Recommended SSD-1B Canny Settings
A comprehensive guide to maximizing the potential of the SSD-1B Canny model in image transformation.
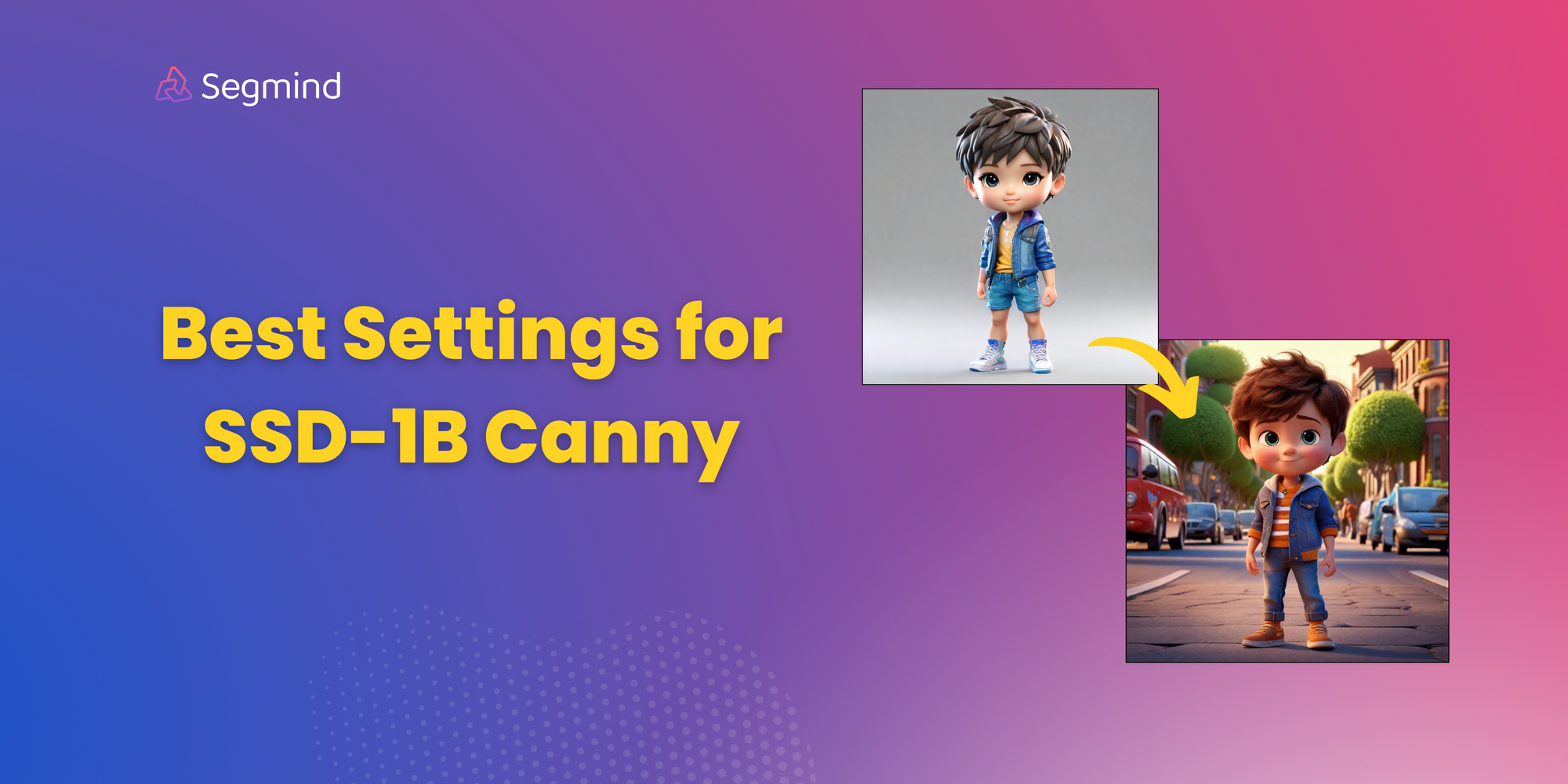
In this blog post, we're about to explore a groundbreaking approach that hands you the reins of image transformation like never before. Picture this – not just highlighting, but precisely defining and accentuating edges in every image at your fingertips.
At the heart of the SSD-1B Canny model is the robust Canny edge detection foundation, celebrated for its exceptional precision in revealing contours within images. Now, imagine taking that precision and soaring to new heights. We will delve deep into the best settings of the SSD-1B Canny model, however before diving deep into effective parameter choices we will take a look into the architecture
Architecture
The training algorithm of SSD-1B employs Canny edges as a foundational element to train the model to produce images with identical edges. This unique approach involves leveraging Canny edges as a set of weights, which play a crucial role in shaping the generation process. These weights serve as controllers, influencing the intensity, position, and shape of the edges within the generated image.
SSD-1B Canny employs Canny edges as a foundational element to train the model to produce images with identical edges. In essence, the algorithm learns from the Canny edges data, allowing the network to discern vital image features and seamlessly integrate them into the output of Stable Diffusion models. This intricate process not only refines the model's ability to replicate edges accurately but also equips it with a nuanced understanding of essential image characteristics.
As a result, the SSD-1B model becomes adept at producing images that not only mirror the provided edges but also exhibit a heightened level of fidelity and relevance to the underlying features of the input data. We will now take a look into the best settings to be utilized in order to get the best out of this model.
Best settings of SSD-1B Canny:
Steps:
This parameter corresponds to the number of denoising steps in the image generation process. It begins with the introduction of random noise generated from the text input, initiating a repeated cycle. At each step in the cycle, some noise is removed, leading to a gradual enhancement in image quality.
Increasing the value of steps translates to more cycles of noise removal, ultimately yielding higher-quality images. In essence, a higher step count allows the model to undergo a more thorough refinement process, contributing to the production of images with superior clarity and visual appeal. We will take a look at a few examples
Prompt : beautiful , American woman , with dark black hair ,wearing polka dot dress, holding a bag, cinematic
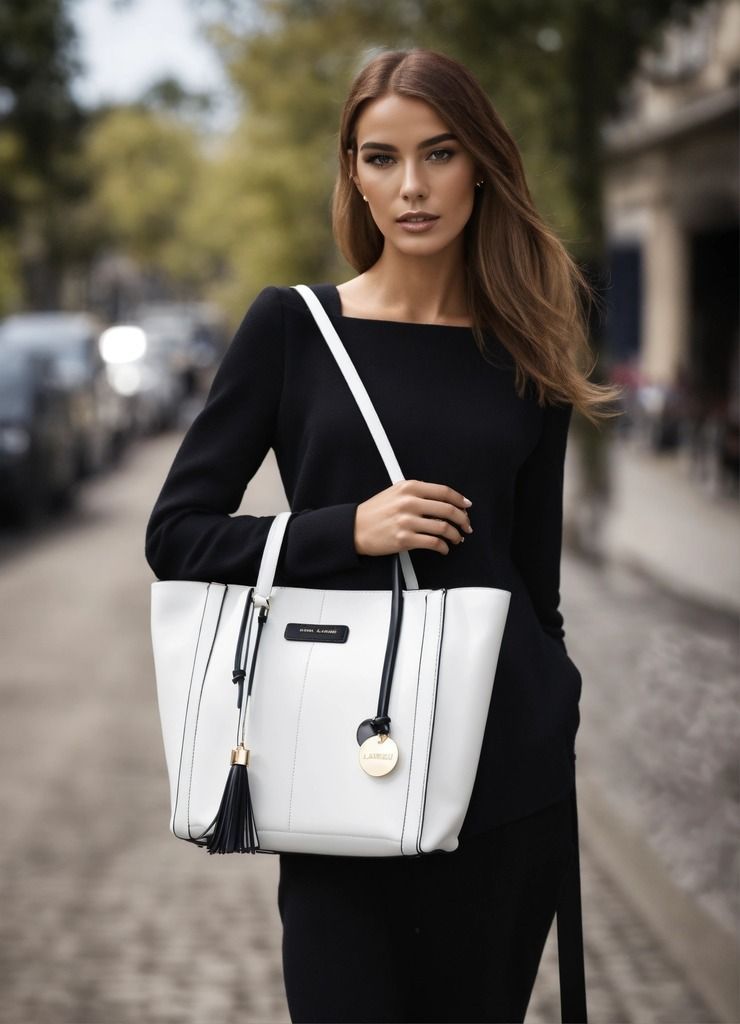
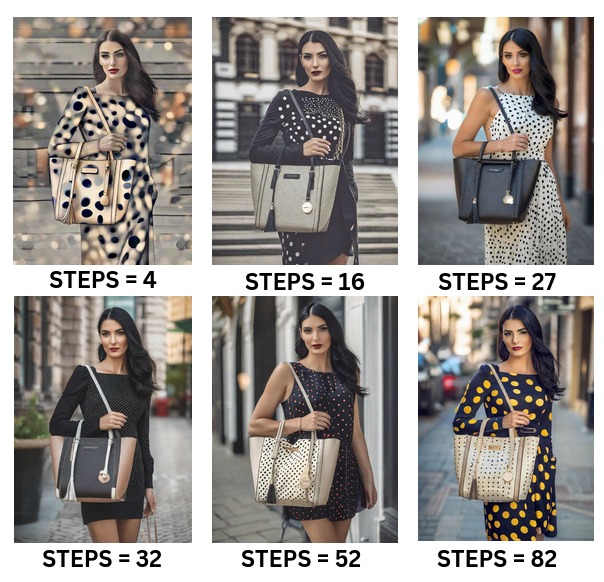
For steps below the range of 40, the images generated tend to exhibit poor artistic quality. High-quality image generation is particularly noticeable within the range of steps 45 to 60. Beyond this range, subsequent steps show little improvement in the overall quality of the generated images. However, the form of the image undergoes repeated alterations without introducing additional details.
It's worth noting that minor details, such as the texture of the cloth and the bag see incremental improvements with each step in the phase.
Guidance Scale
The guidance scale parameter holds a crucial role in shaping how closely the image generation process follows the given text prompts. It serves as a fundamental element in guaranteeing that the generated images closely match the intended meaning and context conveyed by the input text prompts.
When set to a higher value, it strengthens the connection between the generated image and the input text. However, this heightened connection comes with a trade-off – it diminishes diversity and, subsequently, the overall quality of the generated images. It's a delicate balance where adjusting the guidance scale involves considering the desired level of adherence to the text versus the need for diverse and high-quality image outcomes.
Prompt : Girls with icy blue eyes charcoal colored slightly wavy hair, bangs, beautiful, fantasy vibe, mysterious , highly detailed, anime , Ghibli Studios style
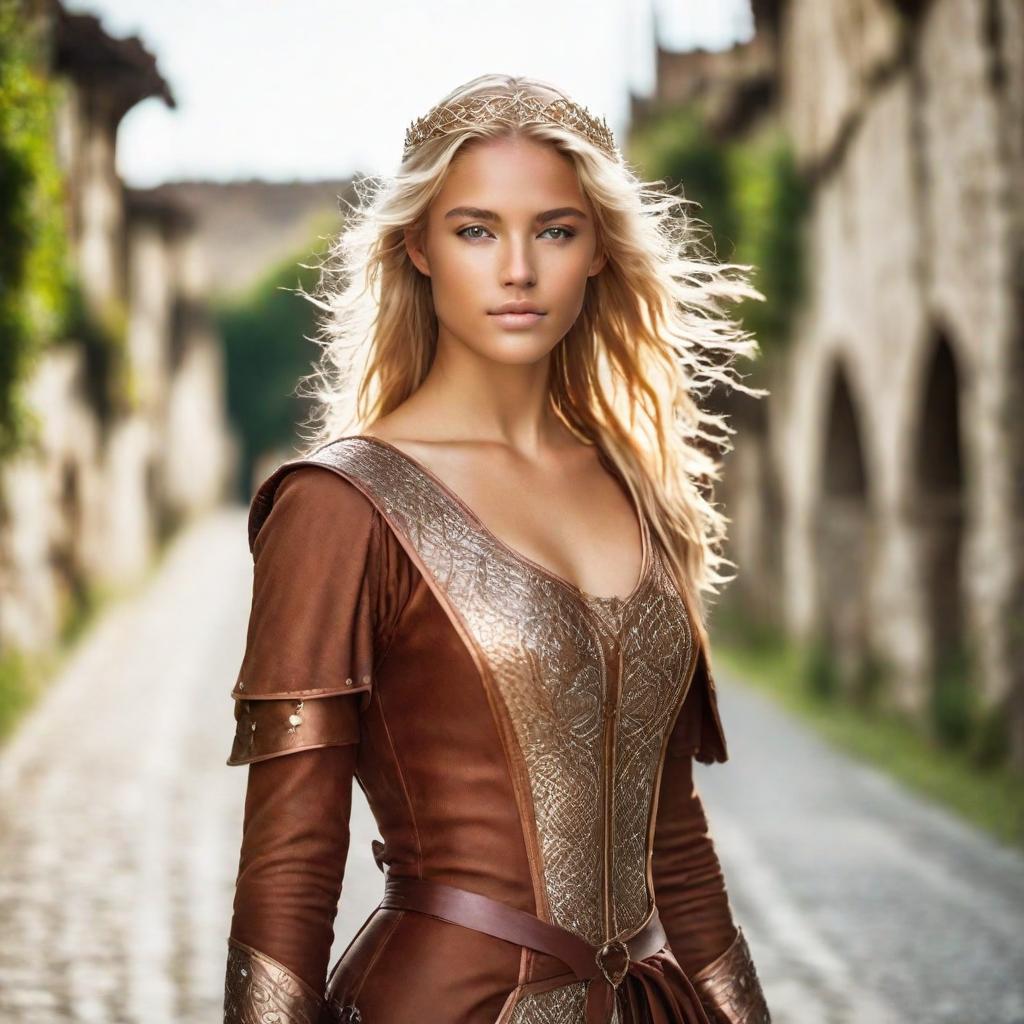
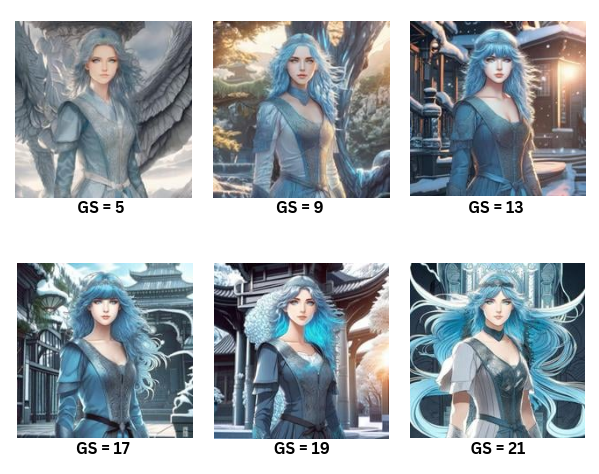
In the optimal range of 12 to 17, the emphasis shifts to highlighting details in the image. For those seeking to generate images rich in tiny details as specified in the prompt, it is advisable to choose values within this specific range. On the other hand, values above 19 or 20 enforce strict adherence to the text but may also impact the artistic quality of the generated image.
After values of 17, we can see the edges being more defined but also the quality of the image doesn't improve significantly.
Controlnet Scale
This parameter affects how closely the generated image follows the input image and text prompt. A higher value strengthens the connection between the generated image and the given input.
It's important to note that setting the value to the maximum isn't always recommended. While higher values might enhance the artistic quality of the images, they may not capture the intricate details or variance needed for a comprehensive output.
Prompt: a brunette with long straight hair surrounding face, beach outfit, neutral expression, slim body, ultrarealistic, photography, 8k
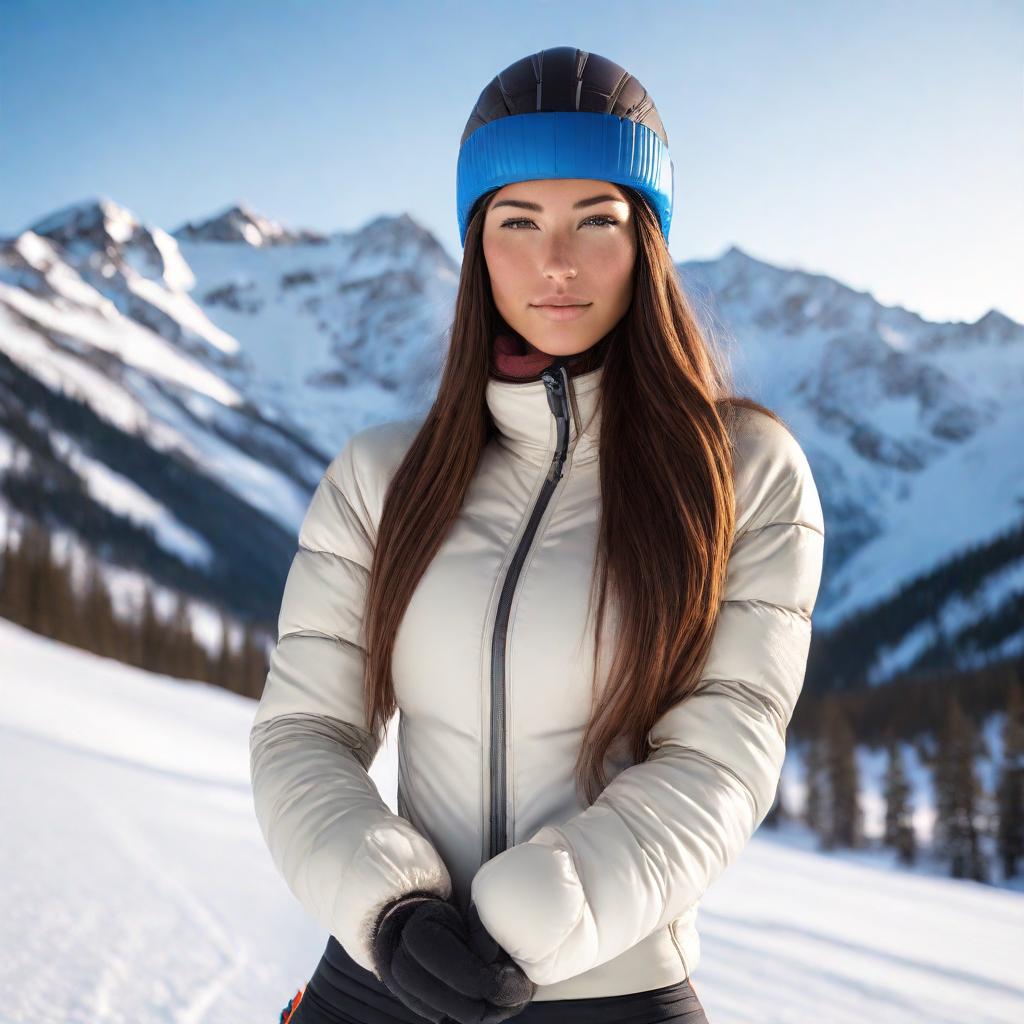
Output:
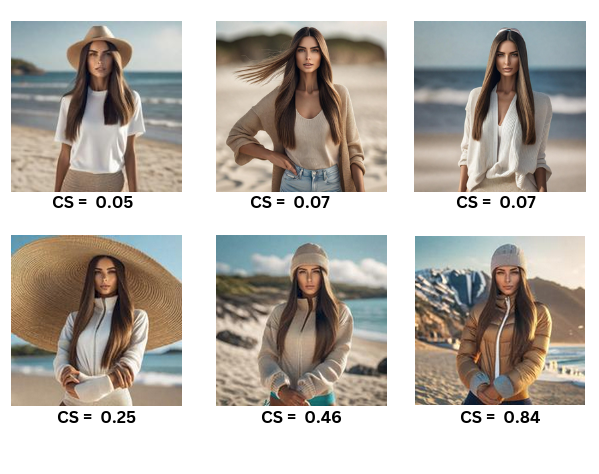
Prompt : a cute little boy standing in the street , Pixar style
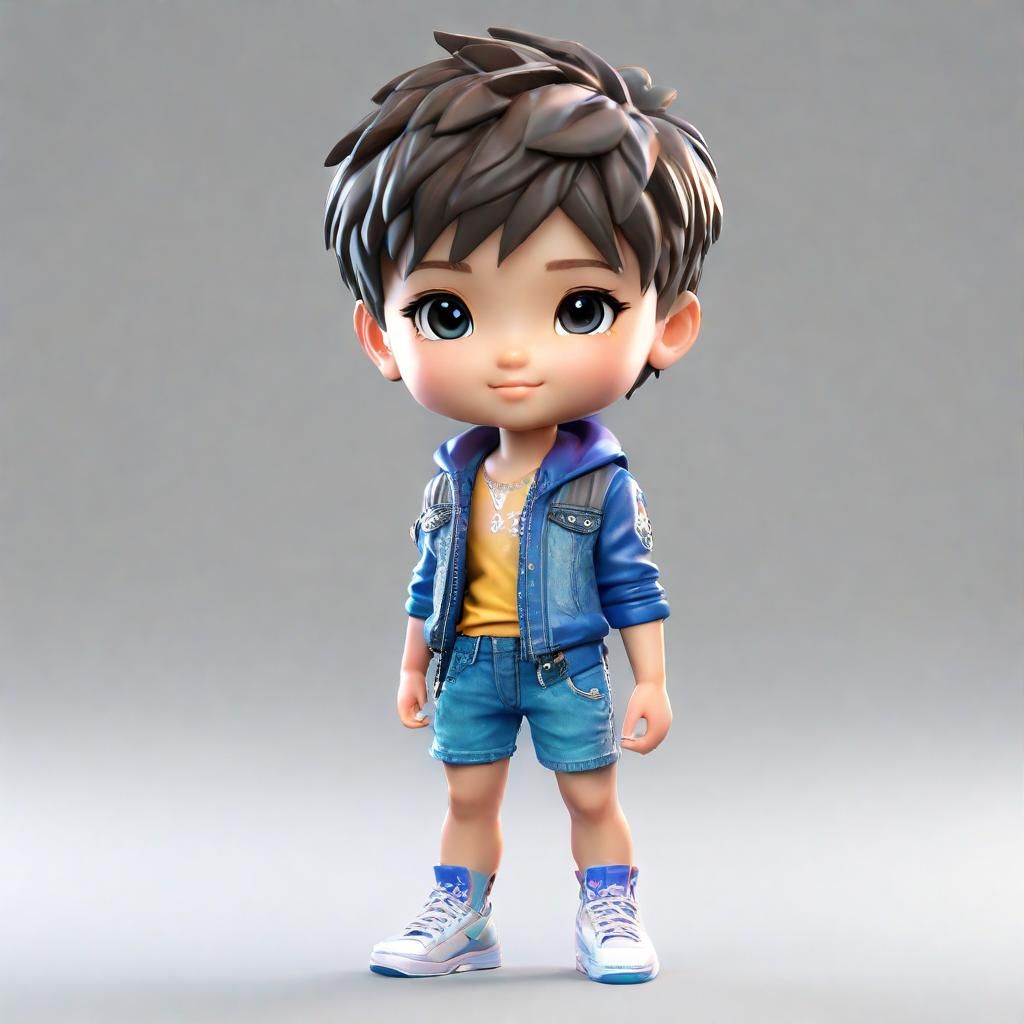
Output:
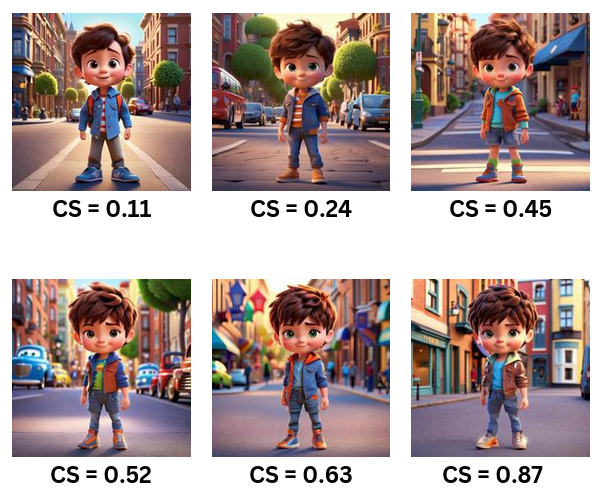
Lower values of the controlnet scale, ranging from 0.0 to 0.20, lead to a subtle alignment of the generated image with the text prompt. This range introduces variety and diversity in the images produced.
On the contrary, as the controlnet scale value increases beyond 0.35, we observe that the generated images closely replicate the details of the original input image. At higher values, there is a reduction in artistic variation, and the images tend to adhere closely to the specifics of the initial input, diminishing the creation of highly diverse and artistic outputs.
Negative prompts
Negative prompts offer users a way to guide image generation by specifying elements they wish to exclude without providing explicit input. These prompts act as a filter, instructing the generation process to avoid certain features based on user-provided text.
By leveraging negative prompts, users can prevent the generation of specific objects, and styles, and address image abnormalities, contributing to an overall enhancement of image quality.
Here are examples of commonly used negative prompts:
- Basic Negative Prompts: worst quality, normal quality, low quality, low res, blurry, text, watermark, logo, banner, extra digits, cropped, jpeg artifacts, signature, username, error, sketch, duplicate, ugly, monochrome, horror, geometry, mutation, disgusting.
- For Adult Content: NSFW, nude, censored.
- For Realistic Characters: extra fingers, mutated hands, poorly drawn hands, poorly drawn faces, mutation, deformed, blurry, dehydrated, bad anatomy, bad proportions, extra limbs, cloned face, disfigured, gross proportions, malformed limbs, missing arms, missing legs, extra arms, extra legs, fused fingers, too many fingers, a long neck.
Effectively utilizing these negative prompts provides users with a powerful tool to shape and refine the output of the image-generation process based on their preferences and requirements.
Prompt : color pigeon in a green lush forest
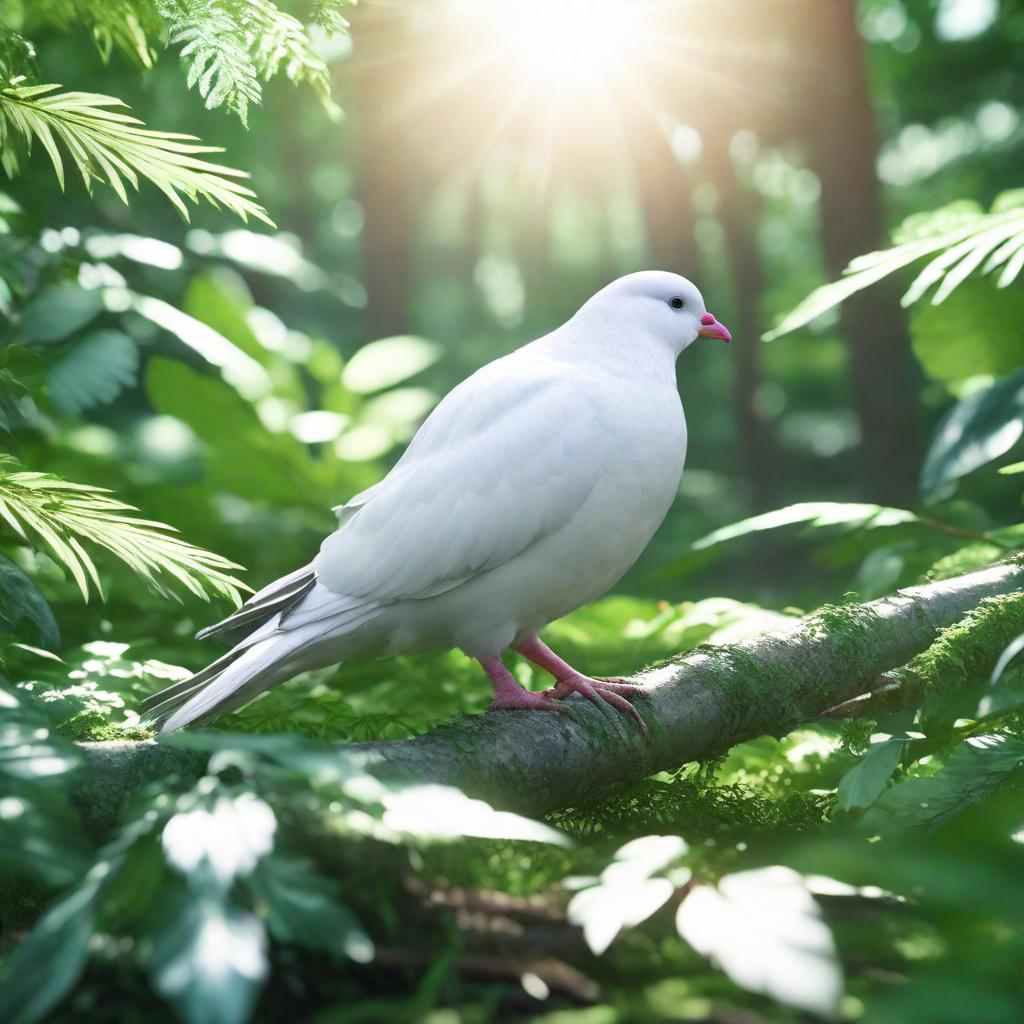
Output:
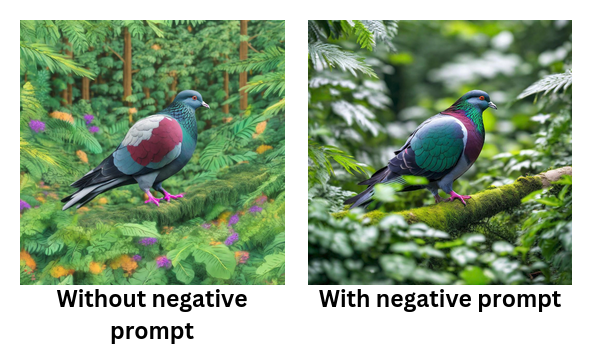
Scheduler
In the context of the Stable Diffusion pipeline, schedulers are algorithms that are used alongside the UNet component. The primary function of these schedulers is integral to the denoising process, which is executed iteratively in multiple steps. These steps are essential for transforming a completely random noisy image into a clean, high-quality image.
The role of schedulers is to systematically remove noise from the image, generating new data samples in the process. Among the various schedulers, UniPC, and DDPM are highly recommended.
Conclusion
In conclusion, SSD-1B Canny emerges as a powerful tool for effortlessly crafting eye-catching and stylish images. Its unique strength lies in striking an optimal balance between efficiency and visual quality in edge detection.
Harnessing the precision of Canny edge detection, SSD-1B Canny ensures accurate delineation of edges. This precision, coupled with its customizable controls, empowers users with the flexibility to tailor edge detection to their unique preferences.
Moreover, SSD-1B Canny proves to be a versatile solution applicable across various domains, from artistic pursuits to technical image analysis. Its ability to deliver instant results in real-time manipulation adds to its appeal, providing users with swift feedback and outcomes.