A Deeper Dive: SSD Depth vs ControlNet Depth
In this blog post, we do a head-to-head comparison between SSD-1B Depth and SD 1.5 ControlNet Depth.
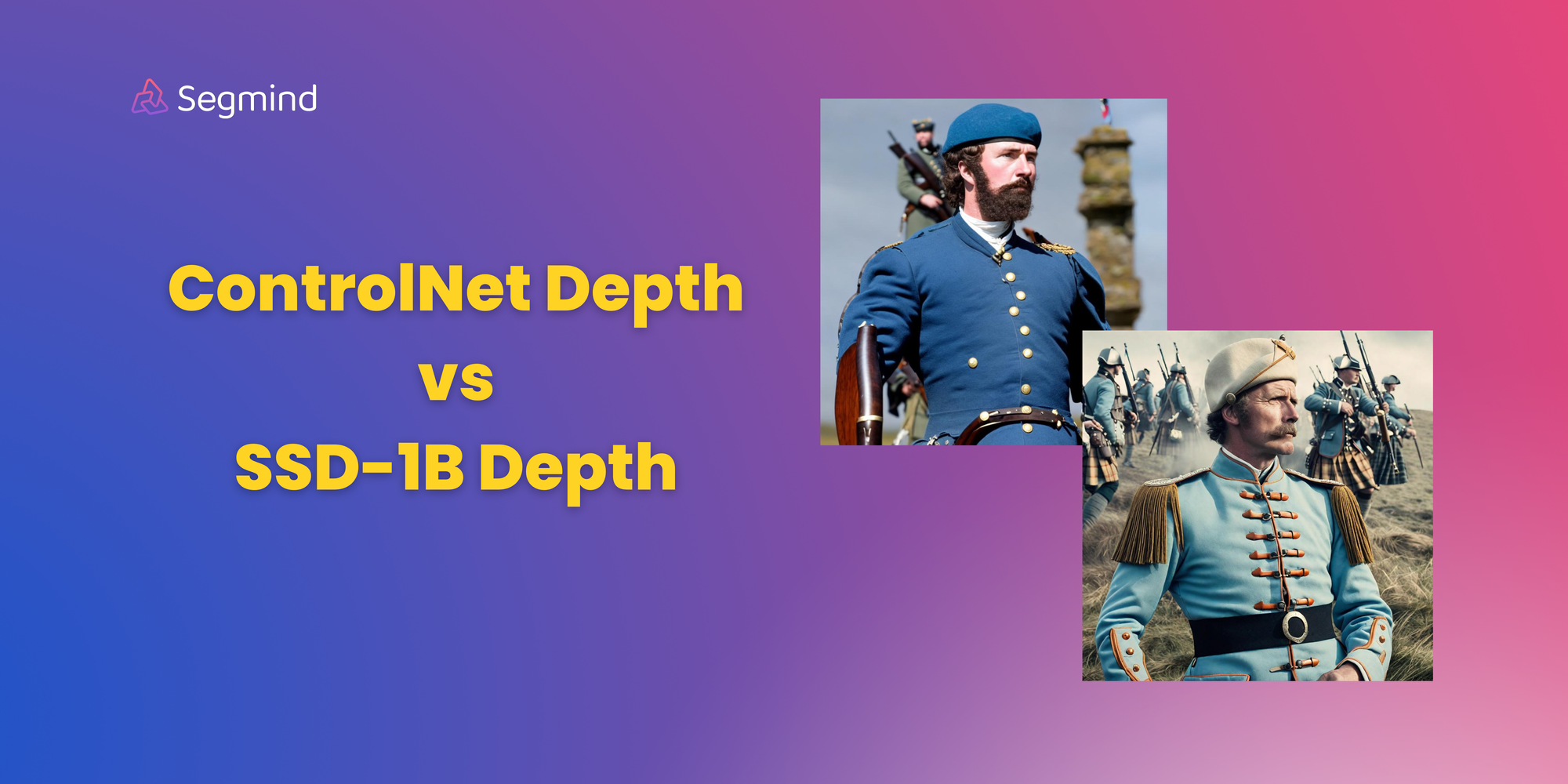
In this blog post, we will take a look at two models that transform the image with depth information and estimations SSD-Depth and ControlNet Depth.
These models create depth maps that transform two-dimensional visuals into immersive, three-dimensional experiences.
Understanding Depth Maps:
A depth map, depicted as a grayscale image matching the original's size, is vital in this transformation. Using shades from white to black, it cleverly conveys depth details. In simple terms, bright white means something is close, while darker shades imply greater distance.
How does ControlNet Depth work?
ControlNet Depth's technical prowess stems from its integration with the original ControlNet model. It operates by combining Stable Diffusion models with the ControlNet architecture.
The Stable Diffusion model acts as the foundation, efficiently producing high-quality images. The ControlNet layer manages conditional inputs or checkpoints and enhances the Stable Diffusion model by incorporating depth information.
This ControlNet layer converts checkpoints into a depth map, which, along with a text prompt, is inputted into the ControlNet Depth model. In simple terms, the checkpoints on the depth map correspond to ControlNet conditioned on Depth estimation. The model processes this data, leveraging provided depth details and specified features to generate a new image.
How does SSD-Depth work?
SSD Depth gets to improve the model by integrating SSD-1B with the ControlNet model. It combines the highly efficient architecture of SSD-1B which reduces the inference time alongside the controlnet architecture.
The SSD-1B Model acts as the foundation for producing high-quality images and the controlnet layer manages to enhance the pictures by incorporating the depth information.
This is done by introducing random fuzziness or noise to a clear picture. Gradually, this process turns the image into a blurry state. We This is accomplished by repeatedly removing the introduced fuzziness or noise, known as denoising. Finally, the depth maps introduce layers to a flat image, creating a more realistic sensation. Throughout each denoising step, the depth map, working in tandem with a text prompt, plays a crucial role in the enhancement process.
Parameters present in these models:
- Negative prompts: Negative prompts allow users to define the kind of image they would not like to see while the image is generated without providing any input. These prompts serve as a guide to the image generation process, instructing it to exclude certain elements based on the user-provided text. By utilizing negative prompts, users can effectively prevent the generation of specific objects, and styles, address image abnormalities, and enhance overall image quality.
- Inference Steps: This parameter concerns the number of denoising steps, indicating the iterations in a process initiated by random noise from the text input. In each iteration, the model refines the image by progressively removing noise. A higher number of steps results in the increased production of high-quality images
- Guidance scale: They introduce layers to a flat image, creating a more realistic sensation. Throughout each denoising step, the depth map, working in tandem with a text prompt, plays a crucial role in the enhancement process.
- ControlNet scale: This parameter shapes how closely the image generation process follows both the input image and the given text prompt. A higher value strengthens the alignment between the generated image and the provided image-text input. Nevertheless, setting the value to the maximum isn't always recommended. While higher values may impact the artistic quality of the images, they don't necessarily capture the intricate details required for a comprehensive output.
- Scheduler: Within the Stable Diffusion pipeline, schedulers play a pivotal role in collaboration with the UNet component. Their primary function is crucial to the denoising process, executed iteratively across multiple steps. These steps are instrumental in the transformation of a randomly noisy image into a clean, high-quality image. Schedulers systematically eliminate noise from the image, generating new data samples in the process. Noteworthy schedulers in this context including Euler and UniPC are highly recommended in general and might vary for particular contexts.
Image comparisons of both these models
We will now take a look into how both these models stack up based on a few key factors, we would like to compare them based on
- Prompt adherence: How well the models stick to the given instructions.
- Art styles and Functionality: The variety and quality of artistic styles the models can produce.
By looking at these aspects, we can get a clearer picture of what each model brings to the table. As a side note, all parameter values in the respective models are fixed according to their best settings.
Prompt adherence:
Prompt adherence gauges how effectively a model follows given text instructions to craft an image. It's crucial to understand that there isn't a specific framework or precise metric for evaluating prompt adherence in text-to-image generation models. Thoughtfully crafted prompts act as a roadmap, guiding the model to produce images aligned with the intended vision.
Let's begin with simple prompts
Prompt : a cinematic cat standing at the stairs present in mount everest
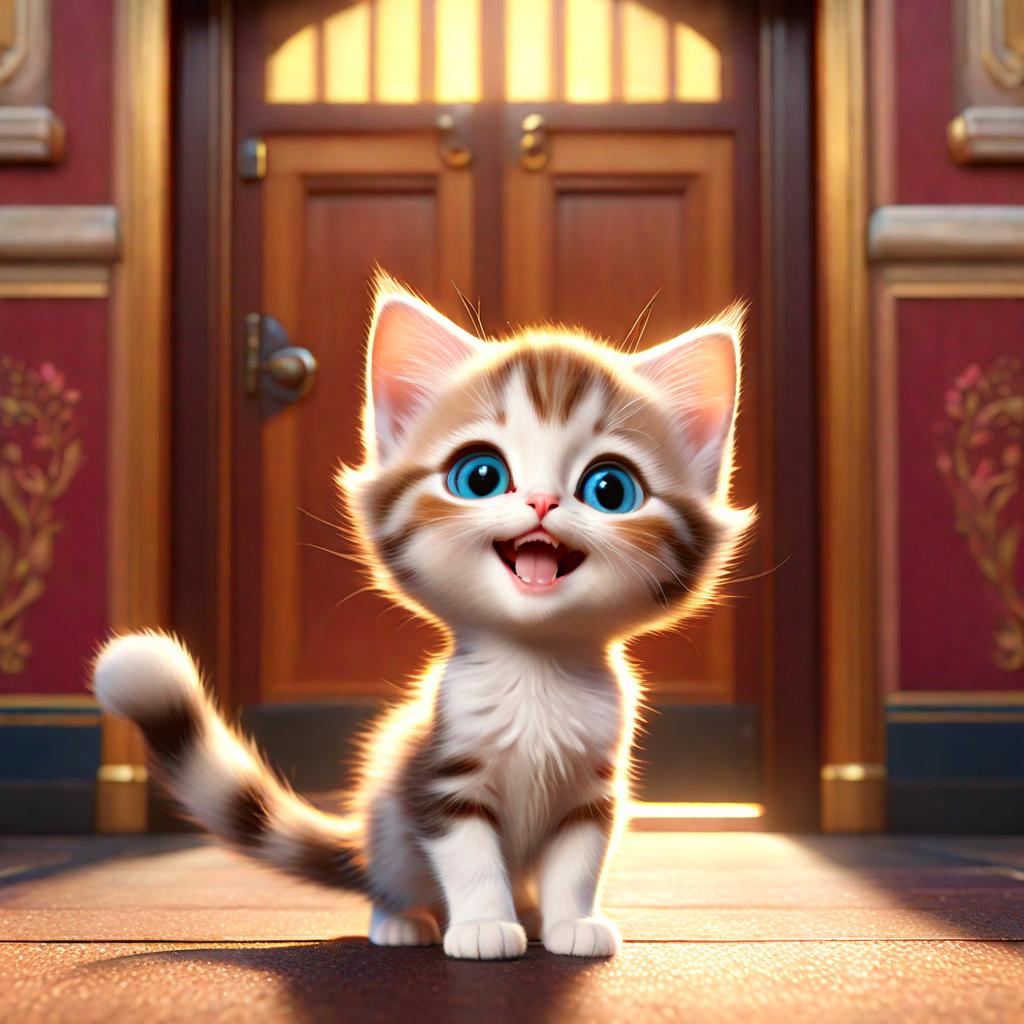
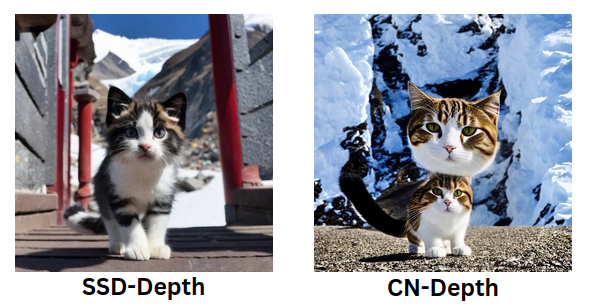
Prompt : Lonely man at a computer alone in a vast open futuristic office, with large windows, San Francisco sunset in background.
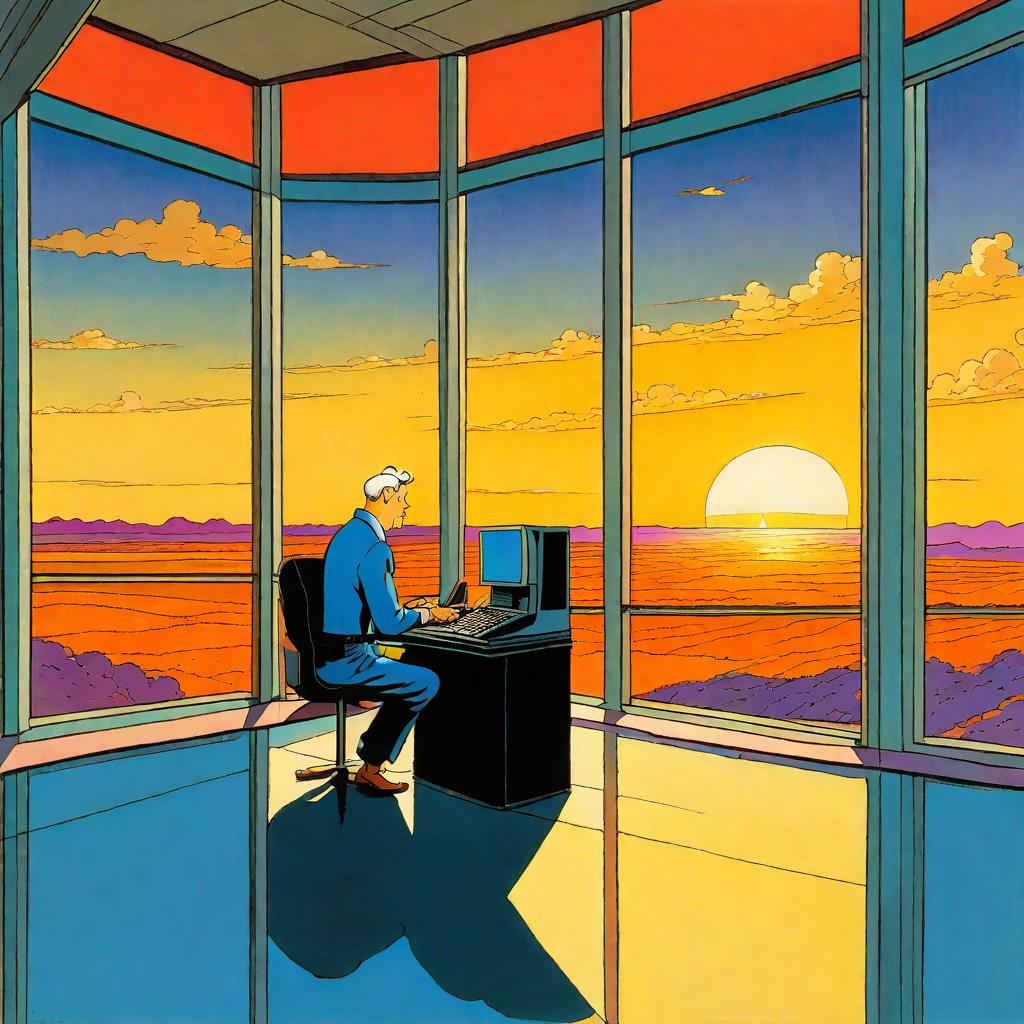
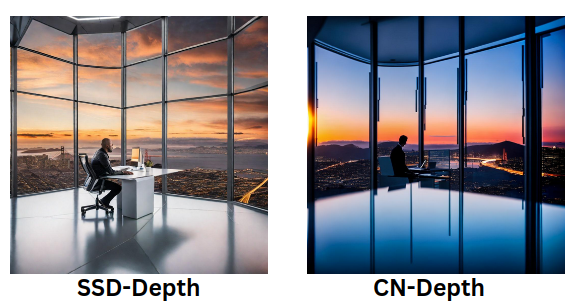
Up until now, we've provided straightforward prompts, but ControlNet Depth has struggled to comprehend them in the first image. In contrast, the SSD Depth model has consistently followed the prompts and produced images of high artistic quality.
Prompt: Two bears cuddling in a snowy forest , 4k , cinematic , dramtic lightning
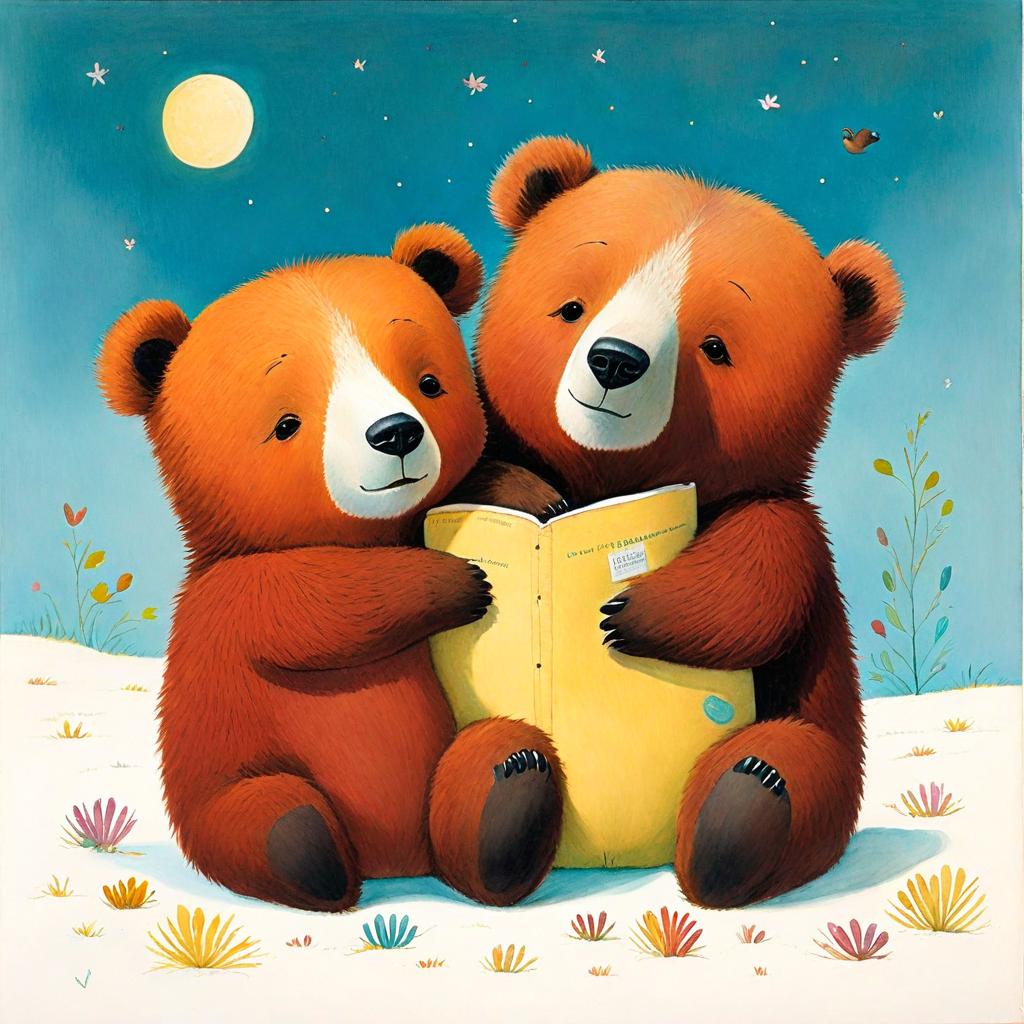
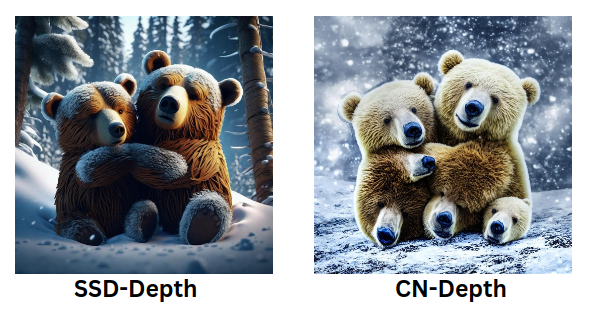
We observe variations in the images generated by both models. While SSD-Depth accurately captures the lighting on the bears, the texture of the skin appears somewhat artificial. In contrast, ControlNet Depth successfully renders realistic skin texture but struggles with both lighting and prompt adherence. Upon closer examination, the legs of the bears in the ControlNet Depth image resemble a bear's face, an unintended and unnecessary outcome.
Prompt : a beautiful japanese woman standing in the streets of tokyo , nightlife , street lightning , 4k , highly detailed , ghibili studios
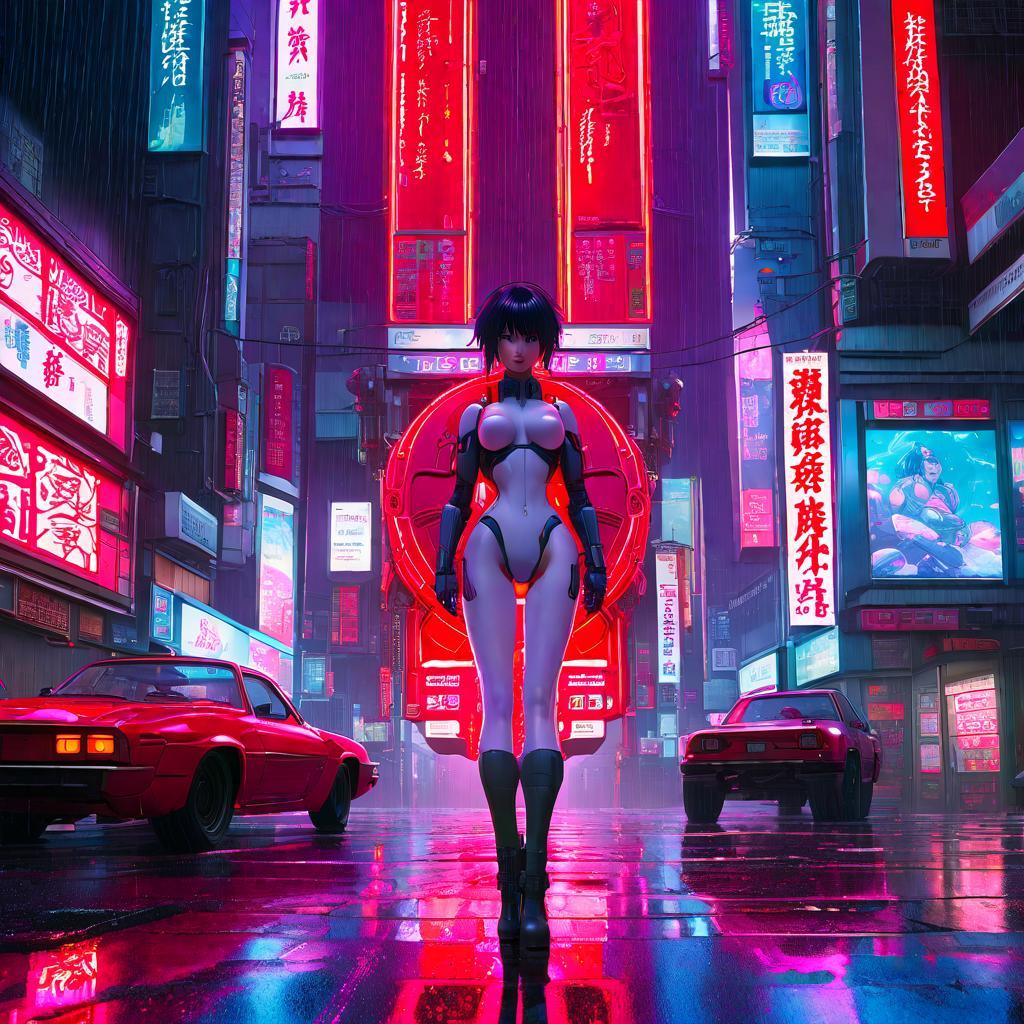
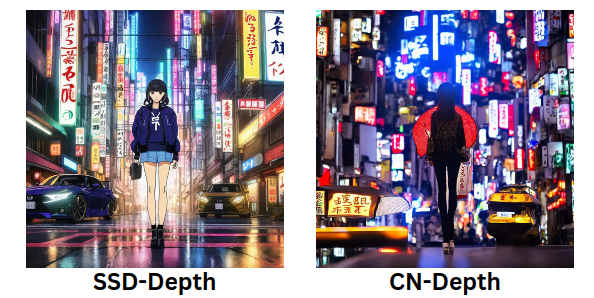
Prompt : a mughal warrior in front of castle, eroded interiors, intel core, dark cyan and orange, medivial art
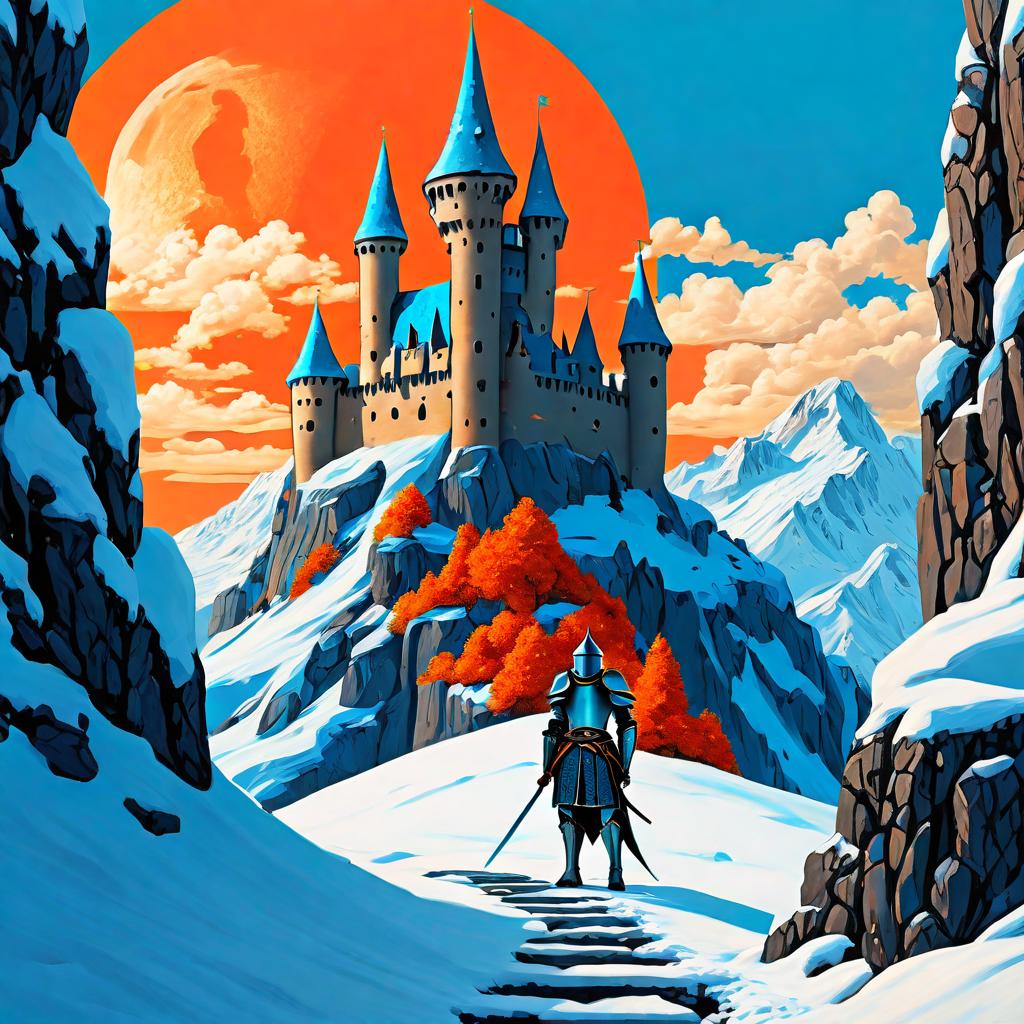
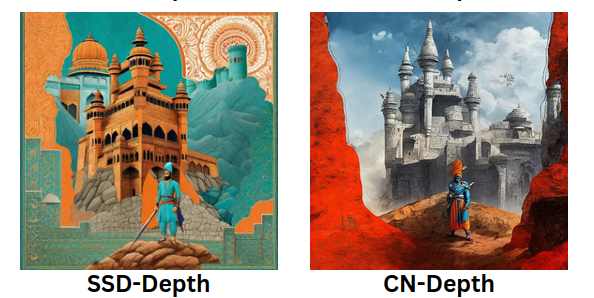
Prompt : Vouge Fashion editorial , analog fashion portrait. Portra 400 high dpi scan. Very Beautiful European model young , golden hour lighting
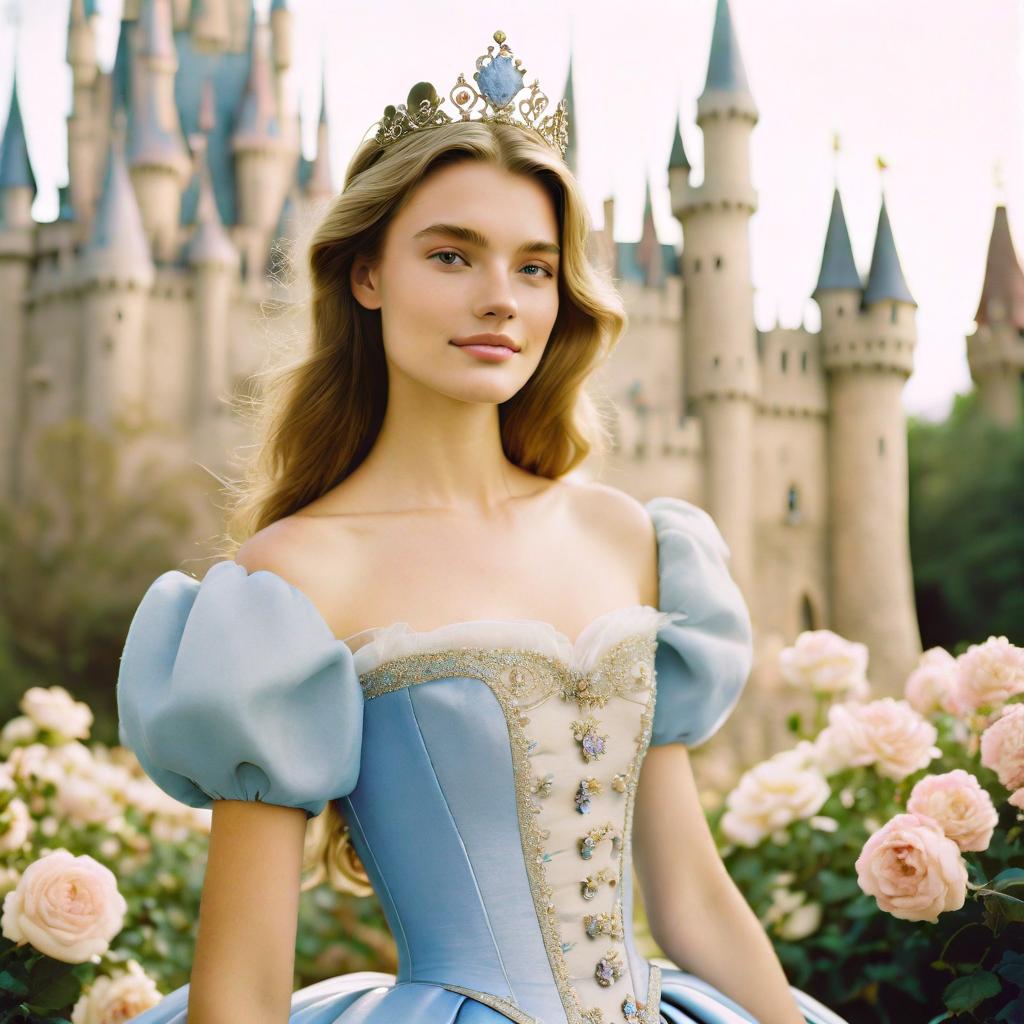
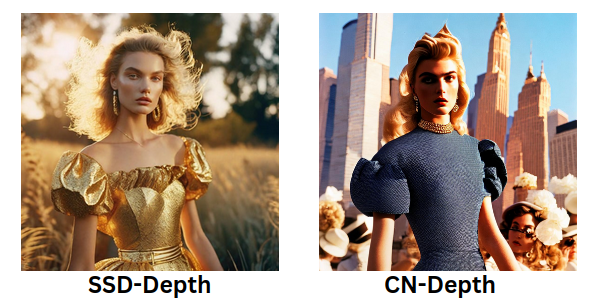
From the above examples, we can notice the image quality produced by SSD-Depth. It excels in adhering to prompts and delivering high artistic quality. Notably, finer details like skin and hair texture are significantly enhanced, maintaining harmony with the prompt-driven changes in the surroundings.
In contrast, ControlNet Depth falls short in various aspects. While it successfully generates a depth map, the final rendered image deviates from the intended qualities. Issues include disproportionate eyes, a disoriented and distorted background, and inconsistent color adherence.
Art styles and functionality:
Checking out the different art styles and the types of prompts these models can handle helps us grasp the full extent of their artistic skills. We'll continue exploring this aspect by giving the models various prompts to test their abilities, just like we did with the previous criteria.
Let us begin by transforming this image into an image where we change the subject to a French supermodel present in Antarctica and also shot in film.
Prompt : Candid Photojournalistic Shot French Supermodel, stunning arctic outfit, antarctica Colorful Gardens,Shot on Medium Format Filmschool
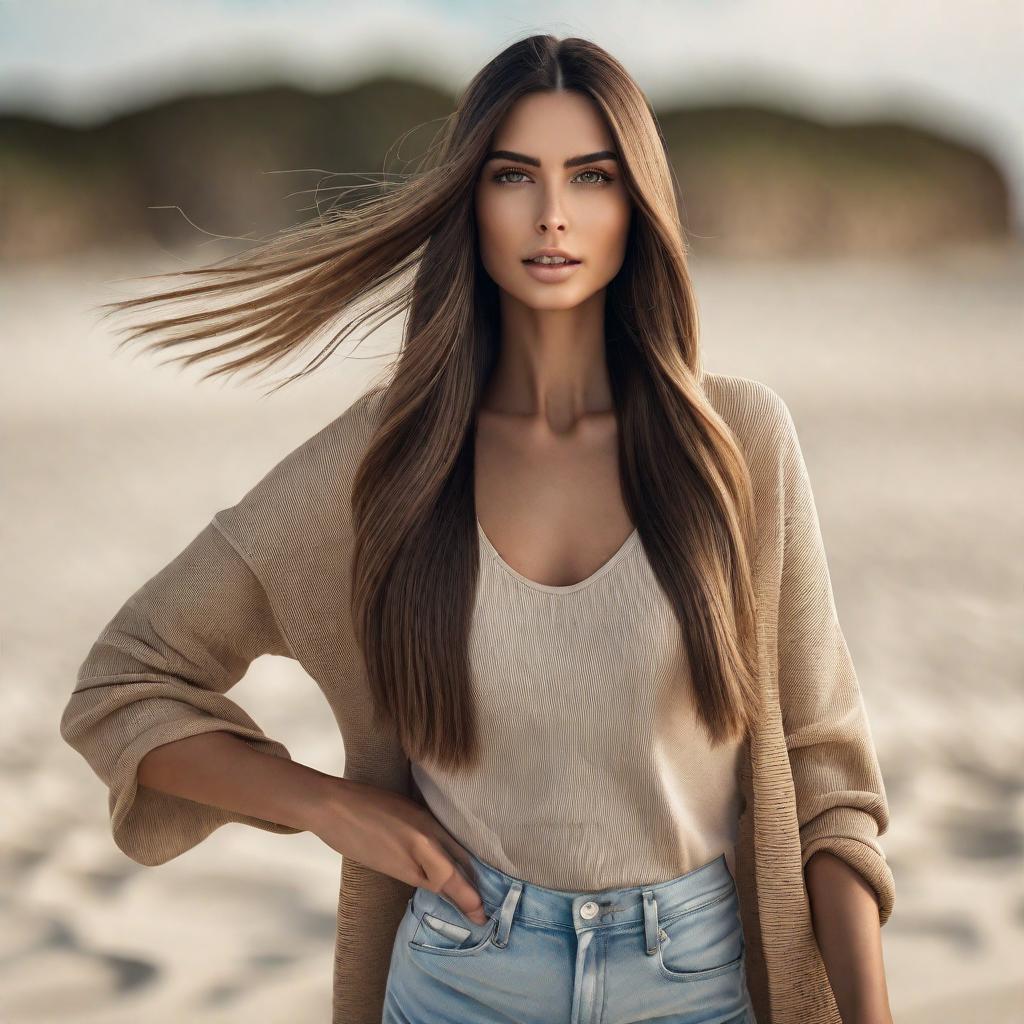
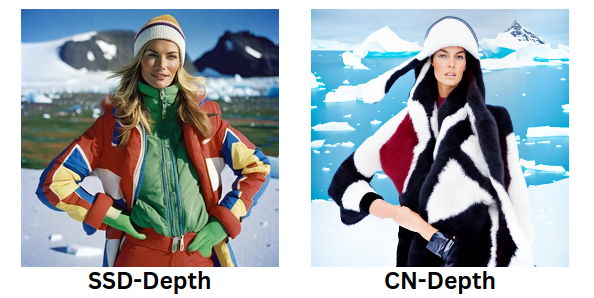
Really impressed by the image generated by SSD-Depth. The hair, costume, and overall integration with the surroundings are spot on. Additionally, the film type specified in the prompt is faithfully reflected in a highly textured and detailed image.
On the other hand, ControlNet Depth generates an image with disproportionate hands and struggles to meet the other conditions outlined in the prompt. The level of detail and adherence to prompt conditions seem lacking compared to the impressive results achieved by SSD-Depth.
Moving on let us now give a blunt prompt but filled with details transforming the below picture into a Scottish clansman in the field, with troops behind him shooting
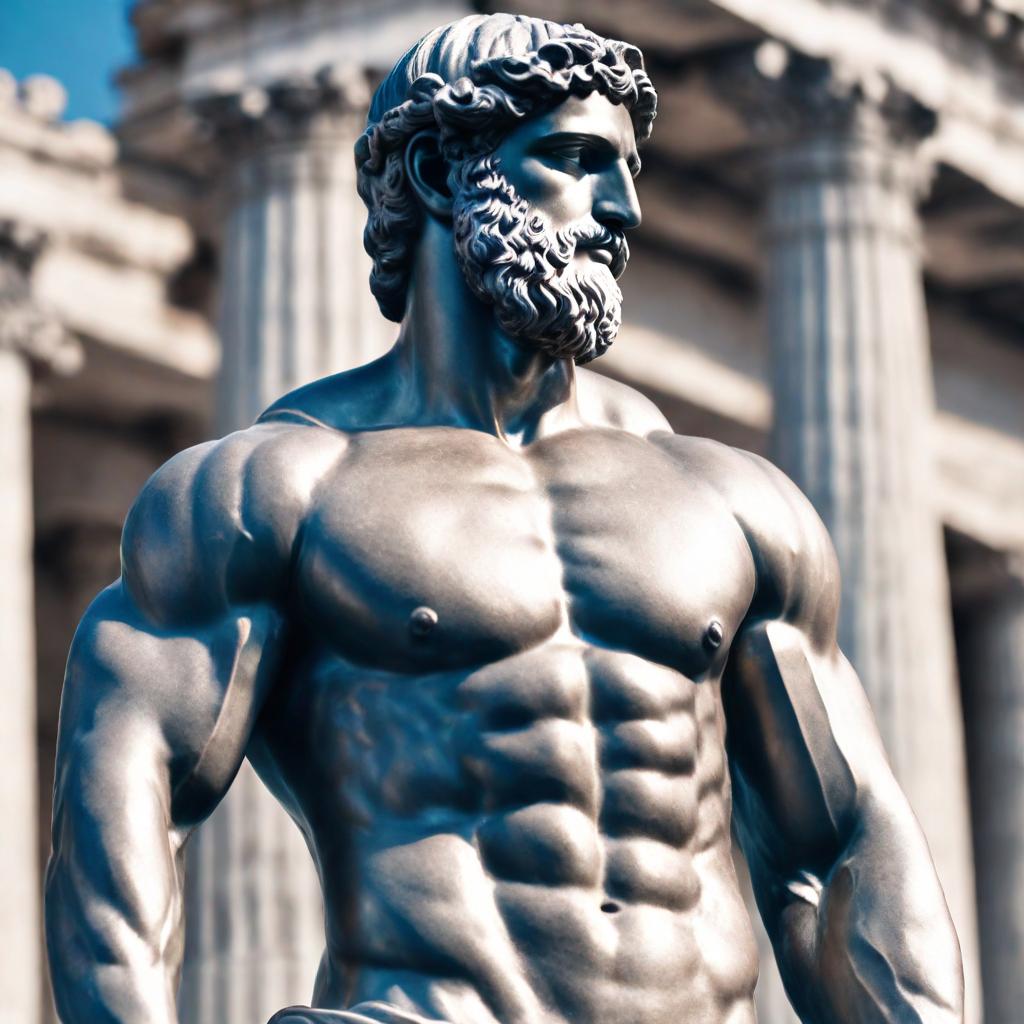
Prompt : Scottish clansman fighting in a battlefield using flintlock muskets, wearing sky blue 19th-century coats, flat caps, and kilts , wide shot shooting troops
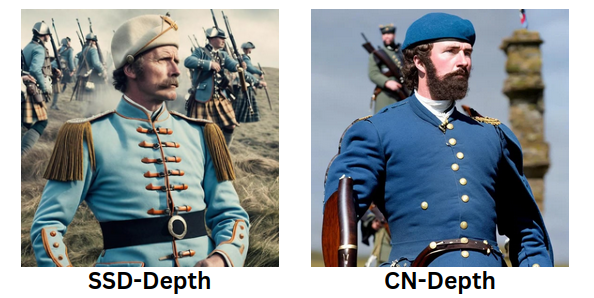
It is interesting to note how the response to the latter part of the prompt, "wide shot shooting troopings," the SSD-Depth model introduces figures in the background of the subject. In contrast, the ControlNet Depth model, limited to modifying the subject, produces lower-quality results primarily due to its weaker adherence to the prompt.
Now, let's proceed to transform the image into a stylish wallpaper, enhancing its visual appeal.
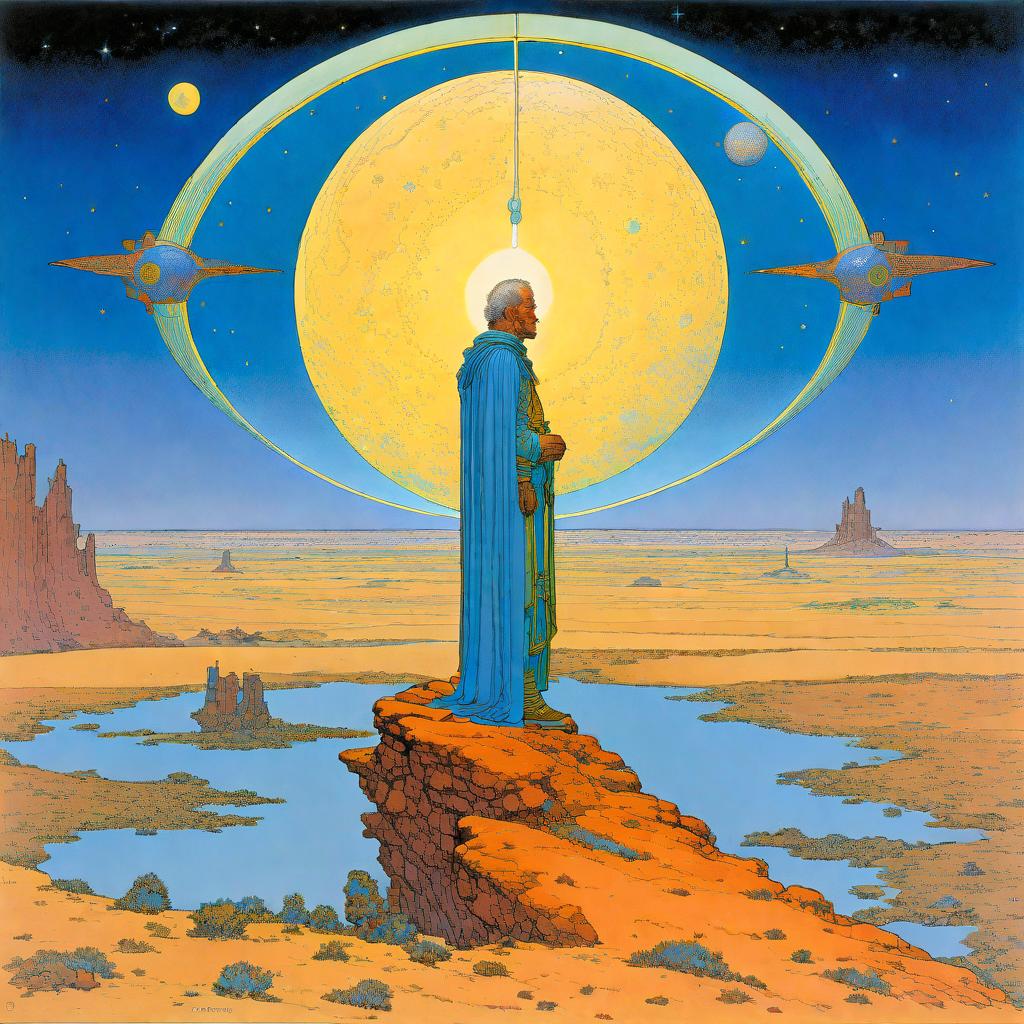
Prompt : Tamerlane male in medieval style, timurid clothes, green eyes, tan skin, dark, high cheekbones, handsome face, full body, cinematic composition, detailed face, background mongolian plains
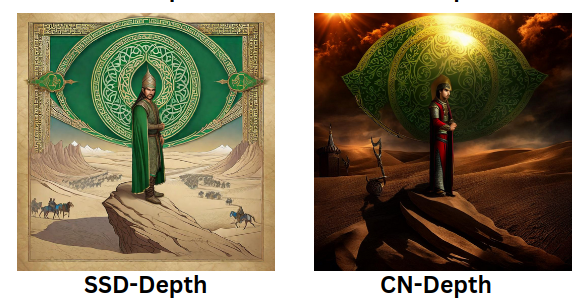
Conclusion:
Analyzing the images above, we can conclude that SSD-Depth excels in generating superior depth maps, adhering to prompts, and delivering high-quality artistic images. In contrast, ControlNet Depth often falls short of prompt understanding and exhibits poorer details in its output.