Detailed Comparison of ControlNet Openpose and SDXL-Openpose
This blog post covers a detailed side-by-side comparison of the SDXL Openpose with SD 1.5 ControlNet Openpose, aiming to dissect their capabilities and performance.
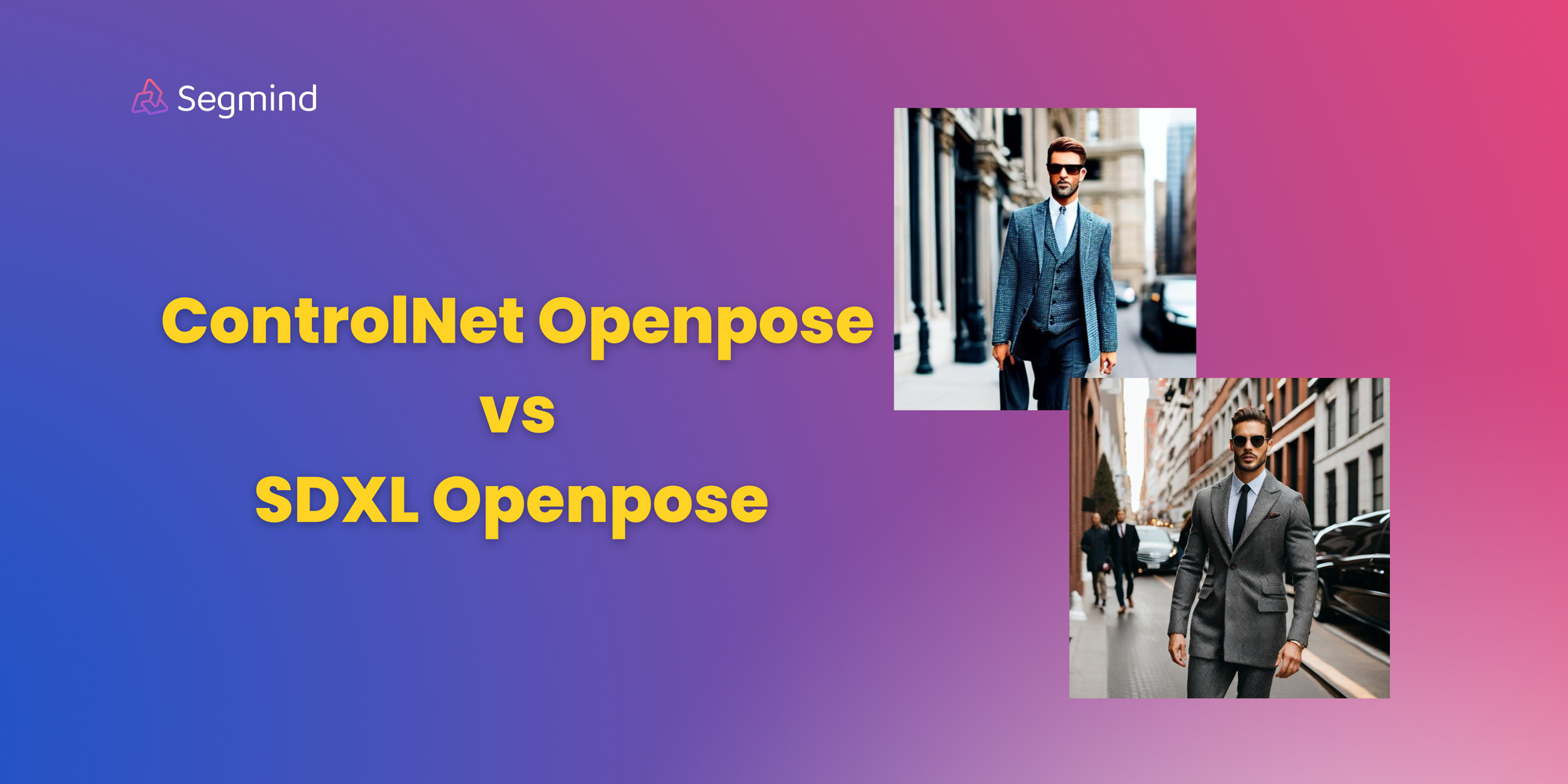
In this blog post, we will take a look at two models that transform the landscape of human pose estimation SDXL-Openpose and ControlNet Openpose.
The SDXL-openpose model combines the control capabilities of ControlNet and the precision of OpenPose, setting a new benchmark for accuracy within the Stable Diffusion framework.
Crafted through the thoughtful integration of ControlNet's control mechanisms and OpenPose's advanced pose estimation algorithms, the SDXL OpenPose Model stands out for its ability to process visual data with exceptional precision. But that's not all – we will delve into a comparison between SDXL Openpose and Controlnet Openpose. Let’s dive into the architecture.
Architecture:
ControlNet architechture:
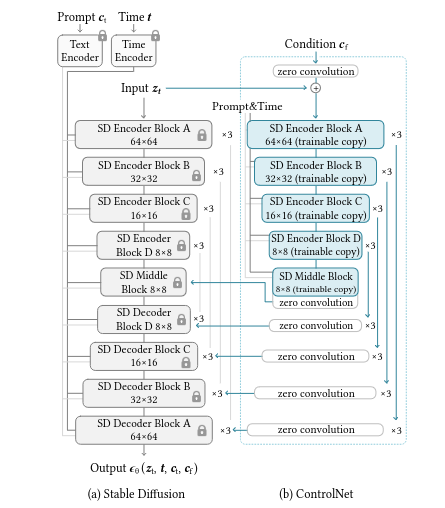
This serves as the base for both the models we will be comparing today.
The ControlNet architecture is designed for acquiring a diverse set of conditional controls. It employs a unique method called "zero convolutions," utilizing convolution layers initialized with zeros.
These layers gradually build parameters from zero, creating a controlled and noise-free environment during fine-tuning. This technique acts as a safeguard against disruptive noise, preserving the integrity of the pre-trained model. Crucially, it enables the smooth integration of spatial conditioning controls into the model.
How Controlnet Openpose work?
The strength of the ControlNet model is in leveraging the OpenPose model's ability to discern intricate details in human poses, such as the positions of hands, legs, and head. This proficiency results in accurate replications of human poses.
ControlNet takes these key points and transforms them into a control map. This map, combined with a text prompt, is then input into the Stable Diffusion model. The result is the generation of images that not only replicate the original pose but also grant users creative freedom to refine other attributes like clothing, hairstyles, and backgrounds.
How does SDXL- Openpose work?
SDXL-Openpose utilizes the Openpose model. In this intricate process, ControlNet assumes a pivotal role by transforming these key points into a control map. This map, when combined with a text prompt, becomes the input for the Stable Diffusion model. The outcome is the generation of images that faithfully replicate the original pose, all while affording users the freedom to exercise creative flexibility.
Parameters of the model:
- Guidance scale
The guidance scale parameter plays a vital role in determining how closely the generated images match the provided text prompts. It ensures that the generated images faithfully capture the intended meaning and context of the input text prompts. Increasing the value of the guidance scale strengthens the connection between the generated image and the input text. However, it is important to acknowledge that a stronger connection comes at a cost.
- Steps
This parameter concerns the number of denoising steps, indicating the iterations in a process initiated by random noise from the text input. In each iteration, the model refines the image by progressively removing noise. A higher number of steps results in the increased production of high-quality images.
- Controlnet scale
This parameter shapes how closely the image generation process follows both the input image and the given text prompt. A higher value strengthens the alignment between the generated image and the provided image-text input.
Nevertheless, setting the value to the maximum isn't always recommended. While higher values may impact the artistic quality of the images, they don't necessarily capture the intricate details required for a comprehensive output.
- Negative prompt
This parameter allows users to specify the elements they wish to exclude from the generated images without providing explicit input. These prompts guide the image generation process, directing it to avoid certain features based on user-provided text.
By employing negative prompts, we can effectively block the generation of specific objects and styles, address image abnormalities, and enhance overall image quality.
- Schedulers
Within the Stable Diffusion pipeline, schedulers play a pivotal role in collaboration with the UNet component. Their primary function is crucial to the denoising process, executed iteratively across multiple steps. These steps are instrumental in the transformation of a randomly noisy image into a clean, high-quality image. Schedulers systematically eliminate noise from the image, generating new data samples in the process. Noteworthy schedulers in this context including Euler and UniPC are highly recommended in general and might vary for particular contexts.
Image comparisons between both models:
We will now take a look into how both these models stack up based on a few key factors, we would like to compare them based on
- Prompt adherence: How well the models stick to the given instructions.
- Functionality: The overall performance and capabilities of the models.
By looking at these aspects, we can get a clearer picture of what each model brings to the table. As a side note, all parameter values in the respective models are fixed according to our findings.
Prompt Adherence:
Prompt adherence is about how well a model follows given instructions to make an image. Notably, there's no precise framework or metric to measure this in text-to-image models. Well-crafted prompts act as a guide, helping the model create images aligned with the intended vision. While there's no universal method to gauge prompt quality, a creative and clear prompt usually leads to more accurate and visually appealing results.
Let's start with simple prompts and then move on to extremely detailed prompts that specify individual nuances in the images.
Prompt : a beautiful fashion model standing in a road , wearing a red polka dress
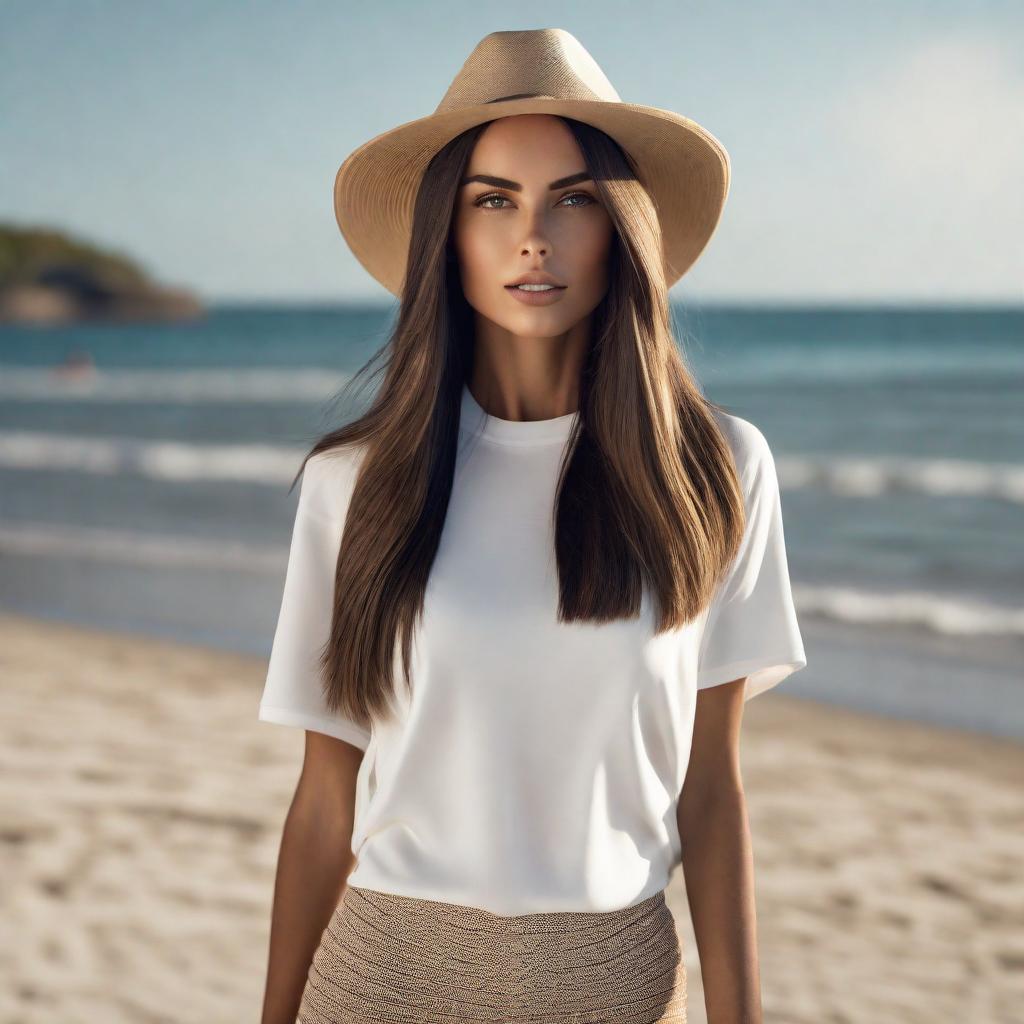
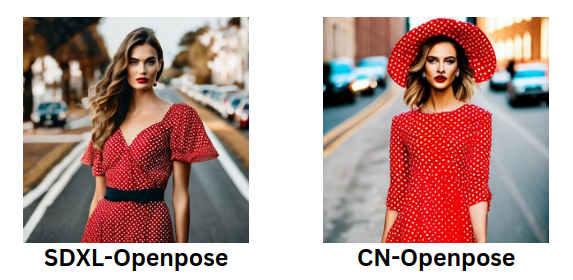
Prompt : a handsome male model with a coat and suit walking down the street
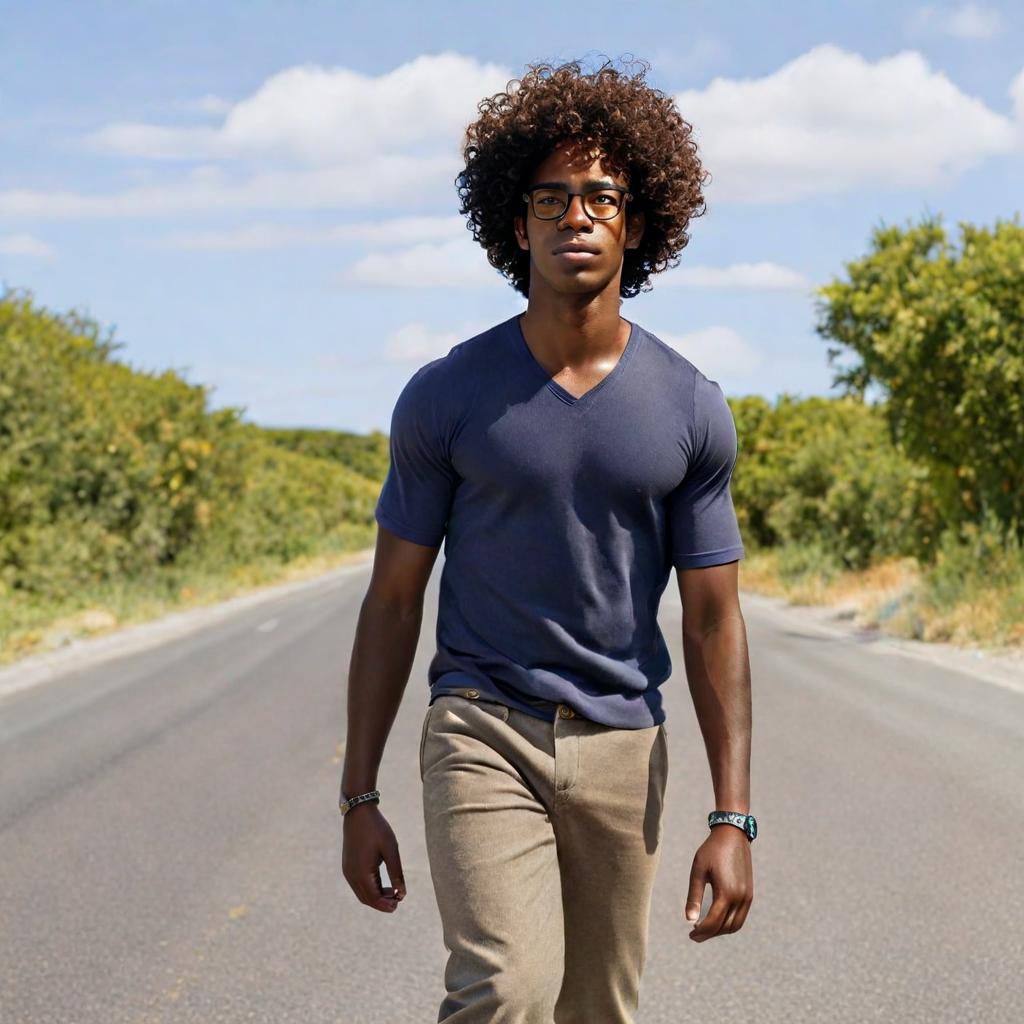
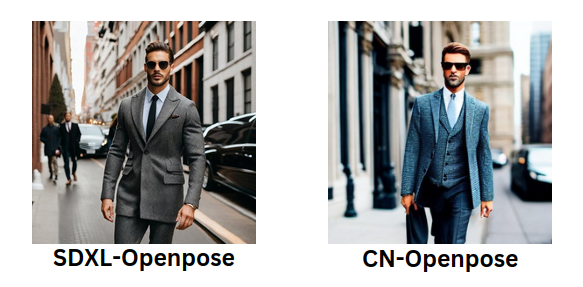
The ControlNet OpenPose models encounter challenges when attempting to modify an existing image based on straightforward prompts. In comparison, SDXL-OpenPose not only exhibits superior quality and intricate details but also adds depth to the image. In contrast, the ControlNet OpenPose model tends to make partial modifications, resulting in noticeable inconsistencies.
Prompt : 4k photo portrait of a beautiful woman in a red raincoat, looking at the camera, at a bustling crosswalk in night , cinematic
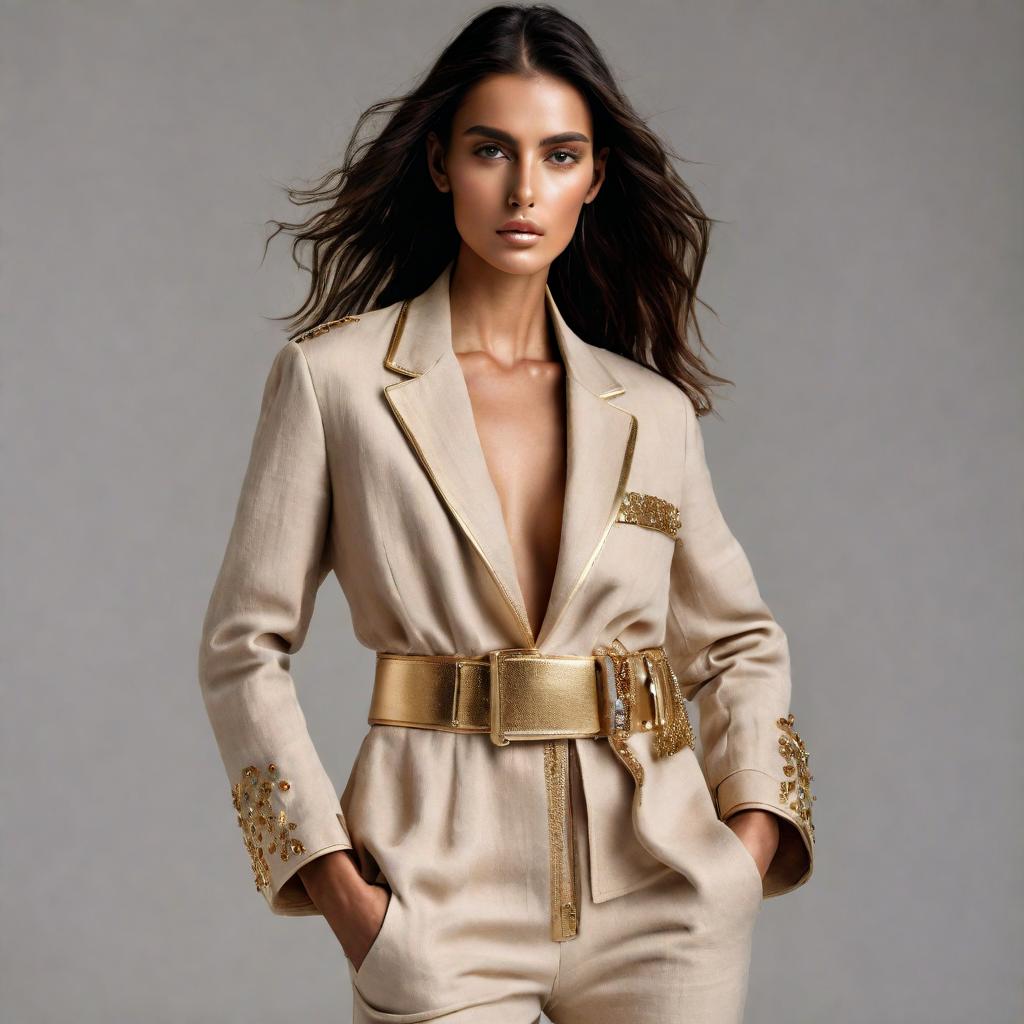
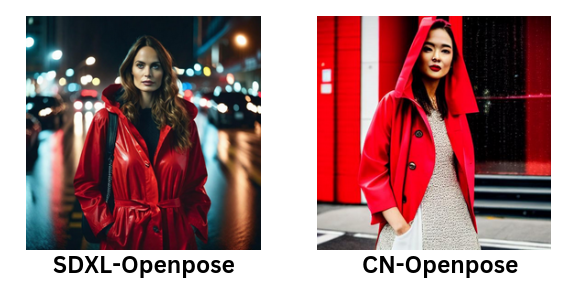
Prompt: Cute adorable little girl , unreal engine, cozy interior lighting, art station, detailed digital painting, cinematic, character design by Mark Ryden and studio ghibili , unreal 5, daz, hyper realistic, octane render
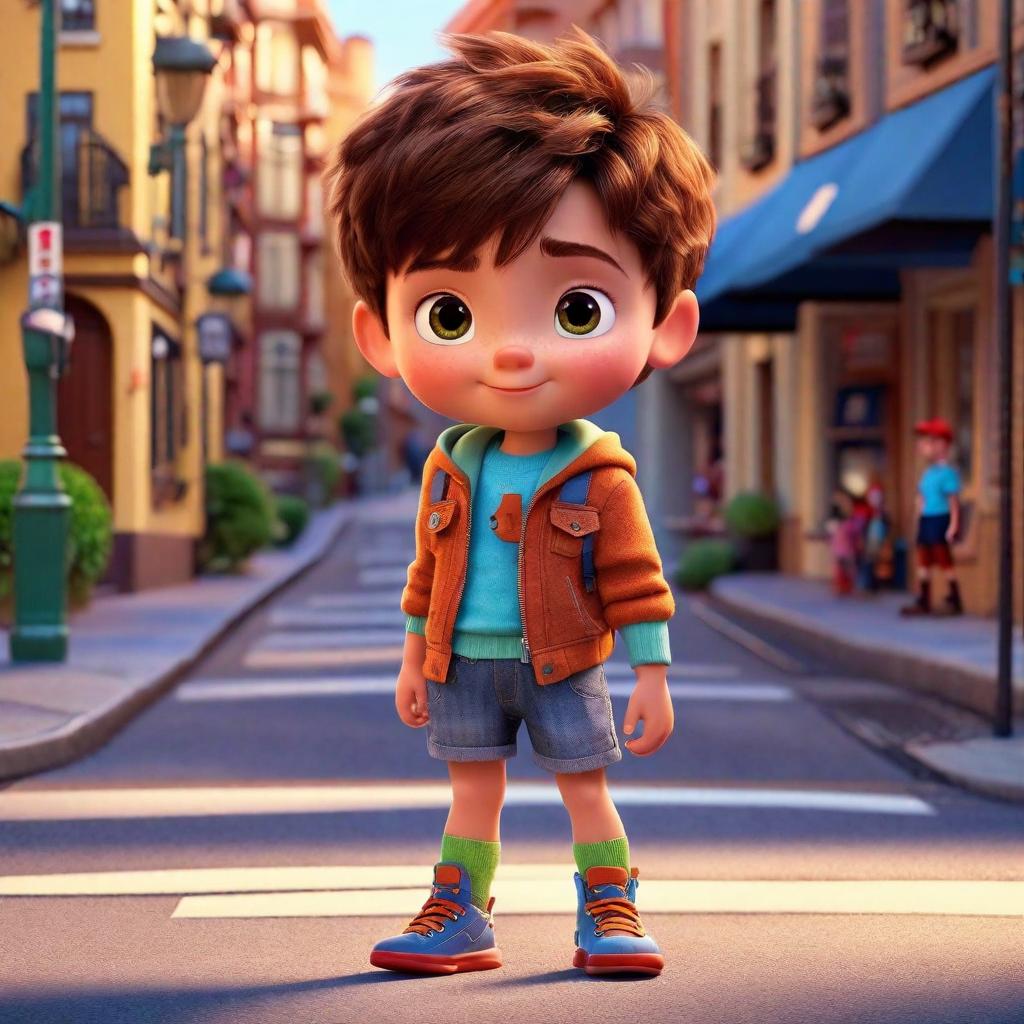
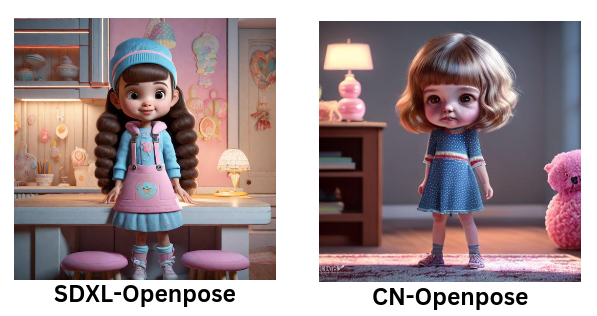
Prompt : A wise old man with white beard and bakoua hat in a tropical setting, sitting on a straw chair, shot on ArriAlexa
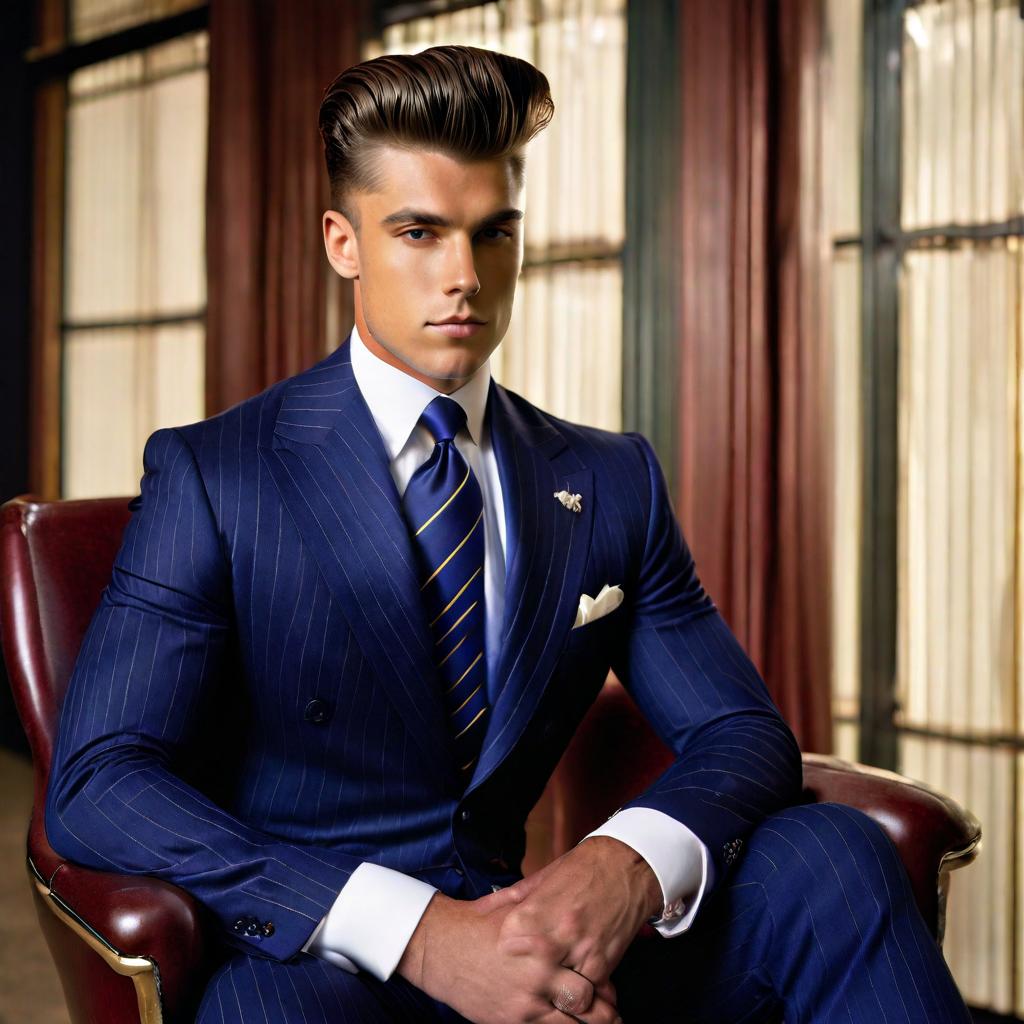
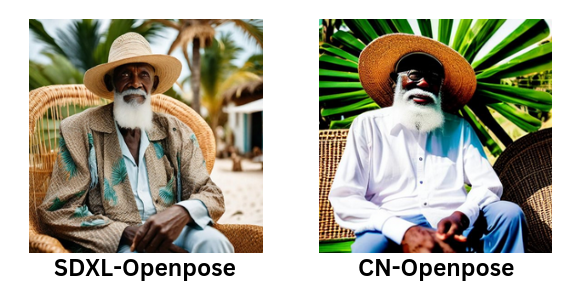
Looking at the examples above, SDXL-OpenPose stands out for following prompts well and delivering high-quality images. It offers more depth and finer details, like hair and skin texture, compared to ControlNet OpenPose. SDXL-OpenPose also handles background details specified in prompts effectively.
On the flip side, while ControlNet OpenPose understands the subject's pose, the generated images based on prompts lack quality. The details aren't up to par, resulting in less visually appealing outcomes. This difference emphasizes SDXL-OpenPose's strength in producing more refined and detailed results.
Functionality:
We will now judge the model’s proficiency in transforming text into images, considering the range of instructions it can interpret and its creative capabilities. Assessing functionality provides insights into the model's strengths and potential limitations.
Let us now modify this image such that we have a woman sitting in the park wearing a shirt and jeans.
Prompt : a american women sitting under the tree in a park , cinematic , shirt and jeans, wide shot , detailed
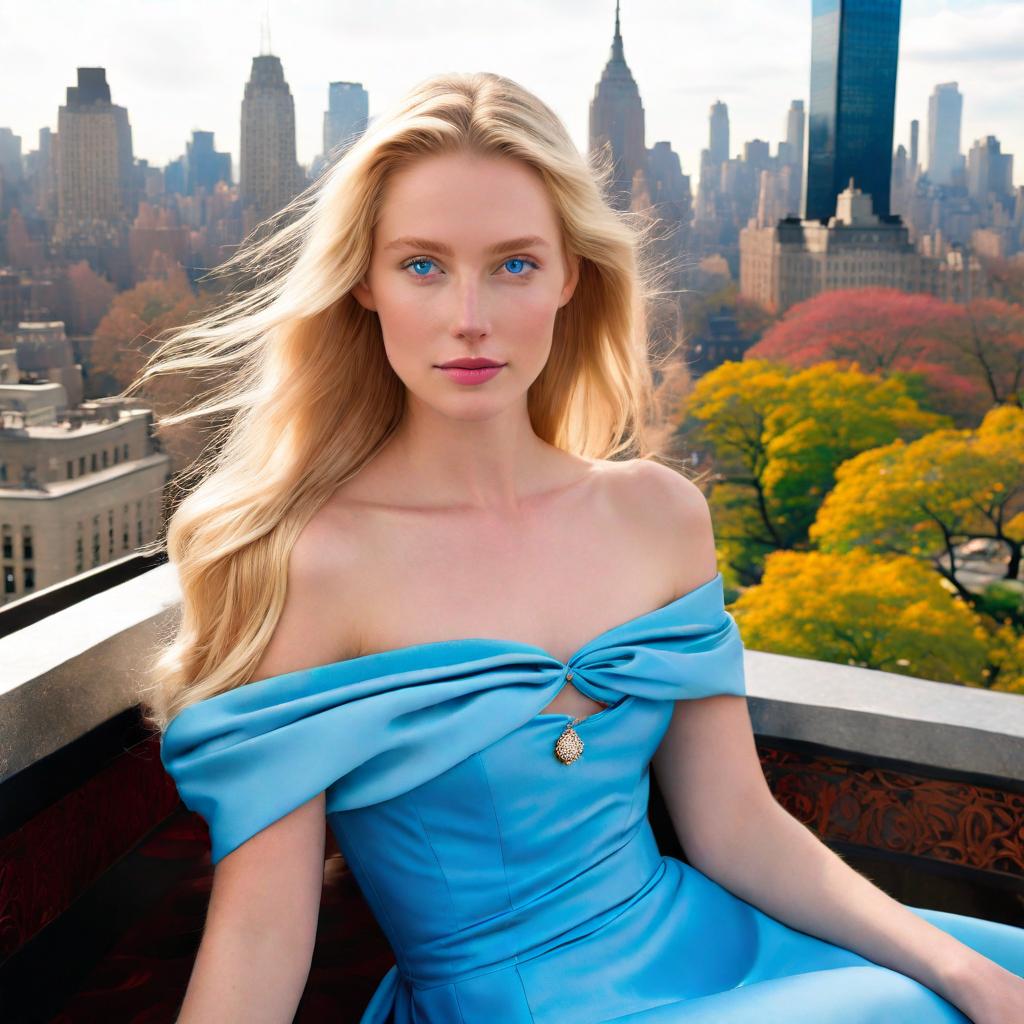
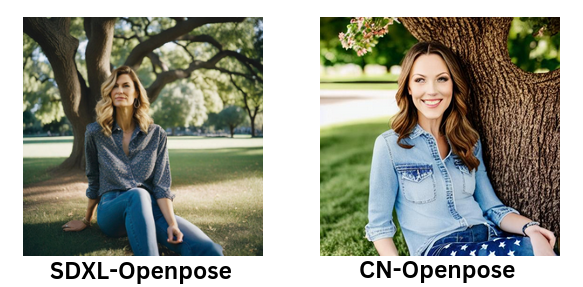
Observing the image generated by ControlNet OpenPose reveals blurred and disproportionate facial features, even when negative prompts are specified appropriately. Additionally, there's a stark contrast in the quality, color, and depth between the two images.
Moving on let us convert this image into a Pixar-style image of a young boy holding a camera in his hand
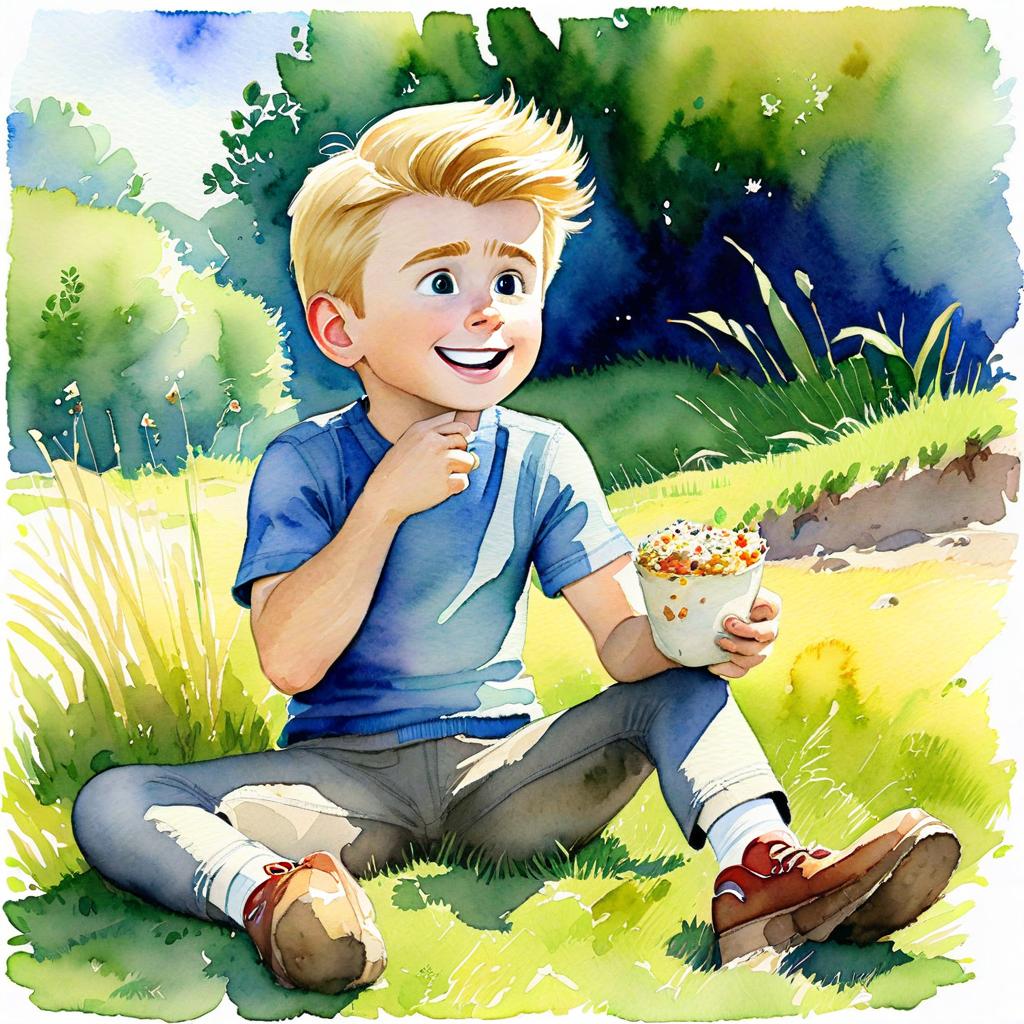
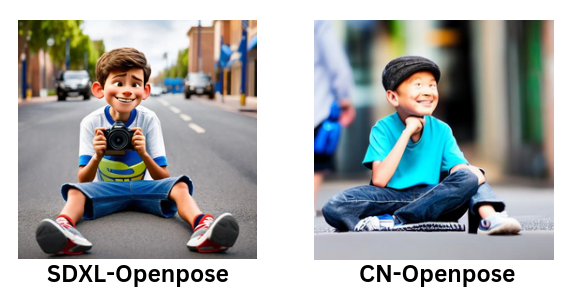
In this example, ControlNet OpenPose once again generates a subpar image. Although it can somewhat understand the image's pose, it notably struggles to adhere adequately to the prompt. On the other hand, while SDXL OpenPose can produce high-quality artistic images, it occasionally falls short in accurately capturing poses.
Let us now move on to an example which is quite detailed regarding the style of the image we desire
Prompt : full body of a Handsome, athletic black male with short hair, perfect face clean shaven, a very handsome muscular man, a hero wearing workout clothes, at New York City park, full body, 8k resolution concept art portrait dynamic lighting hyperdetailed intricately detailed splash art trending on artstation unreal engine 5 volumetric lighting
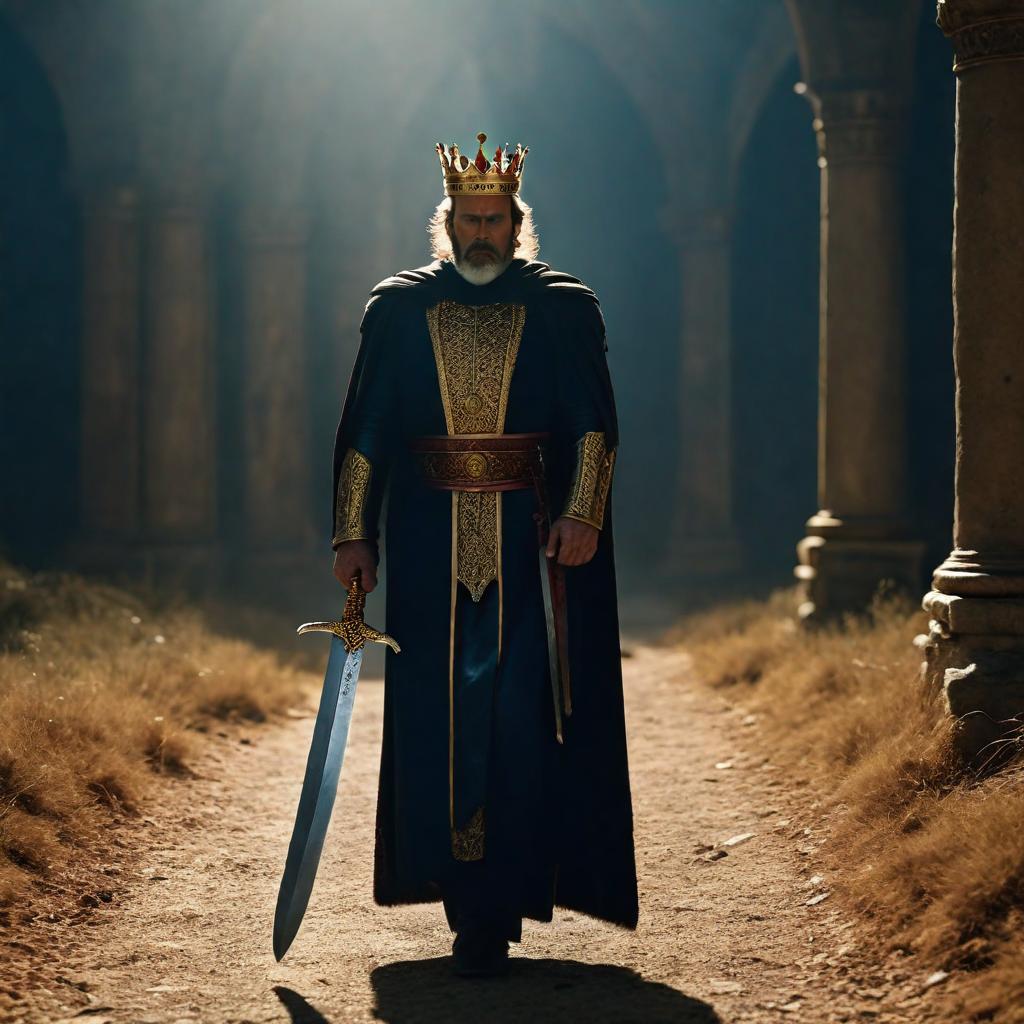
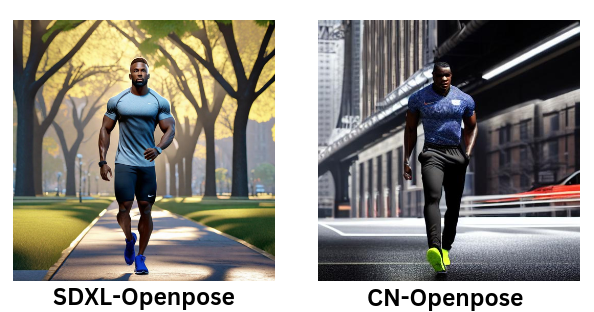
While both models successfully replicate the pose of the input image, there are notable differences in the quality of the generated images. The SDXL OpenPose model exhibits significantly higher quality, adhering closely to the prompt and reproducing similar features. However, upon closer inspection, it becomes evident that the legs appear disfigured and out of shape.
Conclusion:
Both models demonstrate proficiency in understanding and controlling human pose analysis when presented with an input image. However, they encounter challenges when faced with complex poses. ControlNet OpenPose struggles to generate images of high artistic quality, lacking well-defined features of the subject. In comparison, SDXL-OpenPose excels, showcasing superior abilities in translating images according to the desired prompt, marking a significant advancement over ControlNet OpenPose.