capabilities and performance.
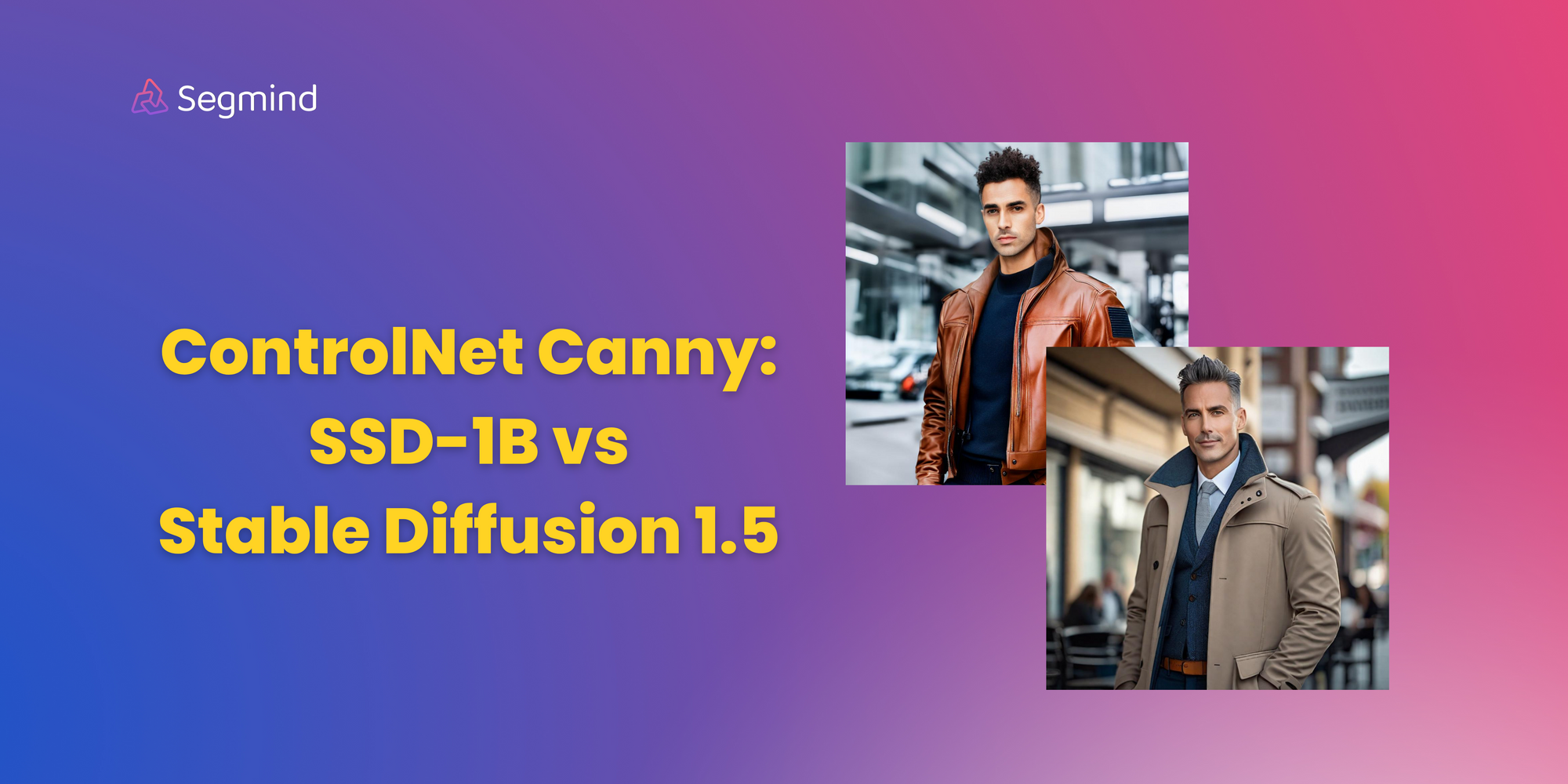
In this blog, we're diving into a cool way to transform images like never before. Think of it as not just highlighting, but defining and bringing out edges in every image at your fingertips.
Our focus will be on two powerful models — SSD-1B Canny and ControlNet Canny SD 1.5, both designed to elevate your image transformation experience. We're committed to a fair and comprehensive comparison of these models, ensuring you have a clear understanding of their unique strengths and capabilities.
So, let's dive deeper into the intricate details of both SSD-1B Canny and ControlNet Canny SD1.5. We'll explore their architectures, delve into their settings, and navigate the terrain of features that set them apart. By the end of this blog post, you'll be equipped with insights to choose the right model for your unique image enhancement requirements.
Architecture
ControlNet Canny SD1.5
ControlNet Canny SD1.5 enhances Stable Diffusion models by allowing users to specify features in output images. It's built upon the Stable Diffusion 1.5 model.
The model adds noise to a 'latent vector,' a representation of the image it aims to generate.
Here's where ControlNet Canny SD1.5 steps in, introducing a 'ControlNet layer' with neural networks. Each network manages a different aspect of generation, like edge intensity or position. Trained with supervised learning, the ControlNet layer uses images with 'Canny edges' and their generated counterparts. It learns to modify the generation process using these edges, controlling intensity, position, and shape.
Once trained, the ControlNet layer can generate images from any Canny edge image. Just provide the layer with the Canny edge image, and it produces a new image with matching edges. This extra layer of control ensures Stable Diffusion models incorporate essential features from the input Canny edges into the generated output.
SSD-1B Canny
The SSD-1B training algorithm relies on Canny edges as a core element to teach the model how to create images with matching edges. It uses Canny edges as weights, acting as controllers that influence the intensity, position, and shape of edges in the generated image.
In essence, SSD-1B Canny learns from Canny edges data, helping the model recognize important image features and seamlessly integrate them into Stable Diffusion model outputs. This process not only improves the model's accuracy in replicating edges but also enhances its understanding of essential image characteristics.
Consequently, the SSD-1B model excels in producing images that not only replicate provided edges but also show a higher level of accuracy and relevance to the input data underlying features.
Parameters of the models:
Steps:
This parameter represents the number of de-noising steps in the image generation process. It starts by adding random noise from the text input, creating a repeated cycle. In each cycle step, some noise is taken away, gradually improving the image quality.
When you increase the step value, it means more cycles of noise removal, resulting in higher-quality images. Essentially, a higher step count lets the model go through a more thorough refinement process, making images clearer and more visually appealing.
Guidance scale:
The guidance scale parameter is crucial for how closely the image generation aligns with the given text prompts. It ensures that the generated images closely match the intended meaning and context of the input text.
When set to a higher value, it strengthens the link between the generated image and the input text. However, this stronger connection comes at a cost – it reduces diversity and, consequently, the overall quality of the generated images.
Controlnet Scale:
This parameter affects how closely the generated image follows the input image and text prompt. A higher value strengthens the connection between the generated image and the given input.
It's important to note that setting the value to the maximum isn't always recommended. While higher values might enhance the artistic quality of the images, they may not capture the intricate details or variance needed for a comprehensive output.
Negative prompts:
Negative prompts offer users a way to guide image generation by specifying elements they wish to exclude without providing explicit input. These prompts act as a filter, instructing the generation process to avoid certain features based on user-provided text.
Scheduler:
In the context of the Stable Diffusion pipeline, schedulers are algorithms that are used alongside the UNet component. The primary function of these schedulers is integral to the de-noising process, which is executed iteratively in multiple steps. These steps are essential for transforming a completely random noisy image into a clean, high-quality image.
The role of schedulers is to systematically remove noise from the image, generating new data samples in the process. Among the various schedulers, UniPC is highly recommended.
Image comparisons between both models:
We will now take a look into how both these models stack up based on a few key factors, we would like to compare them based on
- Prompt Adherence: This tells us how well the models follow the given instructions.
- Art Styles and Functionality: We're exploring the diversity and quality of artistic styles, as well as evaluating how well these models handle lens and subject modifications
By examining these aspects, we'll better understand what each model can do. Just to mention, all the parameter values in the models are set to their best values, making it a fair comparison.
Prompt Adherence:
Prompt adherence is about how well a model follows the given text instructions to make an image. But it's worth noting that there isn't a specific way to measure how good a model is at this. Good instructions act like a guide, helping the model create images that match the intended idea.
Let’s get started by trying simple prompts and then later increase the complexity of the prompts
Prompt : Asian woman with frizzy dark hair smiling wearing a overcoat
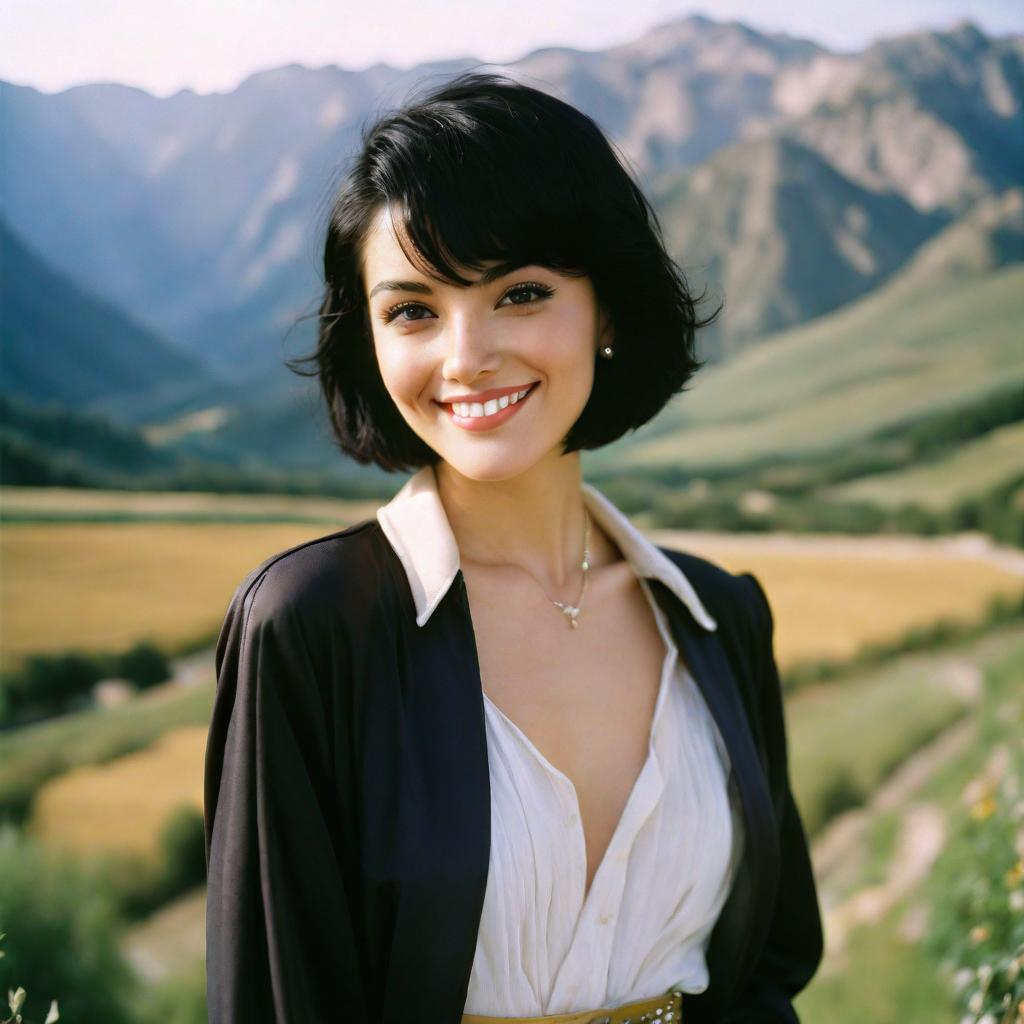
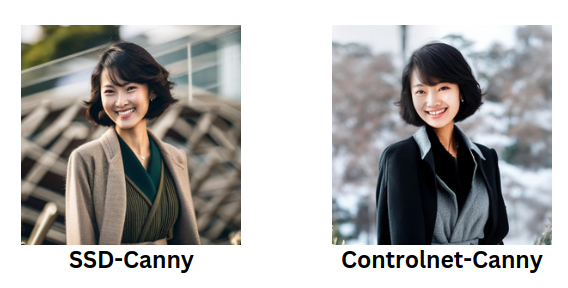
Prompt : American women wearing sweater with long hair standing
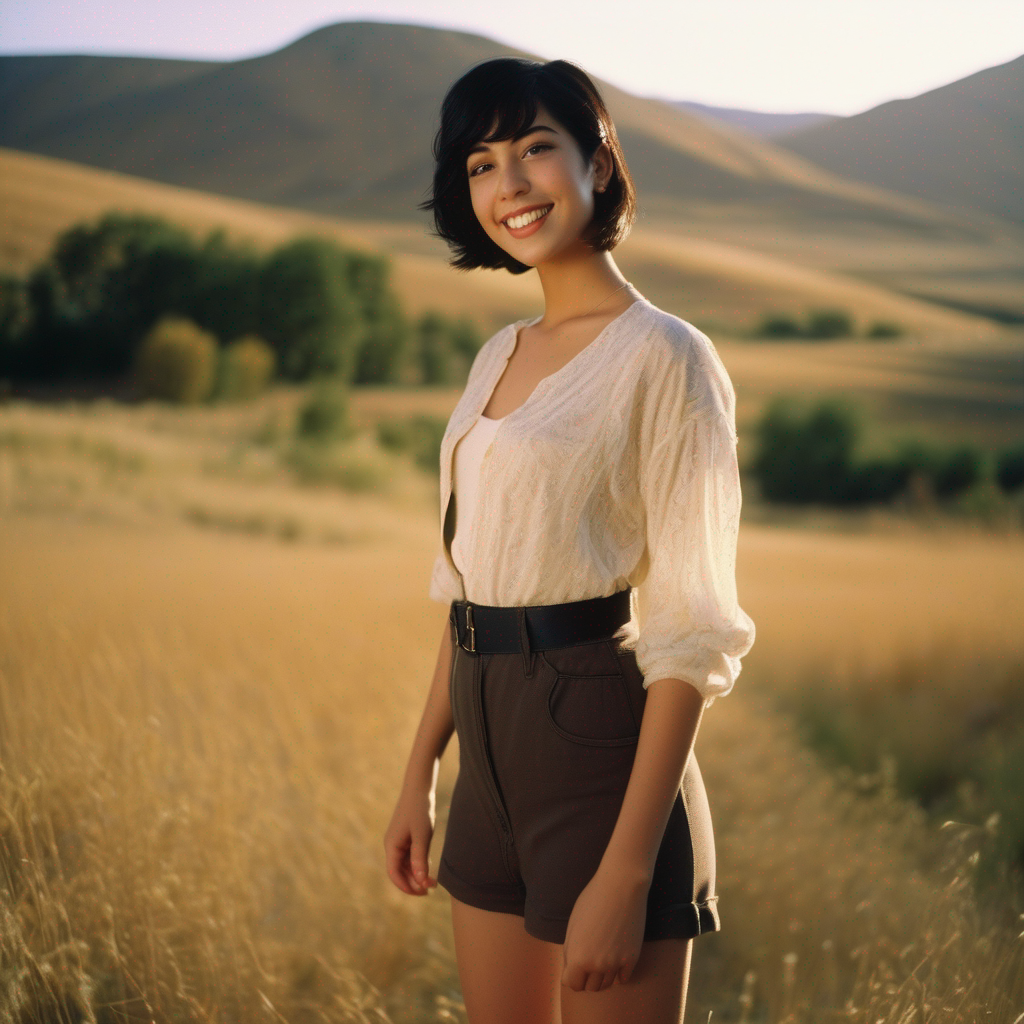
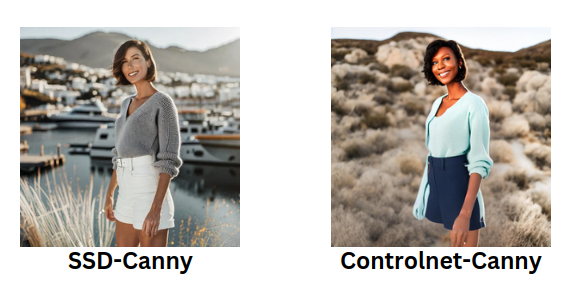
We notice that ControlNet Canny SD1.5 faces challenges with straightforward prompts. In the image above, you can see that the ControlNet-Canny SD1.5 model struggled to identify the edges in the legs of the input image. As a result, it generated an image where one of the legs was missing. In contrast, the SSD model successfully detected the edges and made the necessary changes according to the prompt.
Moving on let us try some more images with different poses as well as complex prompts.
Prompt : front view, a happy stunning Indian woman with curly long dark hair beautiful eyes trimmed eyebrows, wearing red clothes standing in a beautiful field of rose,
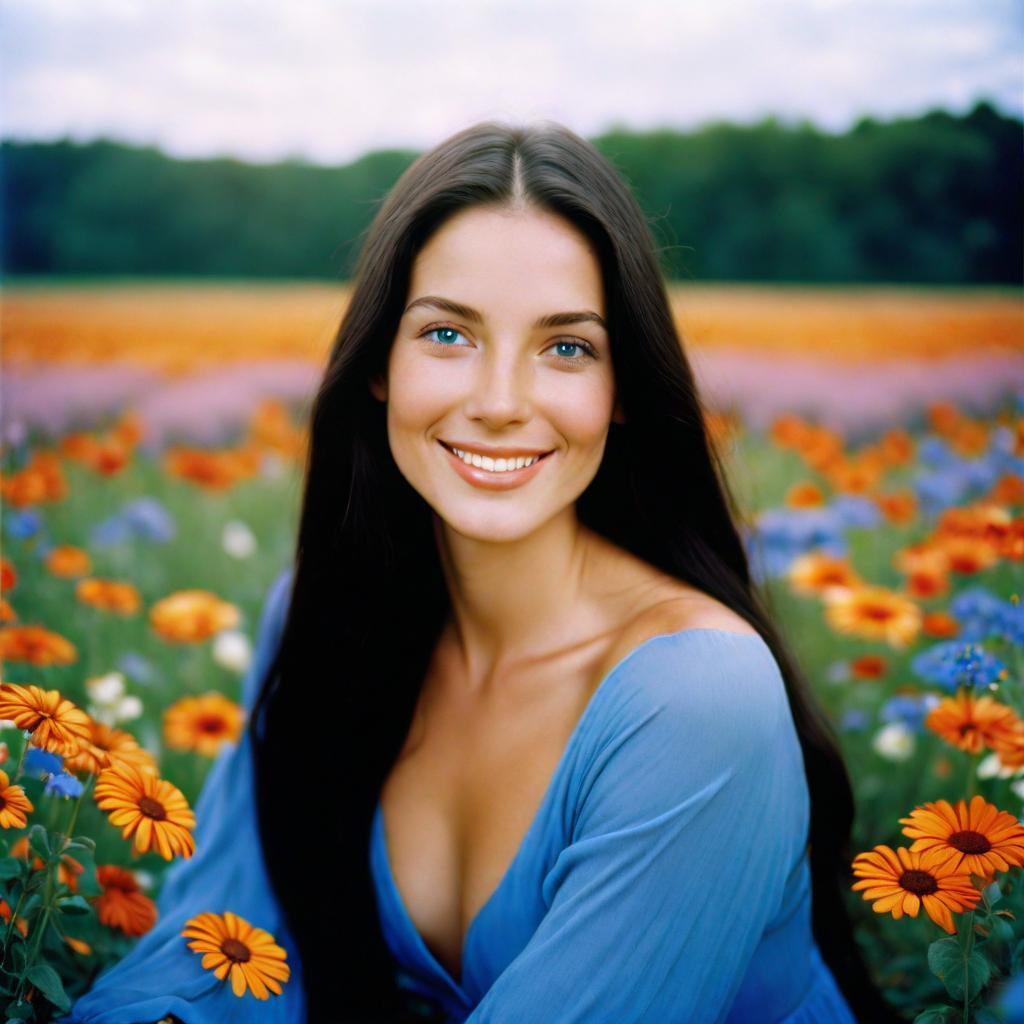
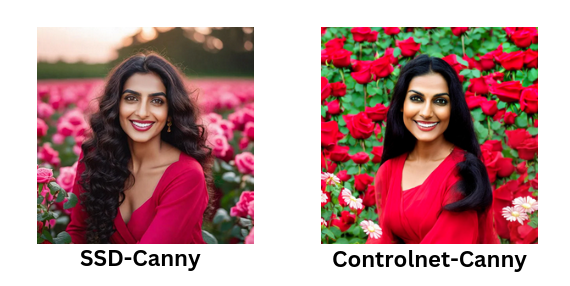
Prompt : Gaucho man organizing the playing chess on a table, on a park square
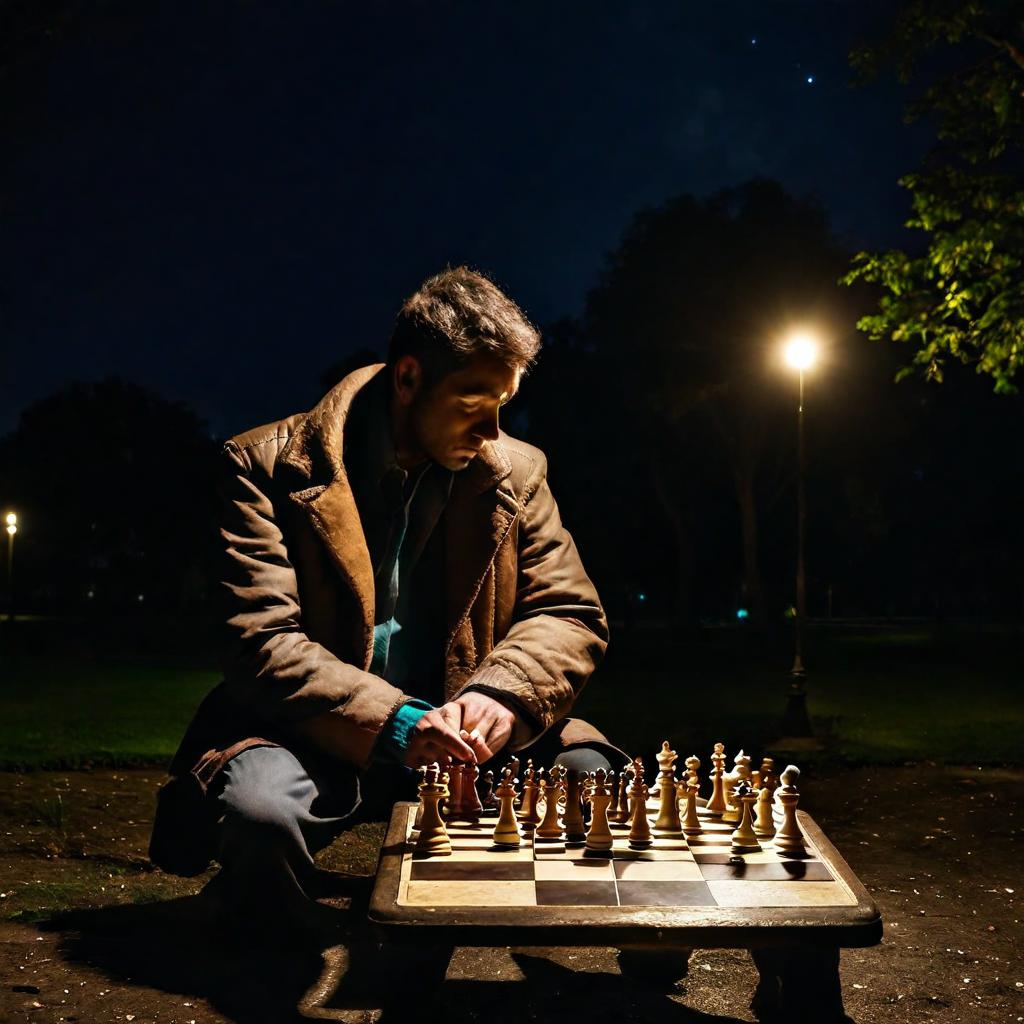
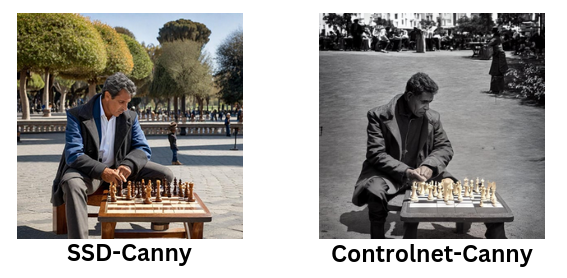
Prompt : An old man yelling with a phone in his hand sitting on a bench , pixar style
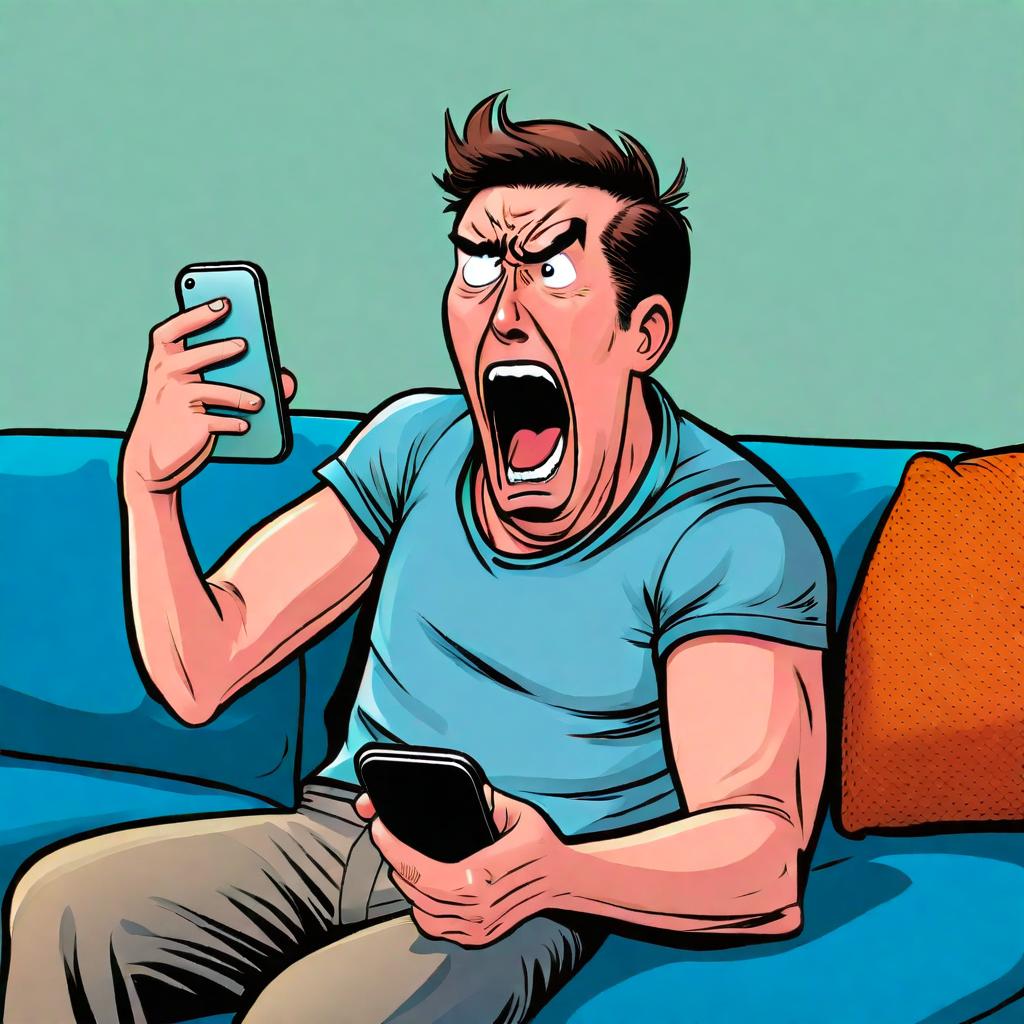
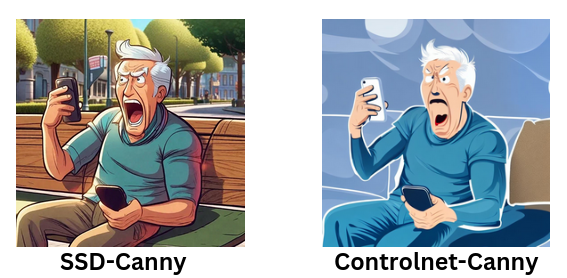
Examining the images above reveals a significant variation in the outputs of both models. SSD-Canny SD1.5 stands out as notably superior to the ControlNet Canny SD1.5 model. It excels in producing images with enhanced depth and exhibits a higher artistic quality. While ControlNet Canny SD1.5 manages to capture the edges present in the input image, its text adherence quality is lacking, resulting in images that appear to be of lower quality.
The SSD-Canny SD1.5 model not only reproduces the specified edges effectively but also adds a layer of richness, creating visually compelling images. In contrast, the limitations in text adherence quality observed in the ControlNet Canny SD1.5 model impact the overall quality of its generated images, indicating a need for improvement in this aspect.
Art Styles and Functionality :
Looking at the different art styles and what these models can do gives us a good idea of their artistic abilities. To dig deeper into this, we'll give both models various prompts. This helps us thoroughly test and compare what they can do, just like we did with the previous criteria.
Now let us try to transform this futuristic Cyberpunk image of a woman into an American woman wearing a turtle captured by Fujicolor C200.
Prompt : american woman wearing turtleneck standing under a telephone booth , Fujicolor C200
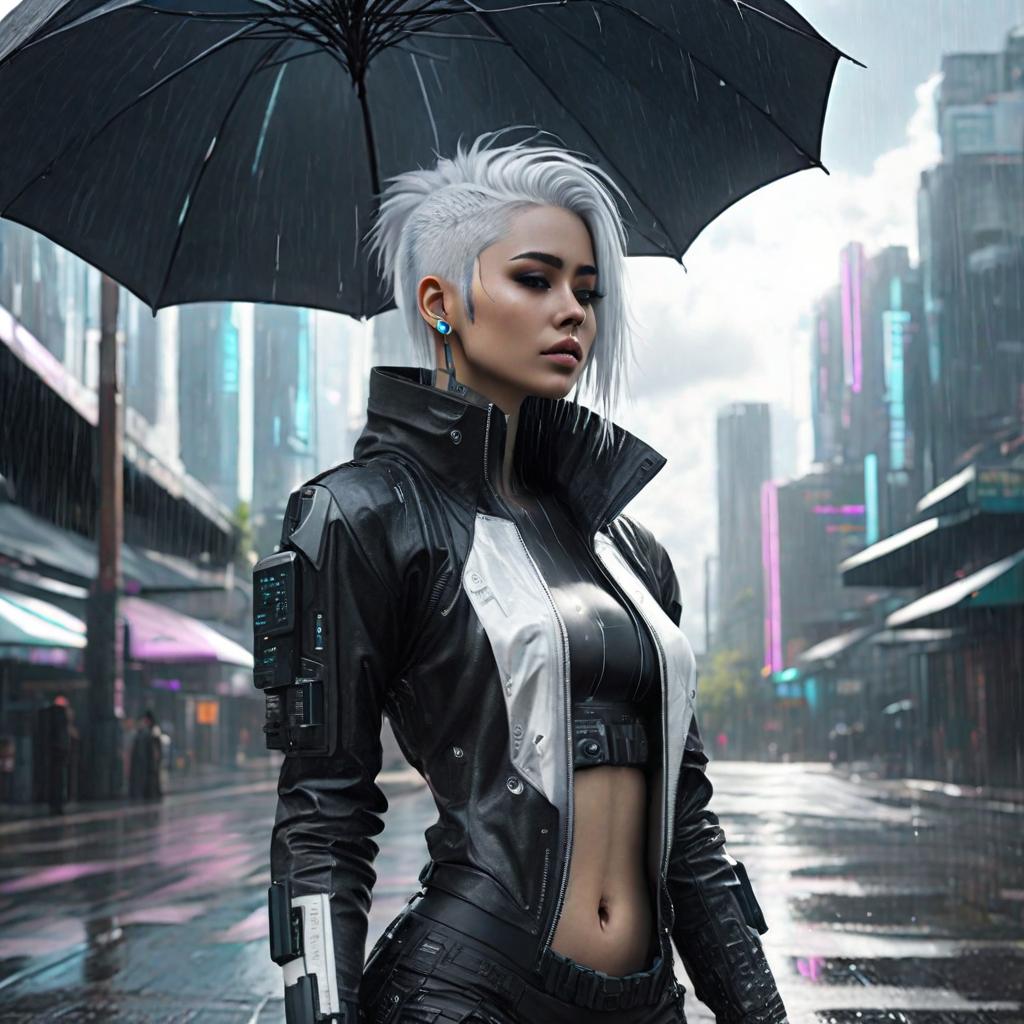
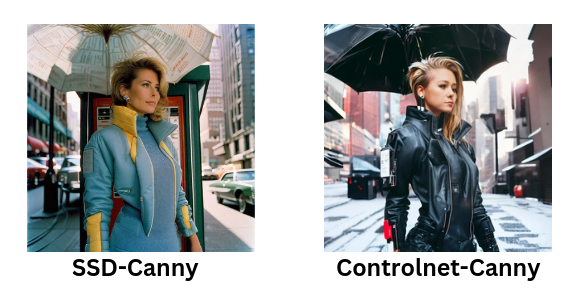
In observing the ControlNet Canny SD1.5 model, although it can make alterations to the dress and the pose is captured perfectly, there is a noticeable struggle to fully adhere to the given prompt. Additionally, changing how the subject is captured, as specified in the prompt, seems to present challenges for this model. On the other hand, SSD-Canny SD1.5 showcases its proficiency by not only modifying the image content but also successfully altering the way the subject is captured, in line with the provided instructions.
Let's experiment with an image depicting the subject captured using a specific type of lens and camera style
Prompt : Handsome man in a coat suit , nostalgic and hazy, shot on Sony FE 24-70mm f/2.8 GM Lens
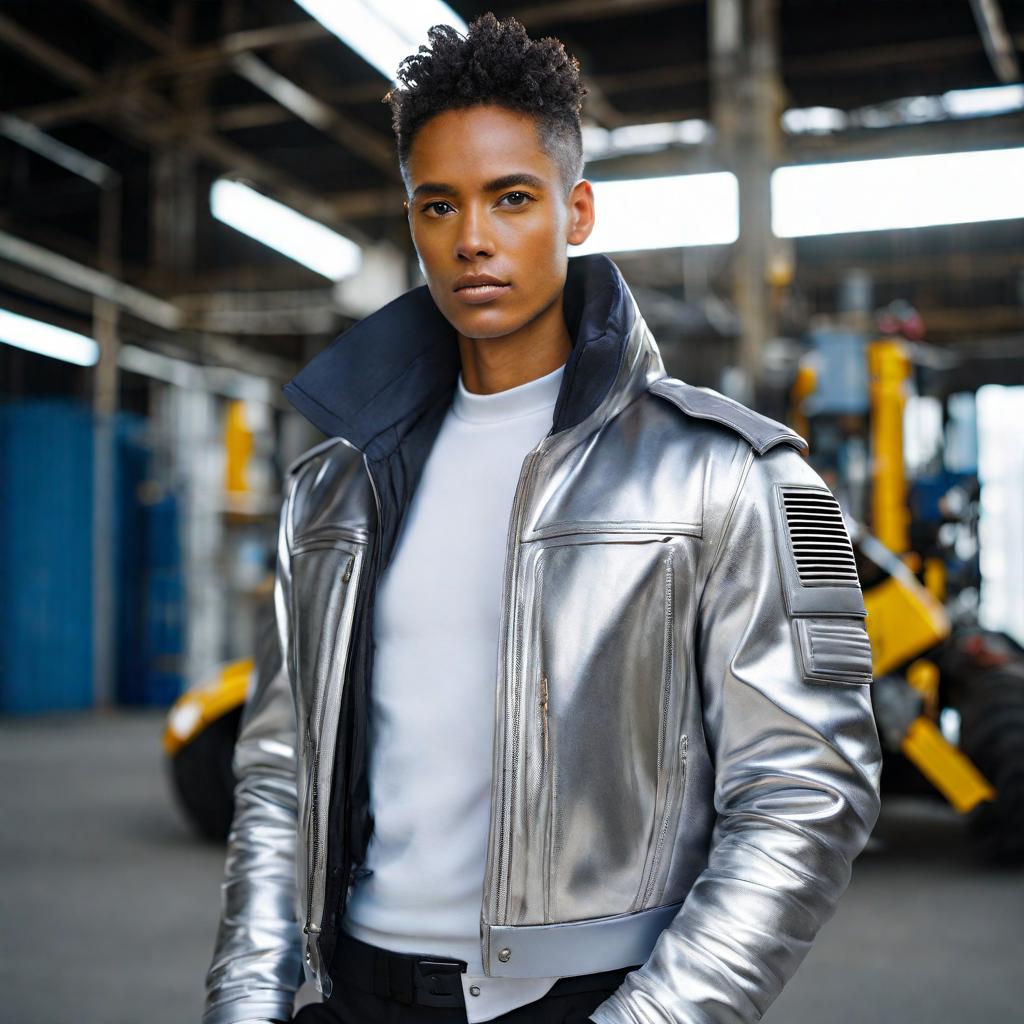
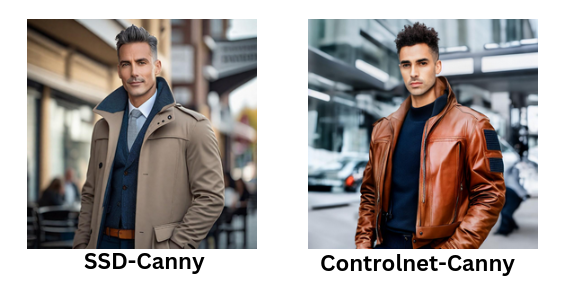
The image quality generated by SSD-Canny SD1.5 appears professional and successfully captures the depth typically associated with that lens. In contrast, the image produced by the ControlNet Canny SD1.5 model falls short in terms of quality and fails to convey the intended depth characteristic of the lens.
Let's introduce a quirky prompt with an unconventional image featuring a peculiar pose to assess the models' ability to detect edges and modify the image based on specific prompts.
Prompt : Superman in comic style flying through the streets of New York, Marvel comic style , sunny and backlighting
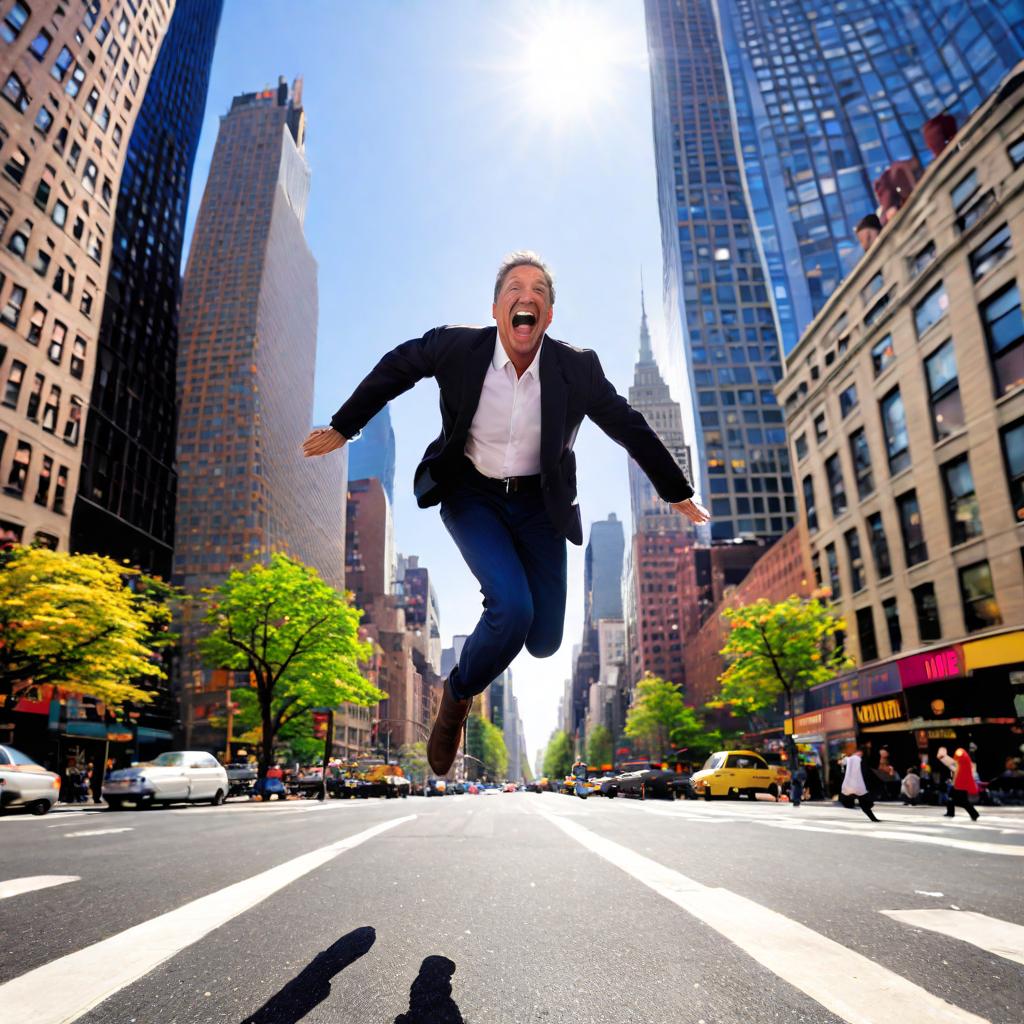
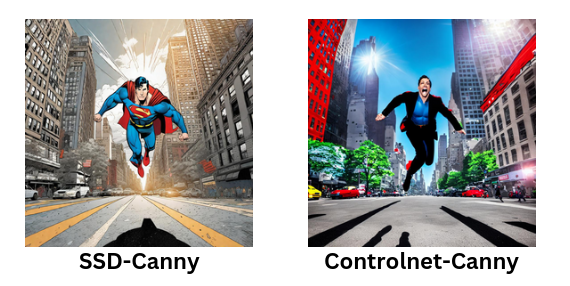
The image generated by SSD-Canny SD1.5 far exceeded my expectations. Not only does it successfully adapt the subject in line with the prompt, but it also extends its capabilities to modify the surroundings and maintain the specified lighting pattern. On the contrary, the ControlNet Canny SD1.5 model falls short, showcasing limitations in adhering to prompts effectively.
Let us now try various styles are check the results. We'll begin by giving both models a straightforward prompt: to replicate the image in an oil painting style.
Prompt : A person running on a track, oil painting
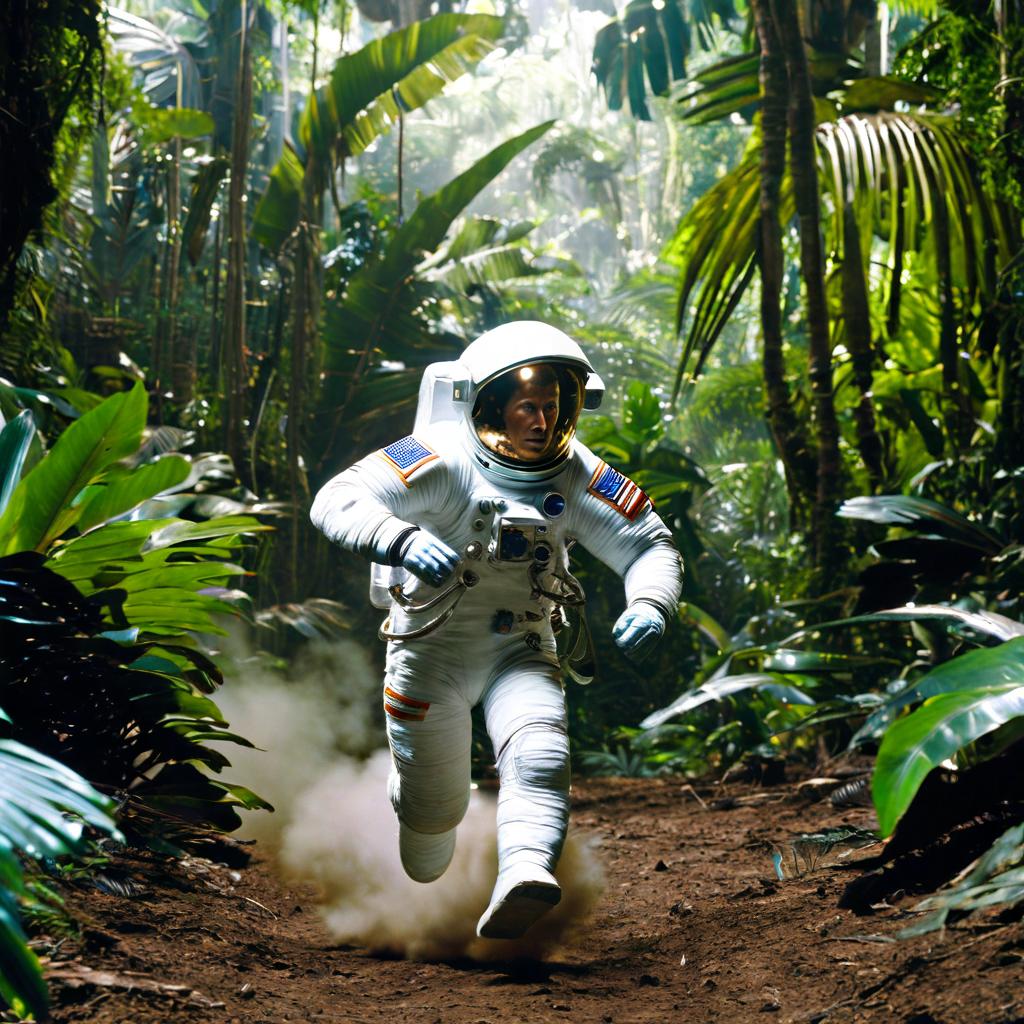
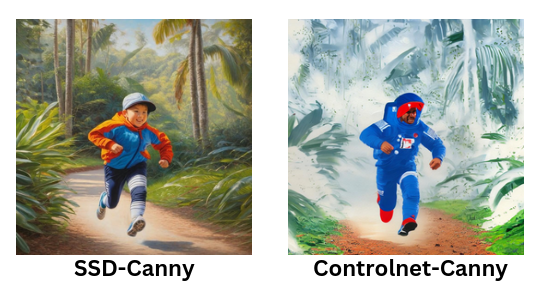
Prompt : A woman smiling and standing, peaceful, digital art , sci-fi
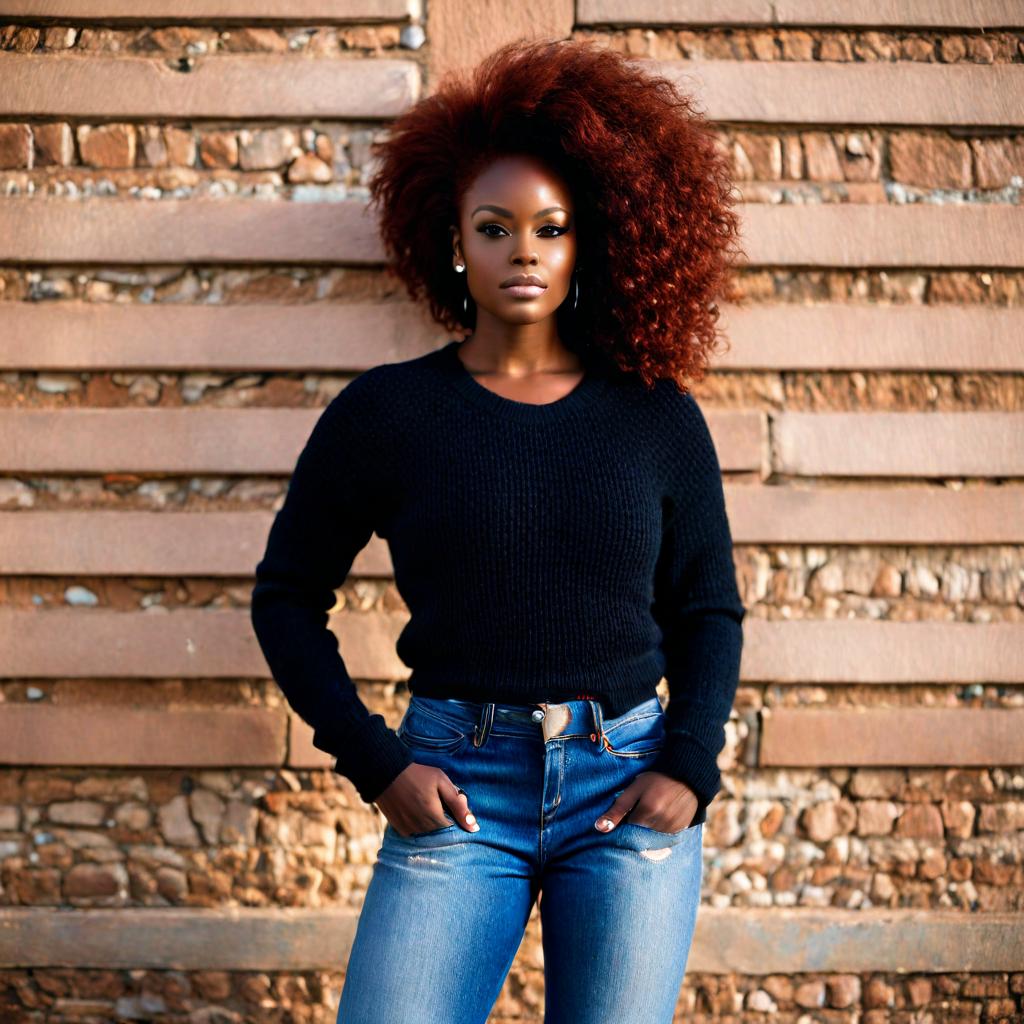
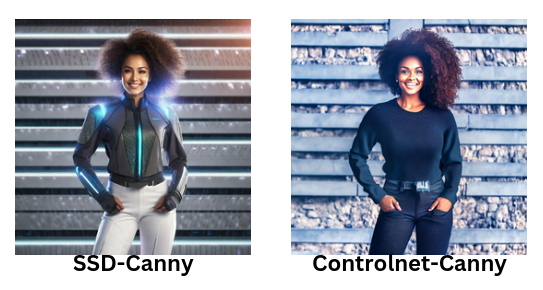
Prompt : An ultra-detailed beautiful panting of a stylish girl wearing streetwear in anime art style
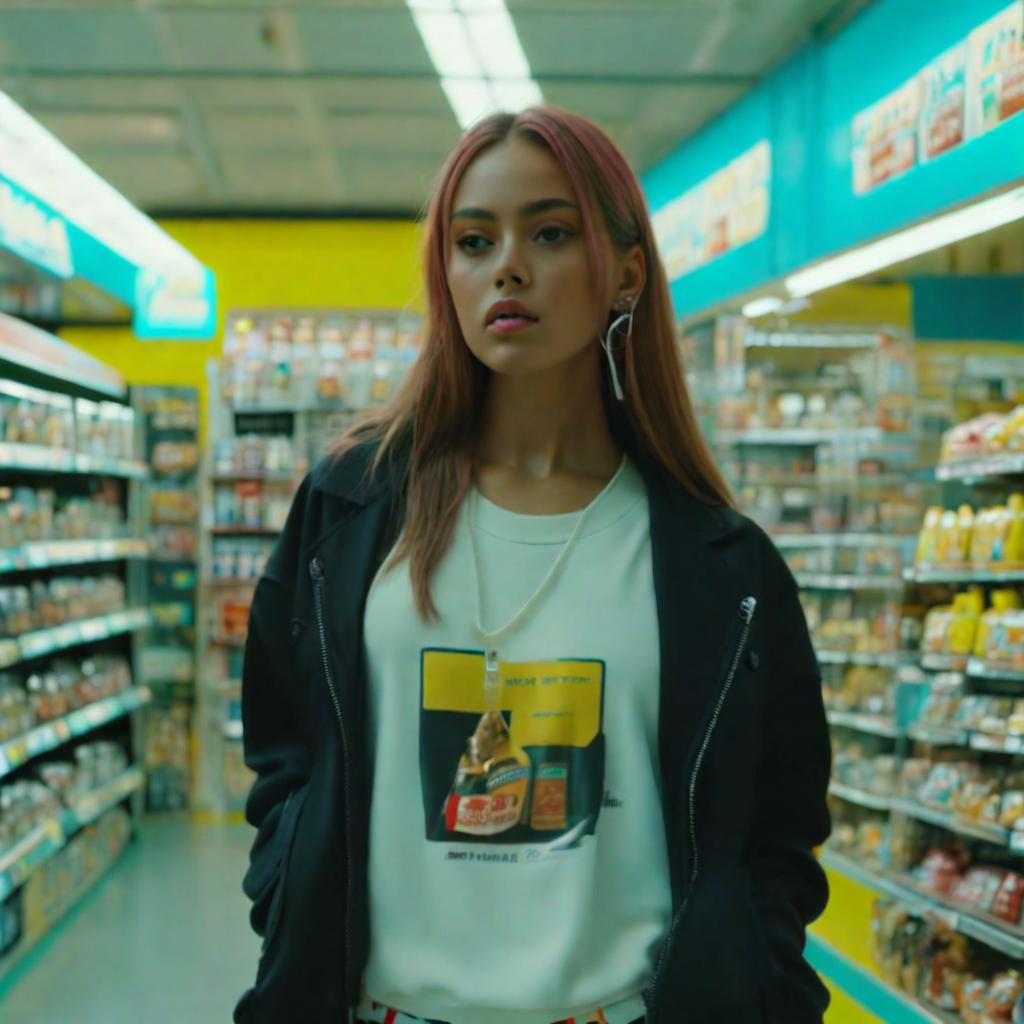
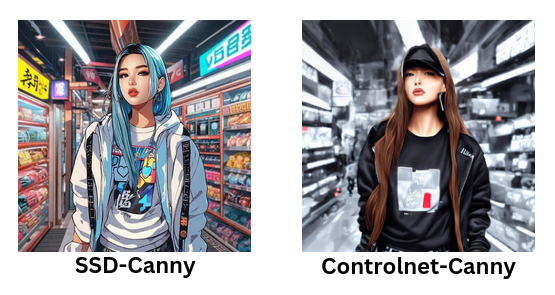
We get to see various prompts ranging from simple blunt descriptions of our intention to extremely detailed and highly specific and find that the SSD-1B Canny model gets to produce far superior images high in artistic quality.
The ControlNet-Canny model excels at edge detection but struggles to effectively modify images according to prompts. Its performance is marked by poor quality, especially in transforming facial features, resulting in sub optimal outcomes for eyes and other facial elements.
Conclusion:
In conclusion, the SSD-1B Canny model emerges as a powerful and versatile tool in the realm of image processing, building upon the robust canny edge detection foundation. Its precision in detailing as well as flexibility in image modification is tailored to the user's preferences.
In our comparison, the SSD-1B Canny consistently shines as the superior model when tested against ControlNet Canny SD1.5, demonstrating its excellence across various prompts.